A Deep Learning-Based Auto-Segmentation System For Organs-At-Risk On Whole-Body Computed Tomography Images For Radiation Therapy
RADIOTHERAPY AND ONCOLOGY(2021)
摘要
Background and purpose: Delineating organs at risk (OARs) on computed tomography (CT) images is an essential step in radiation therapy; however, it is notoriously time-consuming and prone to interobserver variation. Herein, we report a deep learning-based automatic segmentation (AS) algorithm (WBNet) that can accurately and efficiently delineate all major OARs in the entire body directly on CT scans. Materials and methods: We collected 755 CT scans of the head and neck, thorax, abdomen, and pelvis and manually delineated 50 OARs on the CT images. The CT images with contours were split into training and test sets consisting of 505 and 250 cases, respectively, to develop and validate WBNet. The volumetric Dice similarity coefficient (DSC) and 95th-percentile Hausdorff distance (95% HD) were calculated to evaluate delineation quality for each OAR. We compared the performance of WBNet with three AS algorithms: one commercial multi-atlas-based automatic segmentation (ABAS) software, and two deep learning-based AS algorithms, namely, AnatomyNet and nnU-Net. We have also evaluated the time saving and dose accuracy of WBNet. Results: WBNet achieved average DSCs of 0.84 and 0.81 on in-house and public datasets, respectively, which outperformed ABAS, AnatomyNet, and nnU-Net. WBNet could reduce the delineation time significantly and perform well in treatment planning, with clinically acceptable dose differences compared with those in manual delineation. Conclusion: This study shows the feasibility and benefits of using WBNet in clinical practice. (c) 2021 The Authors. Published by Elsevier B.V. Radiotherapy and Oncology 160 (2021) 175-184 This is an open access article under the CC BY-NC-ND license (http://creativecommons.org/licenses/by-nc-nd/4.0/).
更多查看译文
关键词
Automatic segmentation, Organs at risk, Deep learning
AI 理解论文
溯源树
样例
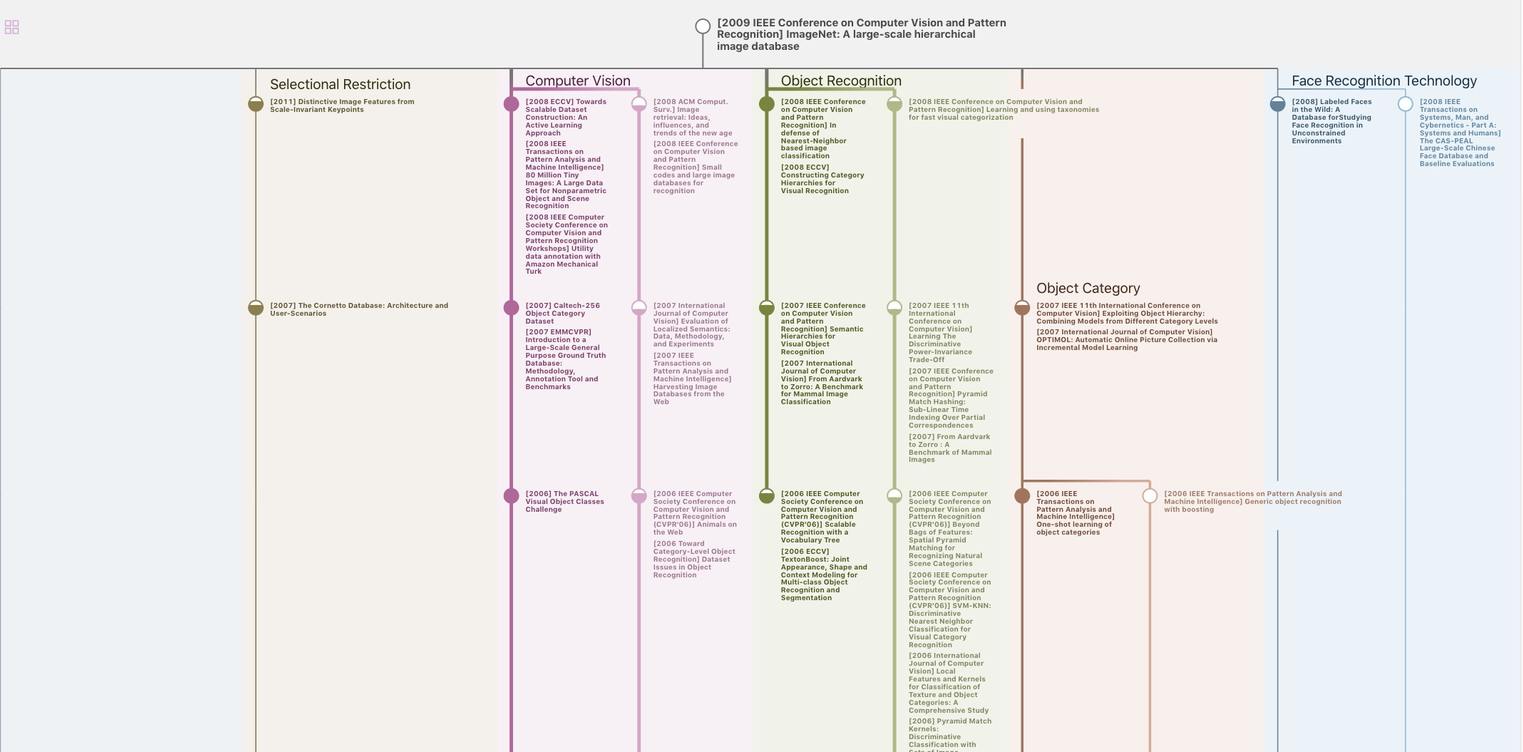
生成溯源树,研究论文发展脉络
Chat Paper
正在生成论文摘要