Machine learning identified materials descriptors for ferroelectricity
Acta Materialia(2021)
摘要
With aid of good materials descriptors, machine learning algorithms are able to accelerate the design of new materials and uncover underlying mechanisms. In the present study, we adopt machine learning methods to discover the most important materials descriptors for properties of ferroelectric materials. A regression study, in typical BaTiO3-based and K1/2Na1/2NbO3-based lead-free ceramics and lead-contained PbMg1/3Nb2/3TiO3-PbTiO3 ceramics, screens out three important materials descriptors determining ferroelectricity from 46 potential descriptors. The three descriptors of Matyonov-Batsanov electronegativity, ratio of valence electron number to nominal charge and core electron distance (Schubert) are confirmed to be dominant as well in classification of perovskite compounds into antiferroelectrics or not. The classification based on these descriptors exhibit an excellent accuracy of 96%, much higher than that of traditional criterion (89%) using tolerance factor and Pauling electronegativity. Furthermore, we propose a machine learning strategy based on our descriptors to predict the phase coexistence. The prediction probability after bootstrapping provides an effective approach to distinguish the phase boundaries and predict the phase ratio of coexisted phases. In all, we identified materials descriptors for ferroelectric materials, which is helpful to reveal the structure-property relationship of ferroelectric materials and guide the design of better ferroelectricity and piezoelectricity.
更多查看译文
关键词
Ferroelectricity,Machine learning,Materials descriptors
AI 理解论文
溯源树
样例
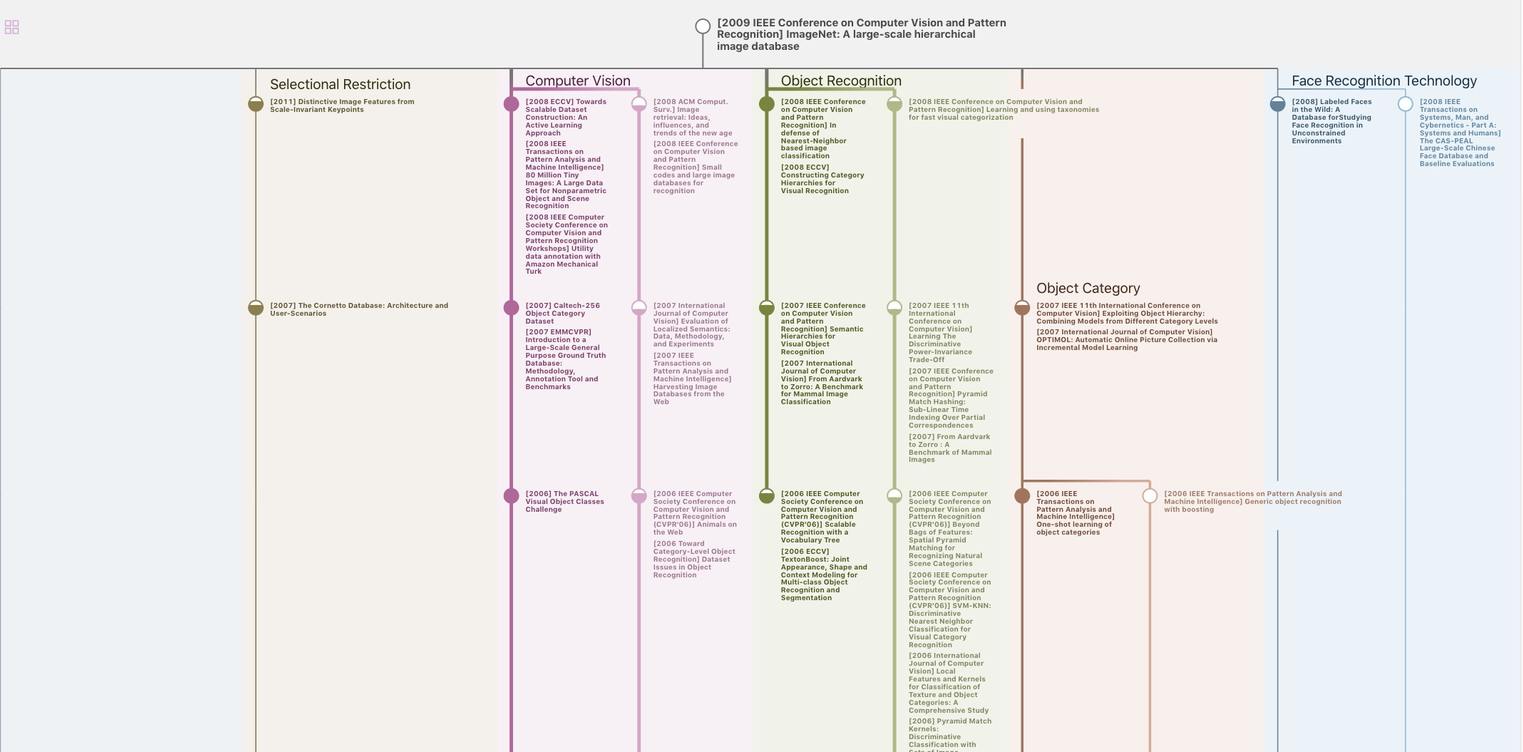
生成溯源树,研究论文发展脉络
Chat Paper
正在生成论文摘要