Regression Bugs Are In Your Model! Measuring, Reducing and Analyzing Regressions In NLP Model Updates.
Annual Meeting of the Association for Computational Linguistics(2021)
摘要
Behavior of deep neural networks can be inconsistent between different versions. Regressions during model update are a common cause of concern that often over-weigh the benefits in accuracy or efficiency gain. This work focuses on quantifying, reducing and analyzing regression errors in the NLP model updates. Using negative flip rate as regression measure, we show that regression has a prevalent presence across tasks in the GLUE benchmark. We formulate the regression-free model updates into a constrained optimization problem, and further reduce it into a relaxed form which can be approximately optimized through knowledge distillation training method. We empirically analyze how model ensemble reduces regression. Finally, we conduct CheckList behavioral testing to understand the distribution of regressions across linguistic phenomena, and the efficacy of ensemble and distillation methods.
更多查看译文
关键词
nlp model! updates,regressions
AI 理解论文
溯源树
样例
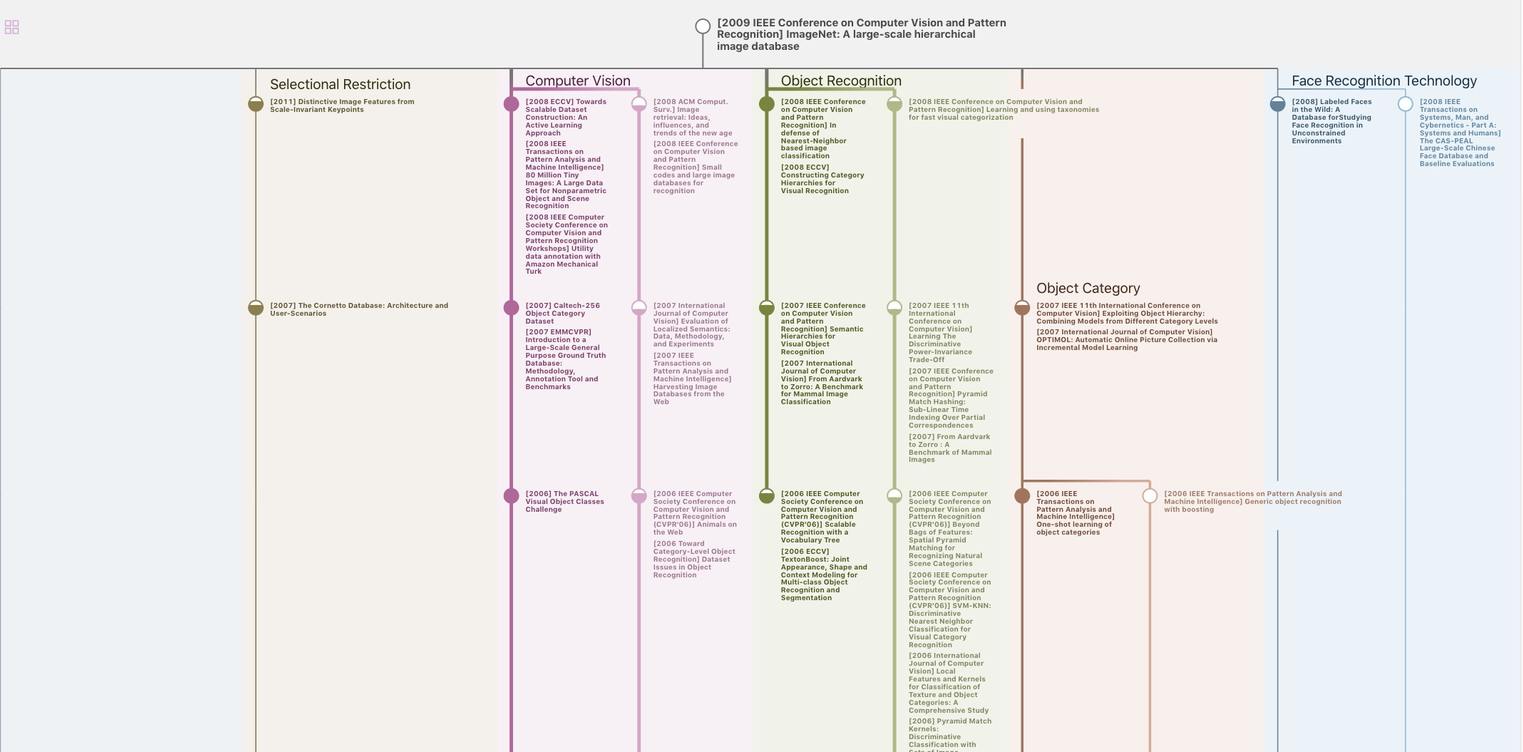
生成溯源树,研究论文发展脉络
Chat Paper
正在生成论文摘要