Graph-Based Deep Learning Frameworks For Molecules And Solid-State Materials
COMPUTATIONAL MATERIALS SCIENCE(2021)
摘要
Recent years have witnessed the rapid increase in the application of deep learning in atomistic systems including both molecules and solid-state materials. The use of graphs and associated design of message passing strategies have enabled multiple deep learning frameworks to achieve reliable and efficient predictions of materials properties with a much smaller cost compared with first-principles atomistic simulations. In this review, we will focus on recent development of graph-based deep learning frameworks and their applications for both molecules and solid-state material systems. The history of the development of graph-based representations for molecules and crystals will be introduced. Essential learning processes defined by the so-called message passing will be reviewed, based on which the performance of different models will be compared. Furthermore, recent development of graph learning frameworks that incorporate material information beyond atom level will be introduced. Current challenges and future perspectives on this emerging field at the crossroad of material science, physics, chemistry, and computer science will be given, with an emphasize on how multiple tiers of material information can be organized and combined in a graph neural network setup.
更多查看译文
关键词
Graph neural network, Deep learning for materials science, Machine learning
AI 理解论文
溯源树
样例
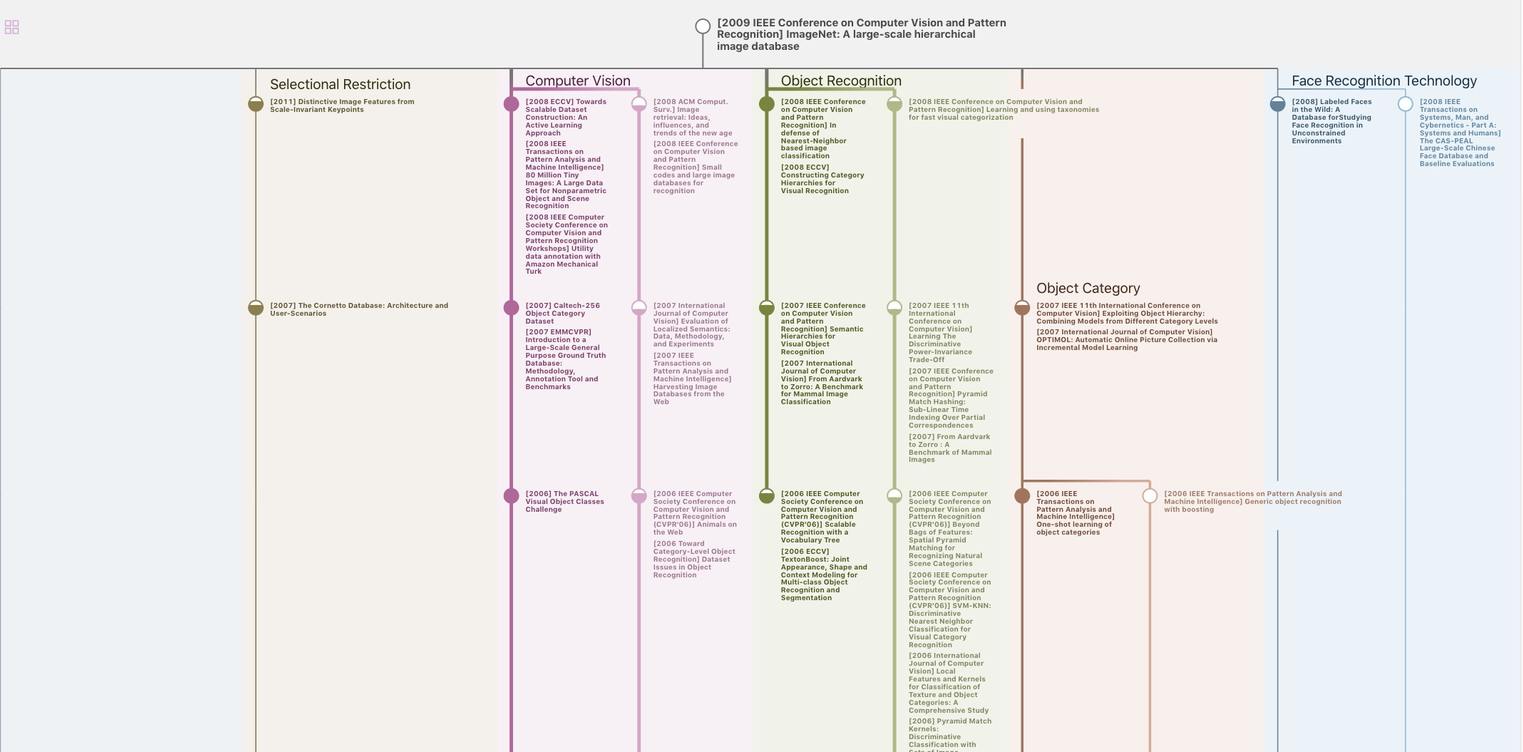
生成溯源树,研究论文发展脉络
Chat Paper
正在生成论文摘要