Efficient Neural Networks For Edge Devices
COMPUTERS & ELECTRICAL ENGINEERING(2021)
摘要
Due to limited computation and storage resources of industrial internet of things (IoT) edge devices, many emerging intelligent industrial IoT applications based on deep neural networks (DNNs) heavily depend on cloud computing for computation and storage. However, cloud computing faces technical issues in long latency, poor reliability, and weak privacy, resulting in the need for on-device computation and storage. On-device computation is essential for many time-critical industrial IoT applications, which require real-time data processing. In this paper, we review three major research areas for on-device computation, specifically quantization, pruning, and network architecture design. The three techniques could enable a DNN model to be deployed on edge devices for real-time computation and storage, mainly due to the reduction of computation and space complexity. More importantly, these techniques could make DNNs applicable to industrial IoT devices.
更多查看译文
关键词
Edge computing, Cloud computing, Neural network, Edge devices, Quantization, Pruning
AI 理解论文
溯源树
样例
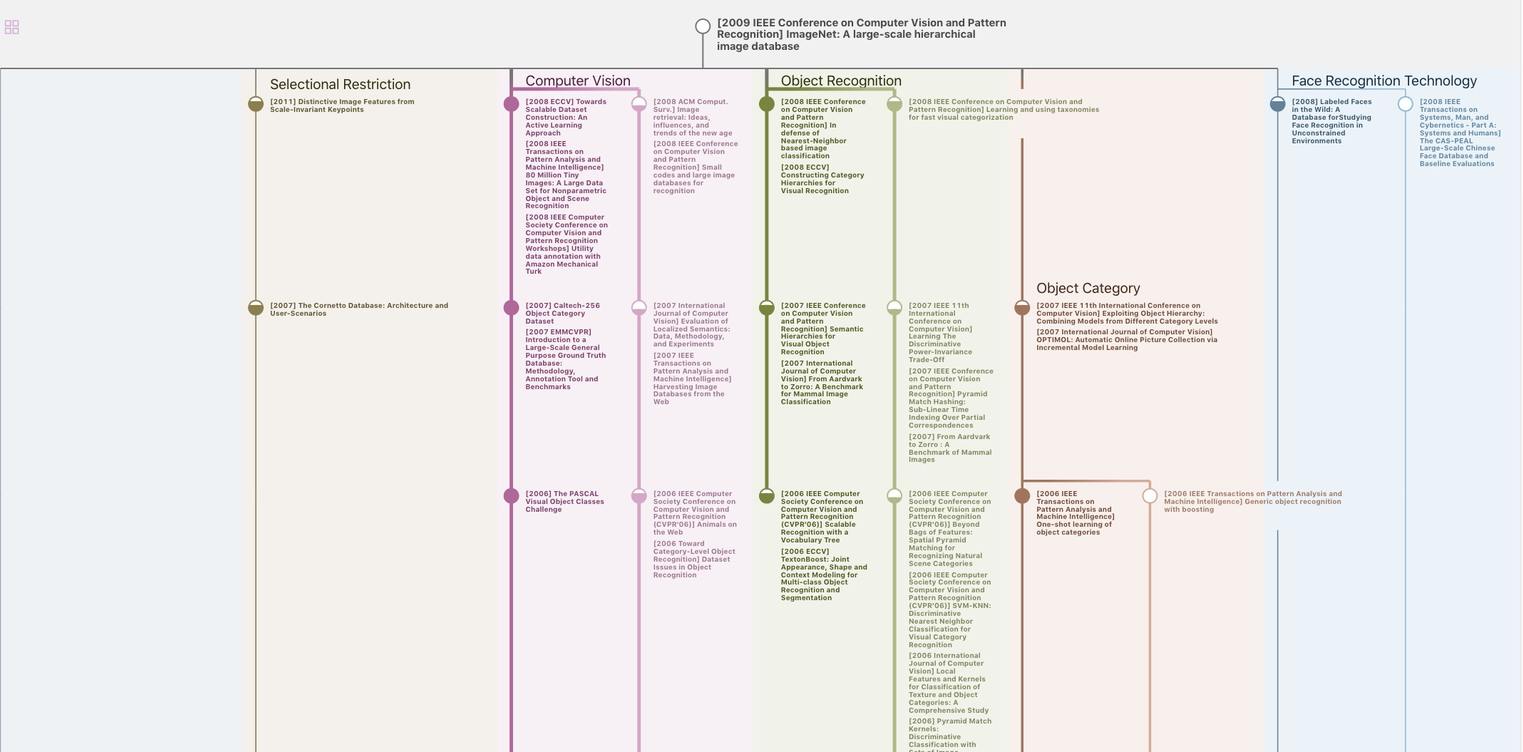
生成溯源树,研究论文发展脉络
Chat Paper
正在生成论文摘要