An Integrated Approach Of Deep Learning And Symbolic Analysis For Digital Pdf Table Extraction
2020 25TH INTERNATIONAL CONFERENCE ON PATTERN RECOGNITION (ICPR)(2020)
摘要
Deep learning has shown great success at interpreting unstructured data such as object recognition in images. Symbolic/logical-reasoning techniques have shown great success in interpreting structured data such as table extraction in webpages, custom text files, spreadsheets. The tables in PDF documents are often generated from such structured sources (text-based Word/LATEX documents, spreadsheets, webpages) but end up being unstructured. We thus explore novel combinations of deep learning and symbolic reasoning techniques to build an effective solution for PDF table extraction. We evaluate effectiveness without granting partial credit for matching part of a table (which may cause silent errors in downstream data processing). Our method achieves a 0.725 F-1 score (vs. 0339 for the state-of-the-art) on detecting correct table bounds-a much stricter metric than the common one of detecting characters within tables-in a well known public benchmark (ICDAR 2013) and a 0.404 F-1 score (vs. 0.144 for the state-of-the-art) on our private benchmark with more widely varied table structures.
更多查看译文
关键词
spreadsheets,PDF documents,structured sources,Web pages,deep learning,symbolic reasoning,downstream data processing,table structures,symbolic analysis,digital PDF table extraction,unstructured data,object recognition,structured data,custom text files,table bounds,logical reasoning,text-based word,LATEX documents,F1 score,ICDAR 2013
AI 理解论文
溯源树
样例
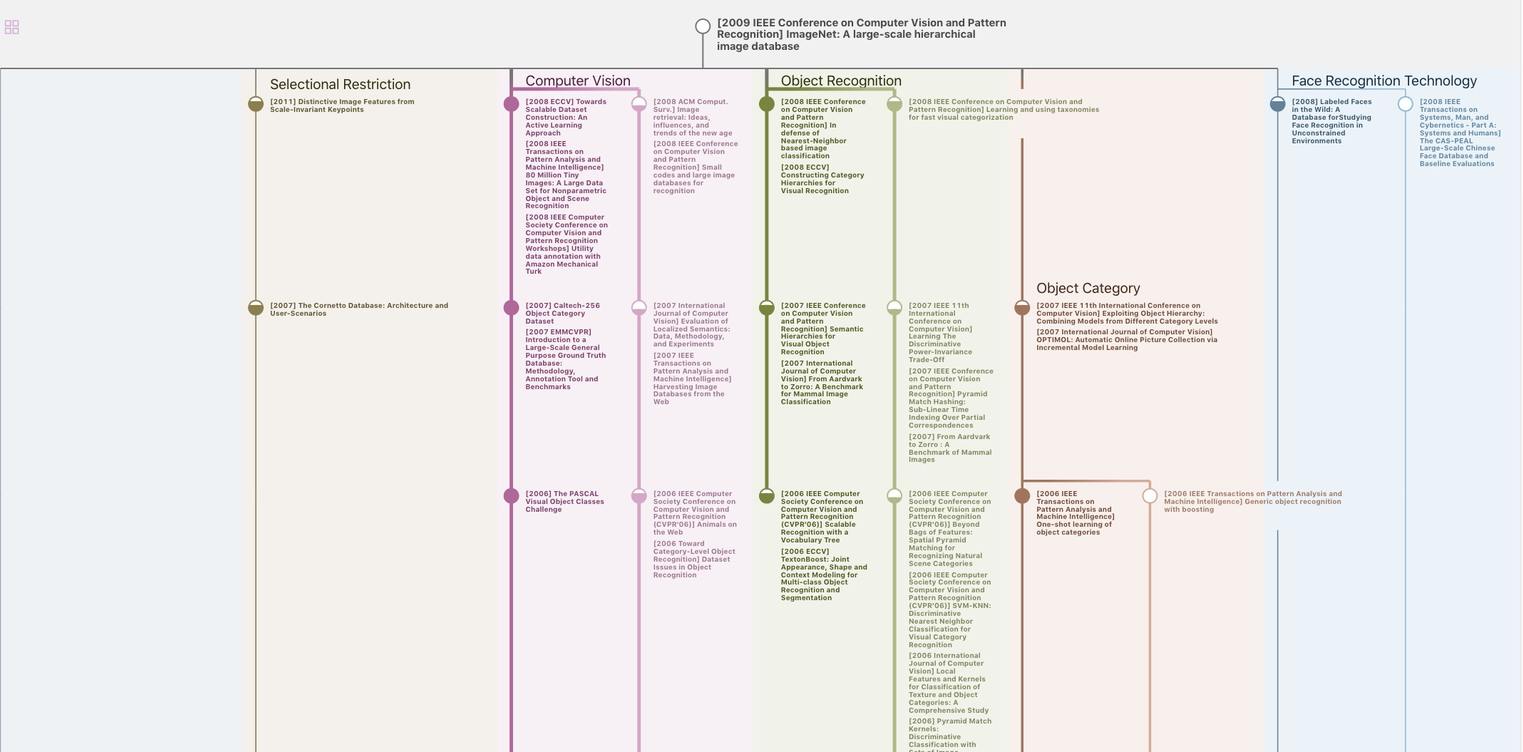
生成溯源树,研究论文发展脉络
Chat Paper
正在生成论文摘要