Encoding Brain Networks Through Geodesic Clustering Of Functional Connectivity For Multiple Sclerosis Classification
2020 25TH INTERNATIONAL CONFERENCE ON PATTERN RECOGNITION (ICPR)(2020)
摘要
An important task in brain connectivity research is the classification of patients from healthy subjects. In this work, we present a two-step mathematical framework allowing to discriminate between two groups of people with an application to multiple sclerosis. The proposed approach exploits the properties of the connectivity matrices determined using the covariances between signals of a fixed set of brain areas. These positive semi-definite matrices lay on a Riemannian manifold, allowing to use a geodesic distance defined on this space. In order to generate a vector representation useful for classification purposes, but still preserving the network structure, we encoded the data exploiting the network attractors determined by a geodesic clustering of connectivity matrices. Then clustering centroids were used as a dictionary allowing to encode subject's connectivity matrices as a vector of geodesic distances. A Linear Support Vector Machine was then used to perform classification between subjects. To demonstrate the advantage of using geodesic metrics in this framework, we conducted the same analysis using Euclidean metric. Experimental results validate the fact that employing geodesic metric in this framework leads to a higher classification performance, whereas performance with a Euclidean metric was sub-optimal.
更多查看译文
关键词
brain areas,positive semidefinite matrices,geodesic distance,vector representation,classification purposes,network structure,network attractors,geodesic clustering,connectivity matrices,Linear Support Vector Machine,geodesic metrics,higher classification performance,encoding brain networks,functional connectivity,multiple sclerosis classification,brain connectivity research,healthy subjects,two-step mathematical framework
AI 理解论文
溯源树
样例
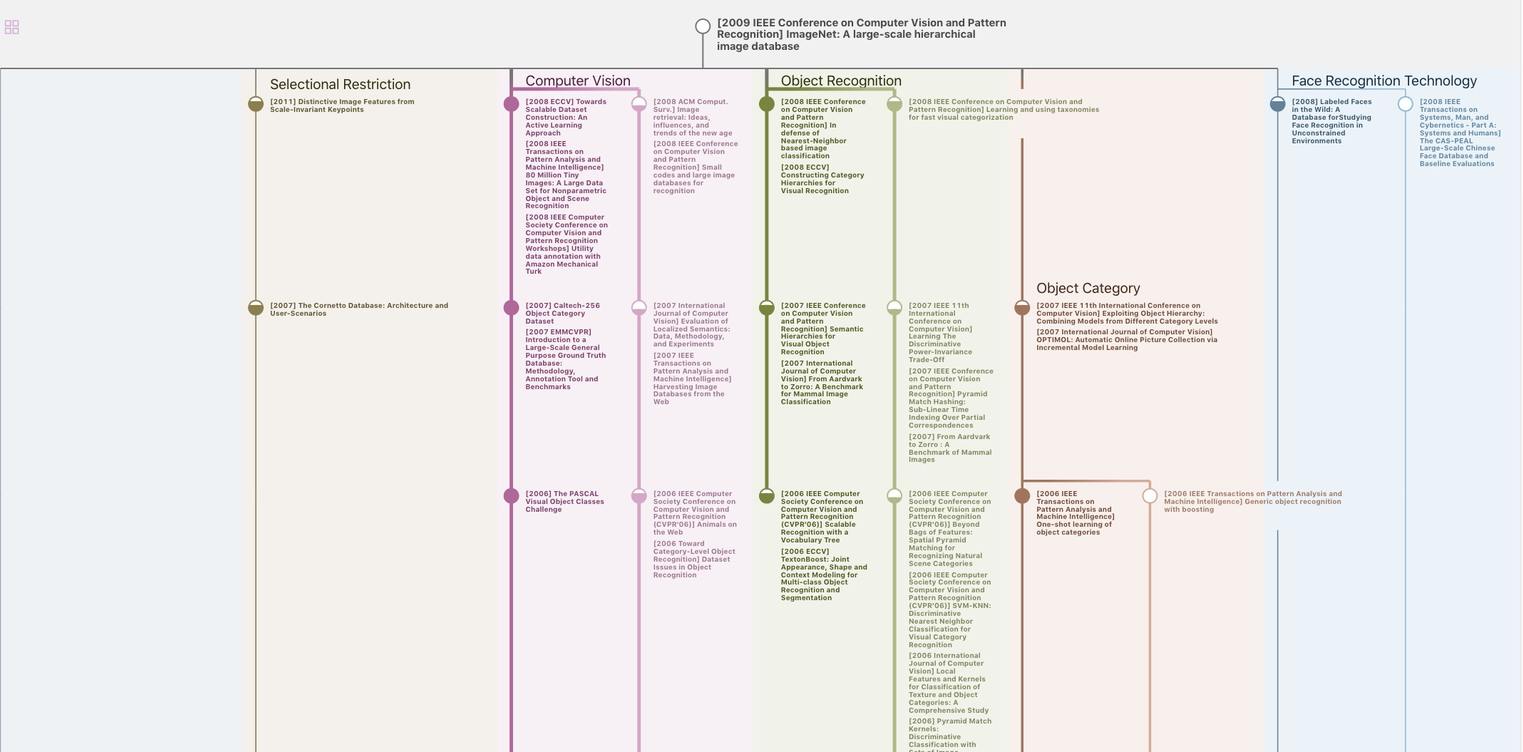
生成溯源树,研究论文发展脉络
Chat Paper
正在生成论文摘要