A Case Study Of Batch And Incremental Recommender Systems In Supermarket Data Under Concept Drifts And Cold Start
EXPERT SYSTEMS WITH APPLICATIONS(2021)
摘要
Recommender systems uncover relationships between users and items, thus allowing personalized recommendations. Nonetheless, users? preferences may change over time, the so-called concept drifts; or new users and items may appear, making the recommender system unable to accurately map the relationship between users and items due to the cold start problem. Consequently, concept drift and cold start are challenges that downgrade the recommender system?s predictive performance. This paper assesses existing approaches for collaborativefiltering recommender systems over a real supermarket dataset that exhibits both of the issues mentioned above. For this purpose, our comparative analysis encompasses batch and streaming learning approaches. As a result, we can observe that streaming-based models achieve better recommendation rates since these are tailored to fit the concept drift. More specifically, the predictive performance of streaming-based recommendations increases by up to 21% over those provided by batch methods. The supermarket dataset used in experimentation is also made publicly available for future studies and recommender systems comparisons.
更多查看译文
关键词
Collaborative filtering, Recommender systems, Positive-only feedback, Dataset
AI 理解论文
溯源树
样例
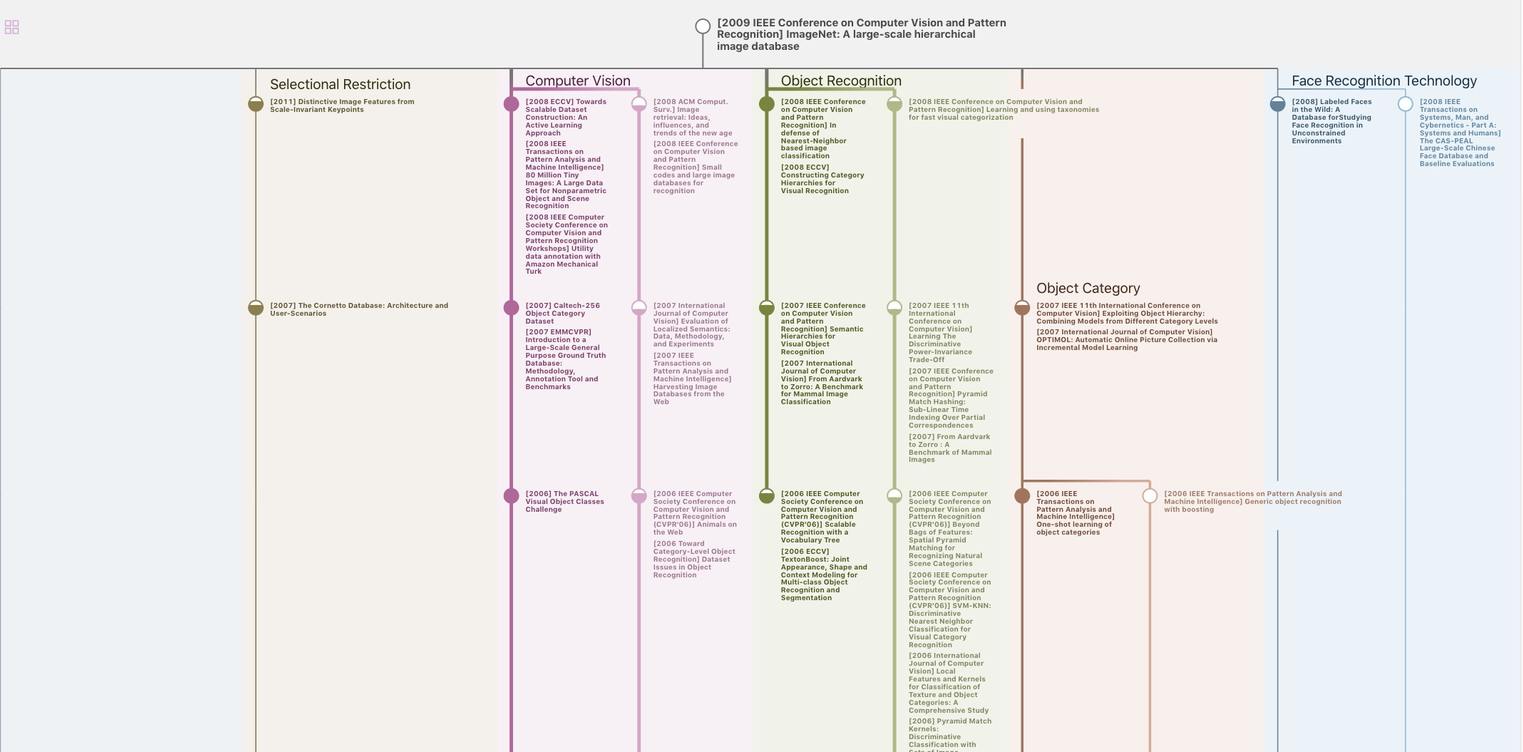
生成溯源树,研究论文发展脉络
Chat Paper
正在生成论文摘要