Roadmap Learning for Probabilistic Occupancy Maps With Topology-Informed Growing Neural Gas
IEEE Robotics and Automation Letters(2021)
摘要
We address the problem of generating navigation roadmaps for uncertain and cluttered environments represented with probabilistic occupancy maps. A key challenge is to generate roadmaps that provide connectivity through tight passages and paths around uncertain obstacles. We propose the topology-informed growing neural gas algorithm that leverages estimates of probabilistic topological structures computed using persistent homology theory. These topological structure estimates inform the random sampling distribution to focus the roadmap learning on challenging regions of the environment that have not yet been learned correctly. We present experiments for three real-world indoor point-cloud datasets represented as Hilbert maps. Our method outperforms baseline methods in terms of graph connectivity, path solution quality, and search efficiency. Compared to a much denser PRM*, our method achieves similar performance while enabling a 27× faster query time for shortest-path searches.
更多查看译文
关键词
Motion and path planning,computational geometry,probability and statistical methods
AI 理解论文
溯源树
样例
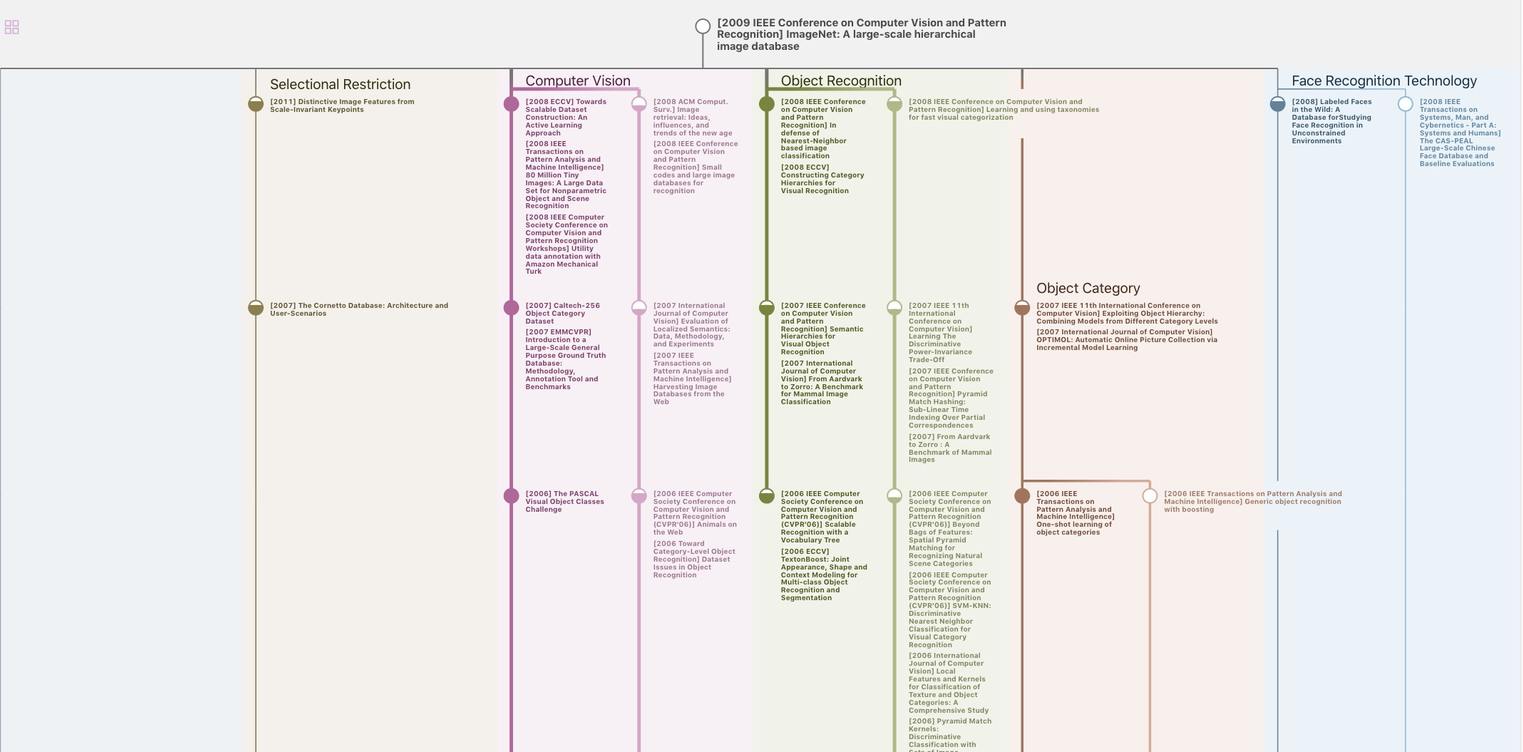
生成溯源树,研究论文发展脉络
Chat Paper
正在生成论文摘要