A Deep Learning Method for Modeling the Magnetic Signature of Spacecraft Equipment Using Multiple Magnetic Dipoles
IEEE Magnetics Letters(2021)
摘要
In this letter, a deep-learning-based neural network for magnetic dipole modeling (MDMnet) is introduced in the framework of dc magnetic cleanliness for space missions. The developed method targets modeling the static magnetic signature of a spacecraft unit that is obtained during the unit-level characterization stage of the extensive prelaunch electromagnetic compatibility test campaign. By employing synthetic magnetic flux density data generated by virtual dipole sources, the MDMnet can be trained to accurately estimate the magnetic parameters of real equipment based on its near magnetic flux density measurements. The target of the deep learning algorithm is, on the one hand, to effectively minimize the prediction errors (loss function) throughout the training process and, on the other hand, to enable the generalization of the model predictions, i.e., exhibit accurate model estimations with unseen magnetic induction data. Extensive simulations toward the stabilization of the MDMnet hyperparameters are outlined, and indicative model inferences employing artificial magnetic flux density data are carried out. Finally, the MDMnet can achieve a predictive accuracy of 0.8 mm with respect to the dipole localization and 1% with respect to the magnetic induction magnitude, verifying the potency of the developed method.
更多查看译文
关键词
Electromagnetics,deep learning,magnetic cleanliness,magnetic dipole modeling,magnetic signature
AI 理解论文
溯源树
样例
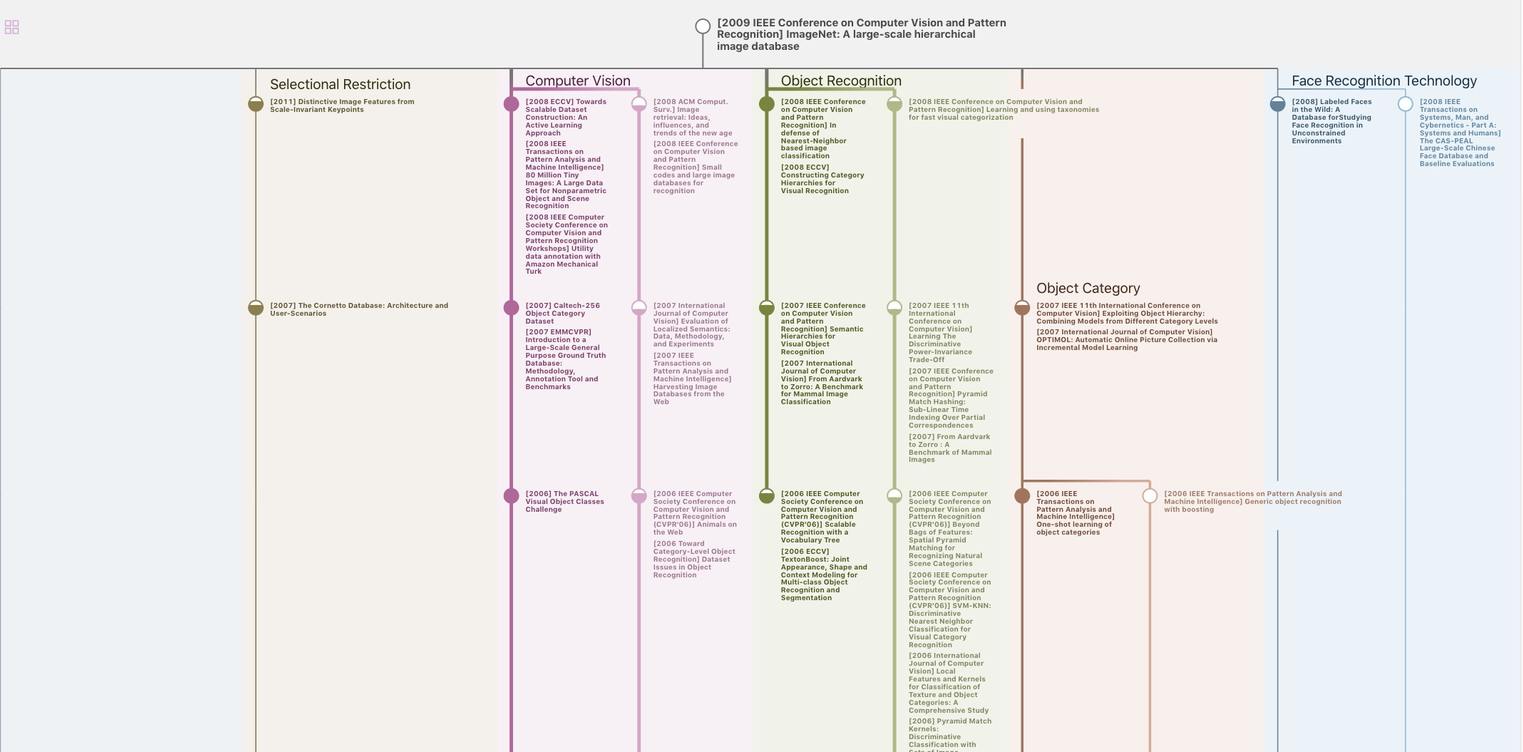
生成溯源树,研究论文发展脉络
Chat Paper
正在生成论文摘要