Deep Learning Approach For Detecting Malicious Activities Over Encrypted Secure Channels
IEEE ACCESS(2021)
摘要
Nowadays, most cyber attackers exploit secure communication channels to hide malicious activities and imitate the behaviors of a legitimate user. These attacks over a secure channel make networked systems more vulnerable to new threats and increase the possibility of significant damage to other end users. Traditional TCP/IP-level traffic inspections do not suffice in investigating a secure sockets layer (SSL) conversation because the SSL conversation data is encrypted by a public key system and the SSL uses its own data unit of an SSL record. In this paper, we propose a novel malicious SSL traffic detection method, which reassembles SSL records from captured IP packets and inspects the characteristics of SSL records using a deep learning method. After an SSL record is reassembled from a single or multiple IP packets, the proposed method extracts unencrypted contents of the reassembled record and generates a sequence of unencrypted data from successive SSL records for deep learning-based classification. The sequences of SSL records are encoded using a long short-term memory autoencoder, and then an encoded feature map is generated for each SSL flow. These feature maps are forwarded to the convolutional neural network-based classifier to determine whether the SSL flow is malicious or not. The experiment shows that our proposed approach has a great separability between benign and malicious traffic flows on an encrypted SSL channel.
更多查看译文
关键词
Cryptography, Inspection, Protocols, Deep learning, Payloads, Feature extraction, Computer crime, Cryptographic protocols, deep learning, intrusion detection, network security
AI 理解论文
溯源树
样例
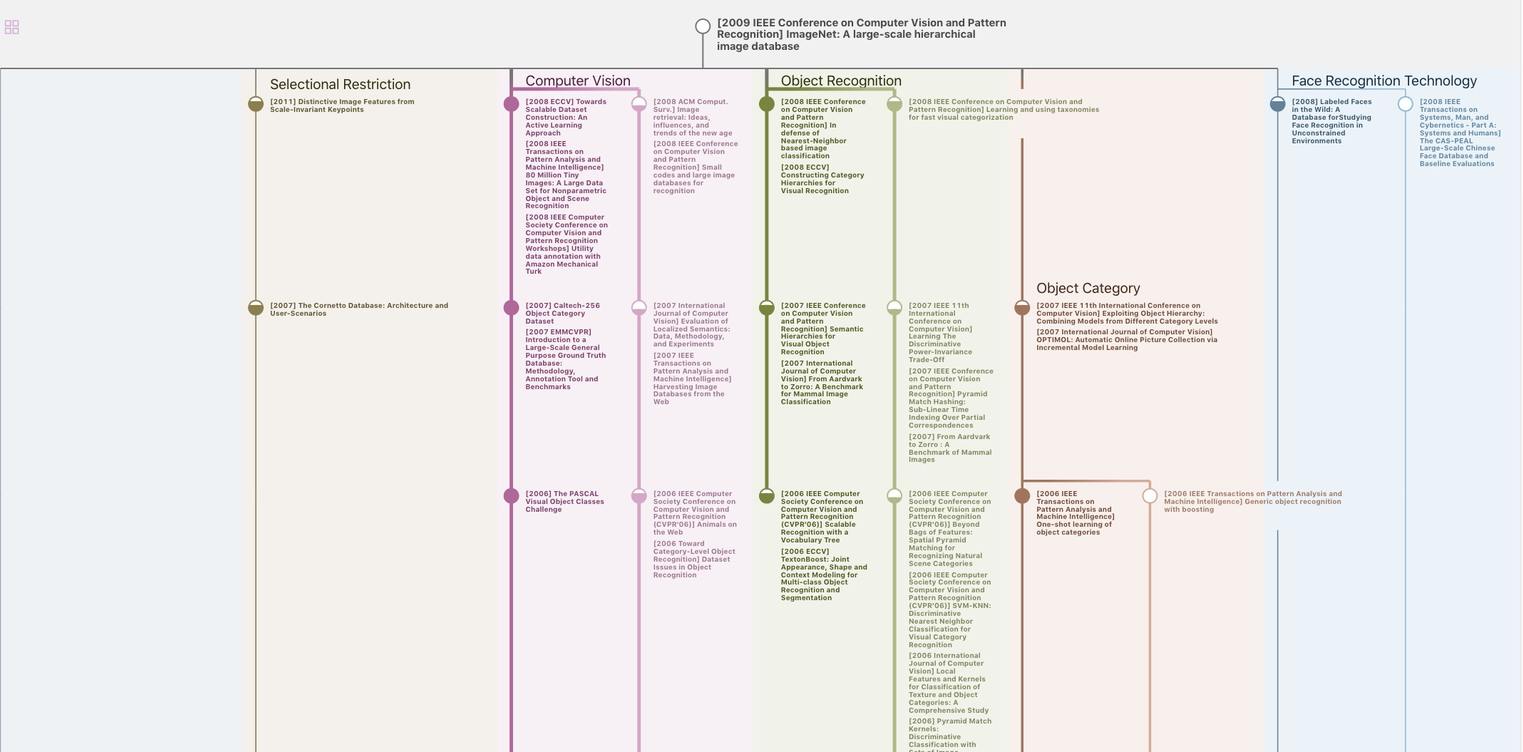
生成溯源树,研究论文发展脉络
Chat Paper
正在生成论文摘要