Estimating Glioblastoma Biophysical Growth Parameters Using Deep Learning Regression.
Brainlesion : glioma, multiple sclerosis, stroke and traumatic brain injuries. BrainLes (Workshop)(2021)
摘要
Glioblastoma ( ) is arguably the most aggressive, infiltrative, and heterogeneous type of adult brain tumor. Biophysical modeling of GBM growth has contributed to more informed clinical decision-making. However, deploying a biophysical model to a clinical environment is challenging since underlying computations are quite expensive and can take several hours using existing technologies. Here we present a scheme to accelerate the computation. In particular, we present a deep learning ( )-based logistic regression model to estimate the GBM's biophysical growth in seconds. This growth is defined by three tumor-specific parameters: 1) a diffusion coefficient in white matter ( ), which prescribes the rate of infiltration of tumor cells in white matter, 2) a mass-effect parameter ( ), which defines the average tumor expansion, and 3) the estimated time ( ) in number of days that the tumor has been growing. Preoperative structural multi-parametric MRI ( ) scans from = 135 subjects of the TCGA-GBM imaging collection are used to quantitatively evaluate our approach. We consider the mpMRI intensities within the region defined by the abnormal FLAIR signal envelope for training one DL model for each of the tumor-specific growth parameters. We train and validate the DL-based predictions against parameters derived from biophysical inversion models. The average Pearson correlation coefficients between our DL-based estimations and the biophysical parameters are 0.85 for , 0.90 for , and 0.94 for , respectively. This study unlocks the power of tumor-specific parameters from biophysical tumor growth estimation. It paves the way towards their clinical translation and opens the door for leveraging advanced radiomic descriptors in future studies by means of a significantly faster parameter reconstruction compared to biophysical growth modeling approaches.
更多查看译文
关键词
Biophysical growth model,Brain tumor,Deep learning,Glioblastoma,Regression
AI 理解论文
溯源树
样例
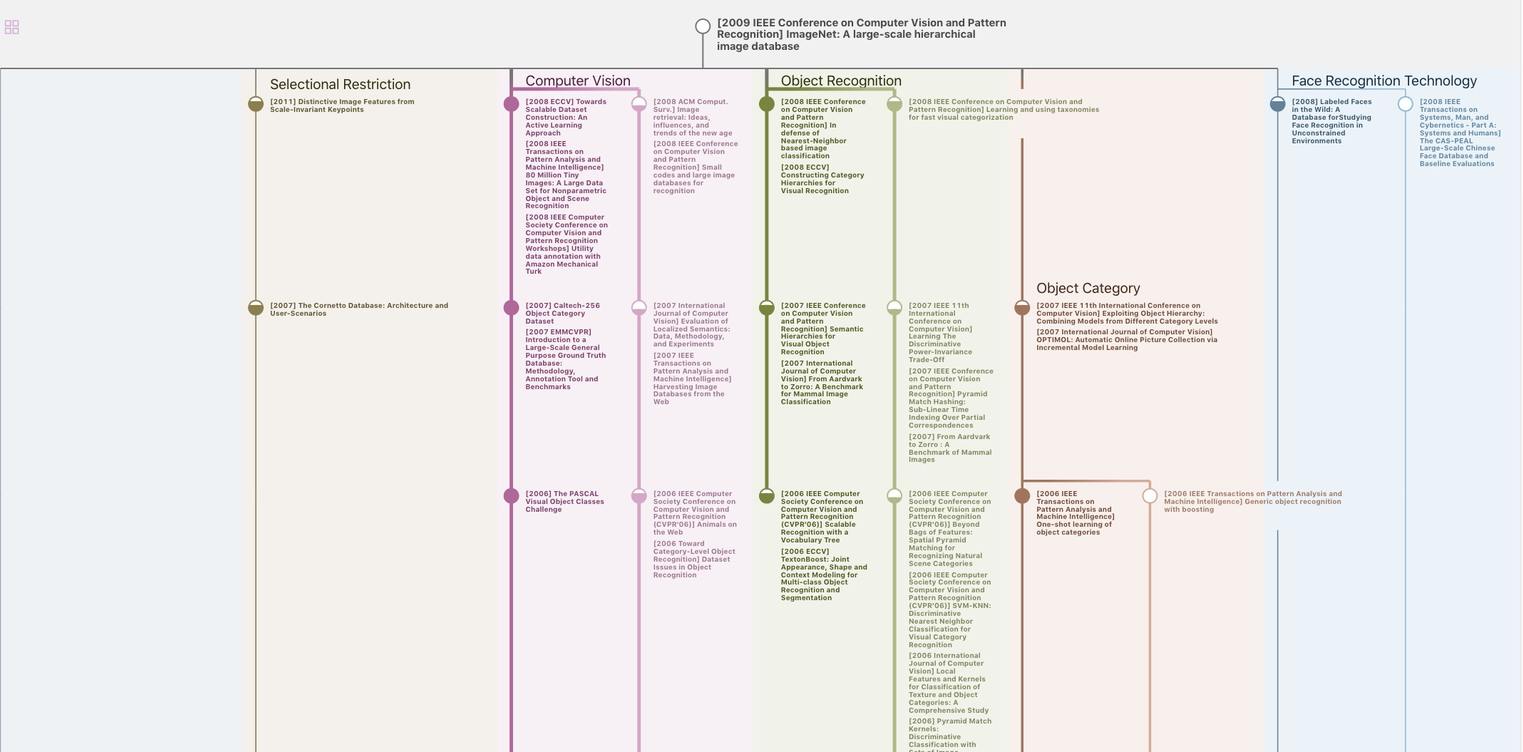
生成溯源树,研究论文发展脉络
Chat Paper
正在生成论文摘要