Reliable Sensing Data Fusion Through Robust Multiview Prototype Learning
IEEE Transactions on Industrial Informatics(2022)
摘要
Due to emerging development of intelligent sensing technologies in Internet of Things, multisensor cooperation has been widely deployed in applications. Although multisensor information fusion can be addressed by multiview learning, its performance tends to degrade if any one sensor is disturbed with annoying noises by the environment or other factors. Therefore, fusing these cross-sensor data in a reliable and secure manner while removing those noises is crucial. Although there have been outlier-against multiview works proposed, most of them suffer from redundant parameters or performance degradation. Even worse, few of them have considered the complementary information across the sensors. In this article, we argue that in multiview information fusion, not only the clean data, but also those outliers share the same prototypes in a common space, except that the outliers are disturbed with noises. To this end, we propose a type of robust multiview prototype (RMVP) learning to fuse the sensing data while removing the noises automatically in the learning process. Specifically, in RMVP, projection matrices are designed for each sensing view to sketch the data prototypes. In addition, one auxiliary margin matrix is modeled for each sensing view to capture its data noises through penalizing a sparsity regularization on it. Afterwards, an alternating algorithm is presented to solve the proposed model. Finally, extensive experiments on intelligent sensing data sets are conducted to testify the effectiveness of the proposed method.
更多查看译文
关键词
Internet of Things,multiview learning,outlier detection,prototype learning,reliable sensing data fusion
AI 理解论文
溯源树
样例
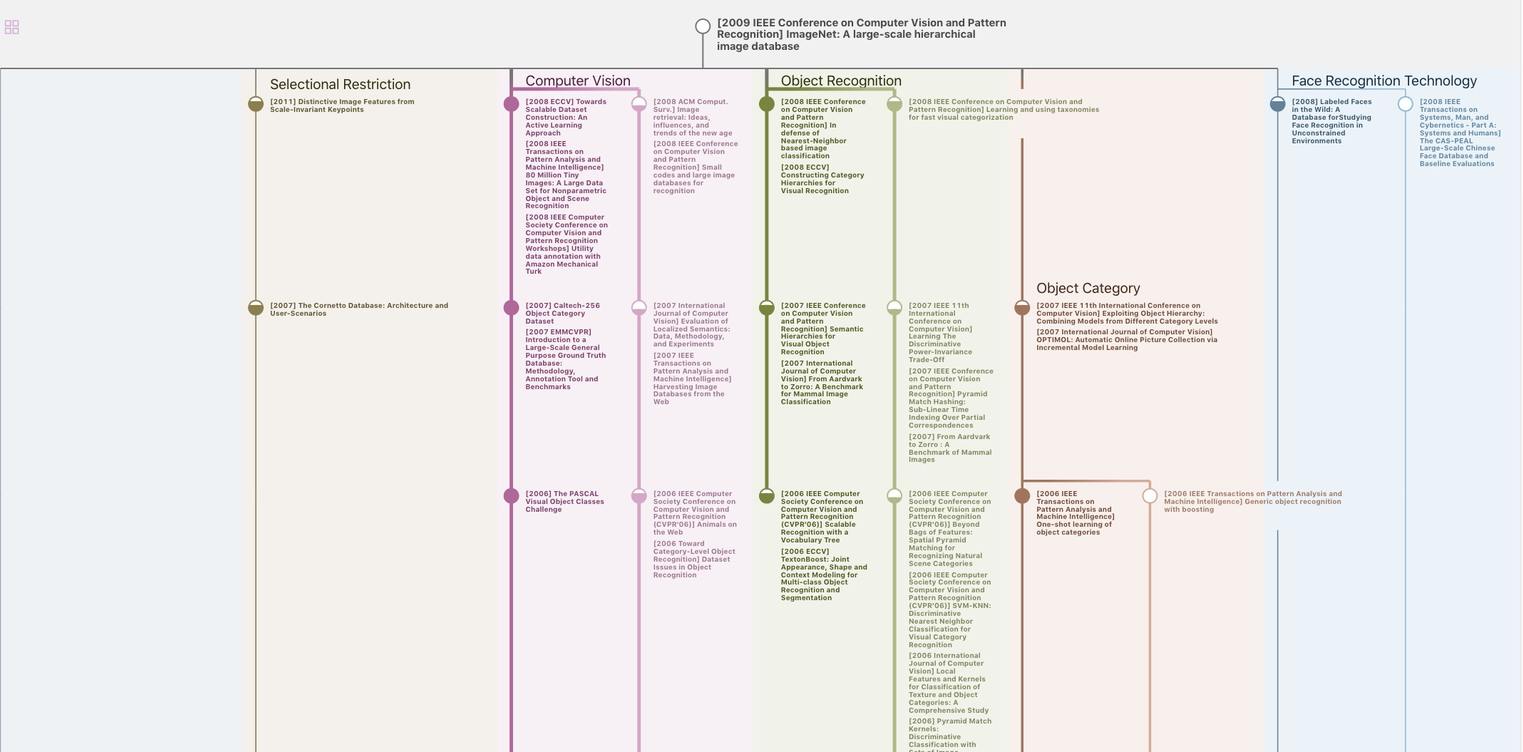
生成溯源树,研究论文发展脉络
Chat Paper
正在生成论文摘要