Identifying The Primary Odor Perception Descriptors By Multi-Output Linear Regression Models
APPLIED SCIENCES-BASEL(2021)
摘要
Semantic odor perception descriptors, such as "sweet", are widely used for product quality assessment in food, beverage, and fragrance industries to profile the odor perceptions. The current literature focuses on developing as many as possible odor perception descriptors. A large number of odor descriptors poses challenges for odor sensory assessment. In this paper, we propose the task of narrowing down the number of odor perception descriptors. To this end, we contrive a novel selection mechanism based on machine learning to identify the primary odor perceptual descriptors (POPDs). The perceptual ratings of non-primary odor perception descriptors (NPOPDs) could be predicted precisely from those of the POPDs. Therefore, the NPOPDs are redundant and could be disregarded from the odor vocabulary. The experimental results indicate that dozens of odor perceptual descriptors are redundant. It is also observed that the sparsity of the data has a negative correlation coefficient with the model performance, while the Pearson correlation between odor perceptions plays an active role. Reducing the odor vocabulary size could simplify the odor sensory assessment and is auxiliary to understand human odor perceptual space.
更多查看译文
关键词
odor perception, primary odor perception descriptor, clustering, linear regression
AI 理解论文
溯源树
样例
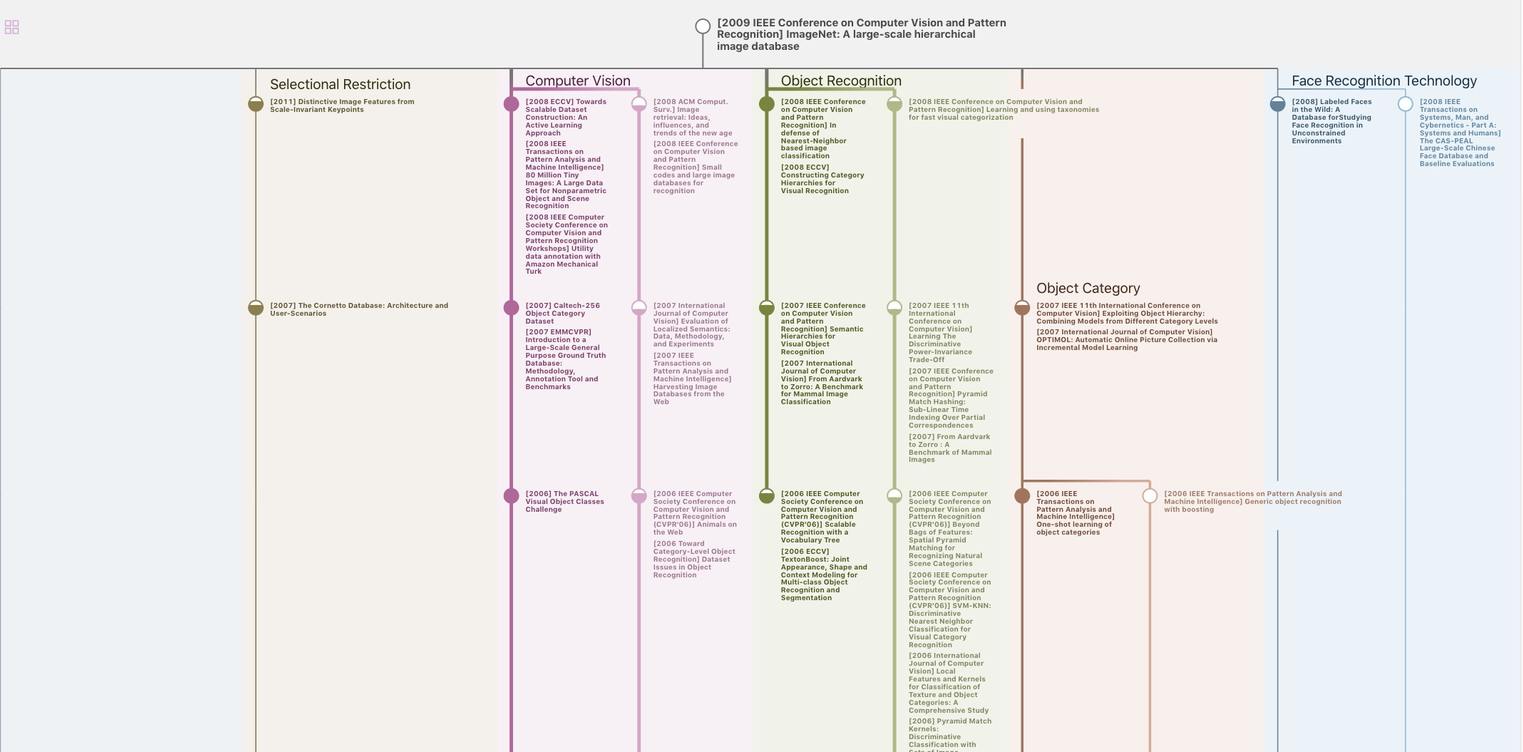
生成溯源树,研究论文发展脉络
Chat Paper
正在生成论文摘要