Modality-Wise Relational Reasoning For One-Shot Sensor-Based Activity Recognition
PATTERN RECOGNITION LETTERS(2021)
摘要
Deep learning concepts have been successfully transferred from the computer vision task to that of wearable human activity recognition (HAR) over the last few years. However, deep learning models require a large volume of annotated samples to be efficiently trained, while adding new activities results in training the whole network from scratch. In this paper, we study the use of one-shot learning techniques based on high-level features extracted by deep neural networks that rely on convolutional layers. Using these feature vectors as input we measure the similarity of two activities by computing their Euclidean distance, cosine similarity or applying self-attention to perceive the relations between the signals. We evaluate four different one-shot learning approaches using two publicly available HAR datasets, by keeping out of the training set several activity classes. Our results demonstrate that the model relying on modality-wise relational reasoning surpasses the other three, achieving 94.8% and 84.41% one-shot accuracy on UCL and PAMAP2 dataset respectively, while we demonstrate the model's sensitivity on fusing sensor modalities and provide explainable attention maps to display the modality-wise similarities.(c) 2021 Elsevier B.V. All rights reserved.
更多查看译文
关键词
Deep learning, One-shot learning, Human activity recognition, Relational reasoning, Self-attention
AI 理解论文
溯源树
样例
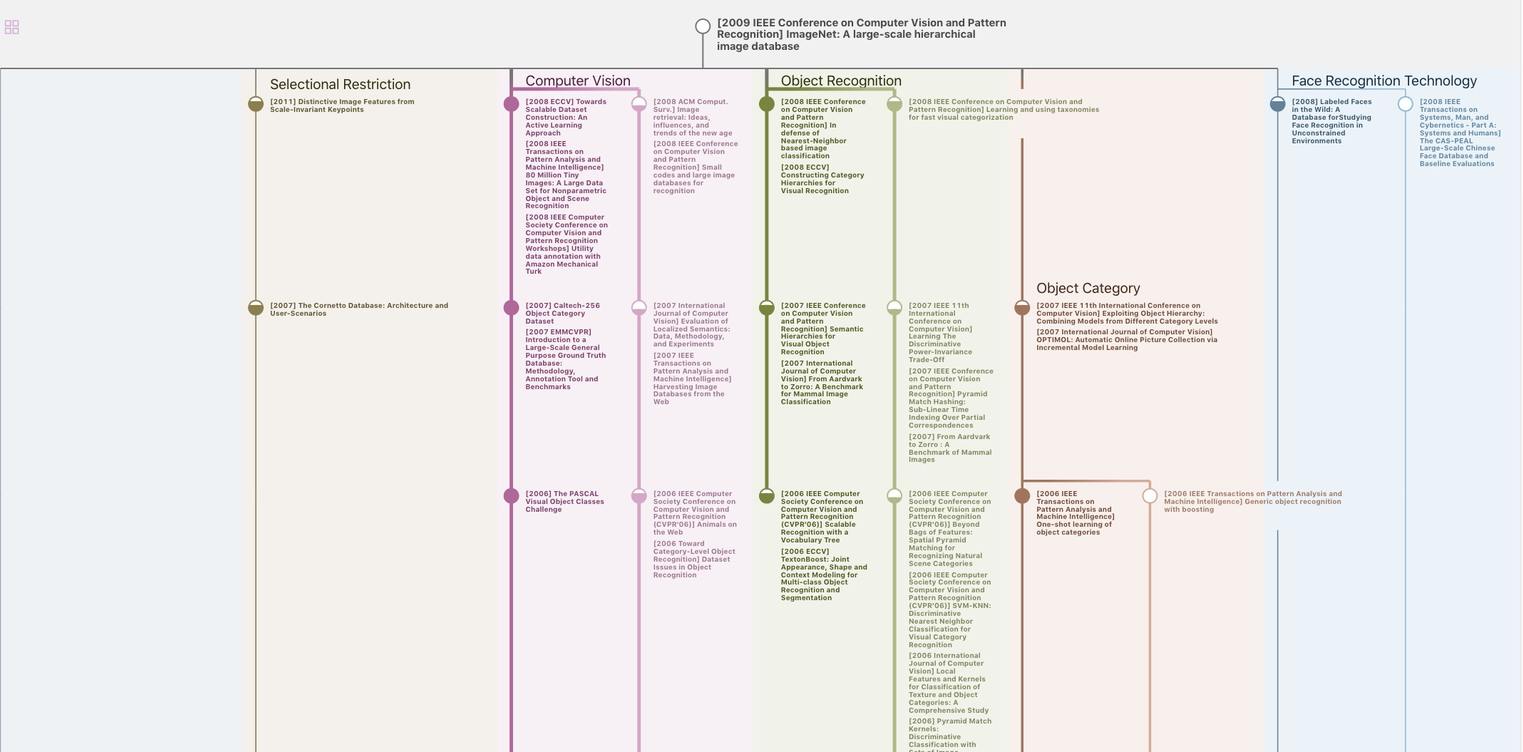
生成溯源树,研究论文发展脉络
Chat Paper
正在生成论文摘要