Construction Of A Virtual Opioid Bioprofile: A Data-Driven Qsar Modeling Study To Identify New Analgesic Opioids
ACS SUSTAINABLE CHEMISTRY & ENGINEERING(2021)
摘要
Compared to traditional experimental approaches, computational modeling is a promising strategy to efficiently prioritize new candidates with low cost. In this study, we developed a novel data mining and computational modeling workflow proven to be applicable by screening new analgesic opioids. To this end, a large opioid data set was used as the probe to automatically obtain bioassay data from the PubChem portal. There were 114 PubChem bioassays selected to build quantitative structure-activity relationship (QSAR) models based on the testing results across the probe compounds. The compounds tested in each bioassay were used to develop 12 models using the combination of three machine learning approaches and four types of chemical descriptors. The model performance was evaluated by the coefficient of determination (R-2) obtained from S-fold cross-validation. In total, 49 models developed for 14 bioassays were selected based on the criteria and were identified to be mainly associated with binding affinities to different opioid receptors. The models for these 14 bioassays were further used to fill data gaps in the probe opioids data set and to predict general drug compounds in the DrugBank data set. This study provides a universal modeling strategy that can take advantage of large public data sets for computer-aided drug design (CADD).
更多查看译文
关键词
Opioid, Big data, QSAR, Data mining, Machine learning, Profiling
AI 理解论文
溯源树
样例
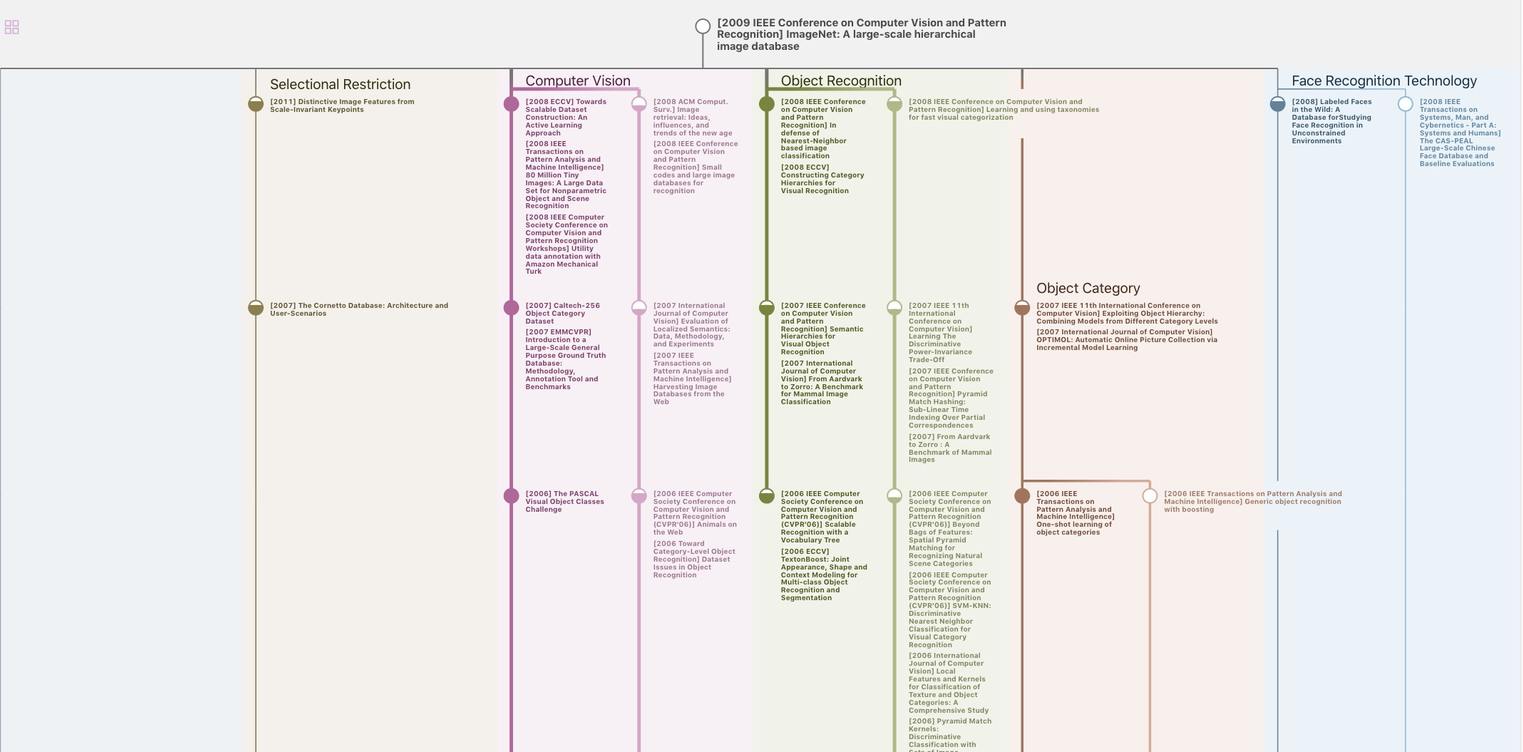
生成溯源树,研究论文发展脉络
Chat Paper
正在生成论文摘要