Towards Efficient 3D Point Cloud Scene Completion via Novel Depth View Synthesis
2020 25TH INTERNATIONAL CONFERENCE ON PATTERN RECOGNITION (ICPR)(2021)
摘要
3D point cloud completion has been a long-standing challenge at scale, and corresponding per-point supervised training strategies suffered from cumbersome annotations. 2D supervision has recently emerged as a promising alternative for 3D tasks, but specific approaches for 3D point cloud completion still remain to be explored. To overcome these limitations, we propose an end-to-end method that directly lifts a single depth map to a completed point cloud. With one depth map as input, a multiway novel depth view synthesis network (NDVNet) is designed to infer coarsely completed depth maps under various viewpoints. Meanwhile, a geometric depth perspective rendering module is introduced to utilize the raw input depth map to generate a re-projected depth map for each view. Therefore, the two parallelly generated depth maps for each view are further concatenated and refined by a depth completion network (DCNet). The final completed point cloud is fused from all refined depth views. Experimental results demonstrate the effectiveness of our proposed approach composed of aforementioned components, to produce high-quality, state-of-the-art results on the popular SUNCG benchmark.
更多查看译文
关键词
refined depth views,efficient 3D point cloud scene completion,3D point cloud completion,per-point supervised training strategies,end-to-end method,single depth map,multiway novel depth view synthesis network,coarsely completed depth maps,geometric depth perspective rendering module,raw input depth map,depth completion network,final completed point cloud
AI 理解论文
溯源树
样例
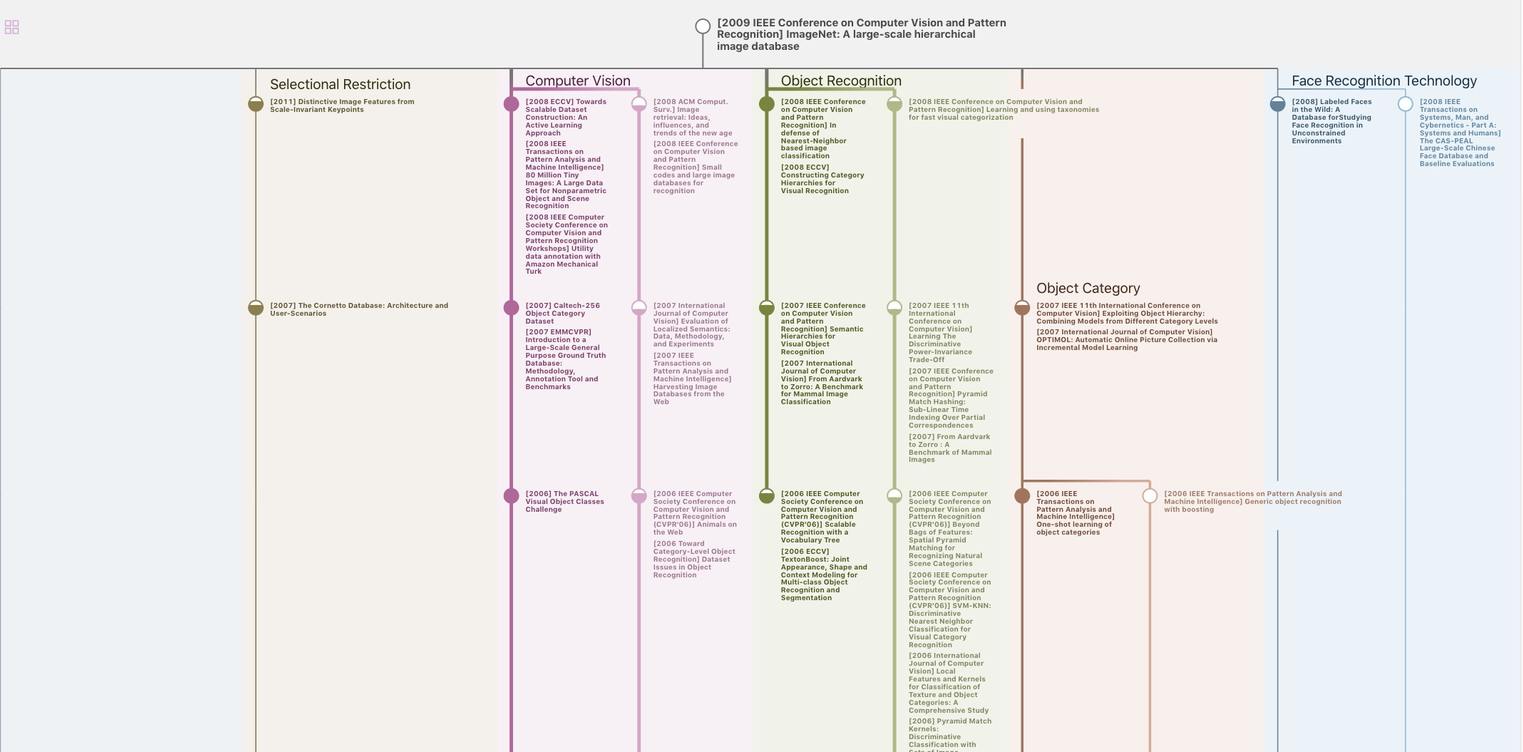
生成溯源树,研究论文发展脉络
Chat Paper
正在生成论文摘要