Mrp-Net: A Light Multiple Region Perception Neural Network For Multi-Label Au Detection
2020 25TH INTERNATIONAL CONFERENCE ON PATTERN RECOGNITION (ICPR)(2020)
摘要
Facial Action Units (AUs) are of great significance in communication. Automatic AU detection can improve the understanding of psychological condition and emotional status. Recently, a number of deep learning methods have been proposed to take charge with problems in automatic AU detection. Several challenges, like unbalanced labels and ignorance of local information, remain to be addressed. In this paper, we propose a fast and light neural network called MRP-Net, which is an end-to-end trainable method for facial AU detection to solve these problems. First, we design a Multiple Region Perception (MRP) module aimed at capturing different locations and sizes of features in the deeper level of the network without facial landmark points. Then, in order to balance the positive and negative samples in the large dataset, a batch balanced method adjusting the weight of every sample in one batch in our loss function is suggested. Experimental results on two popular AU datasets, BP4D and DISFA prove that MRP-Net outperforms state-of-the-art methods. Compared with the best method, not only does MRP-Net have an average F1 score improvement of 2.95% on BP4D and 5.43% on DISFA, and it also decreases the number of network parameters by 54.62% and the number of network FLOPs by 19.6%.
更多查看译文
关键词
F1 score improvement,BP4D,DISFA,multilabel AU detection,MRP-Net,AU datasets,network FLOP,light Multiple Region Perception neural network,batch balanced method,facial landmark points,Multiple Region Perception module,facial AU detection,end-to-end trainable method,light neural network,deep learning methods,emotional status,psychological condition,automatic AU detection,Facial Action Units
AI 理解论文
溯源树
样例
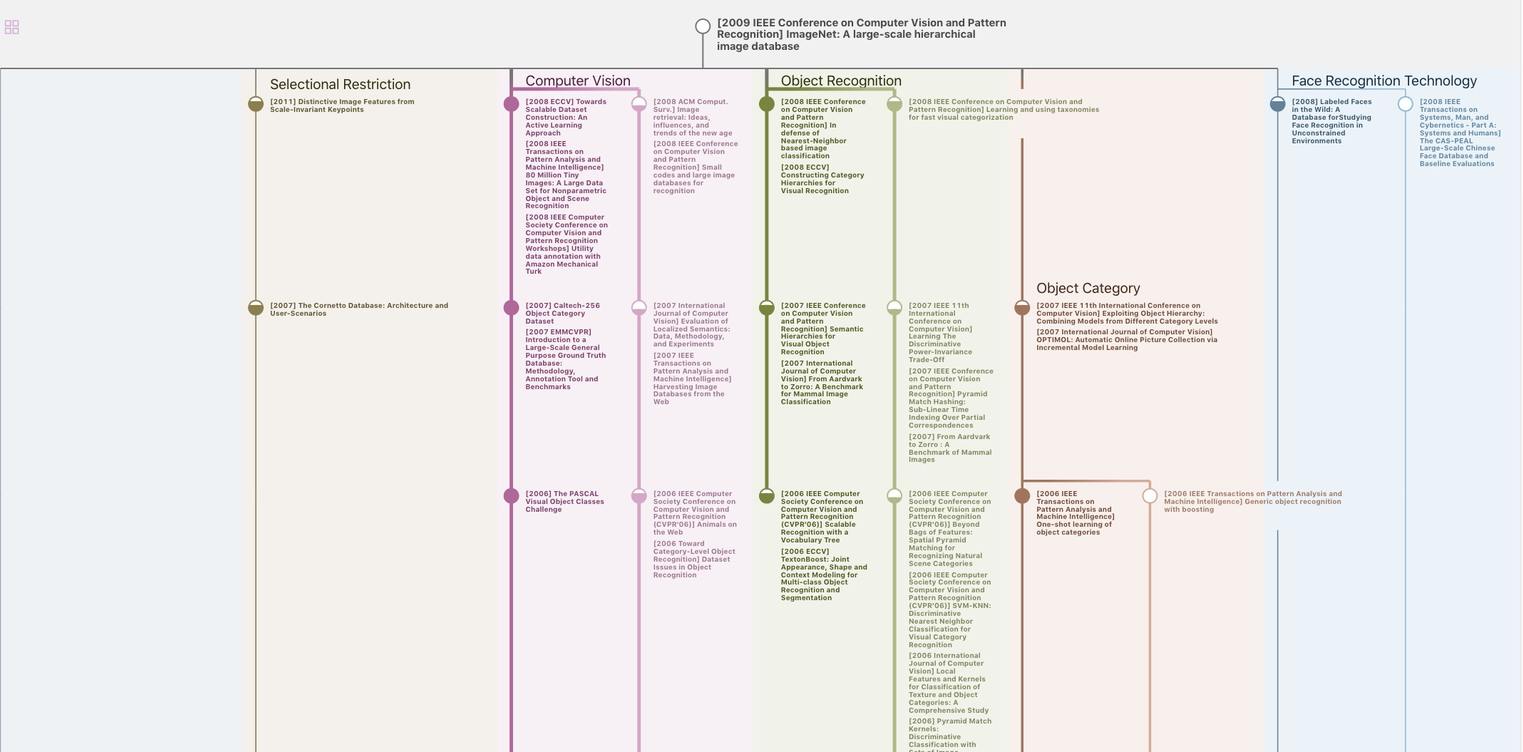
生成溯源树,研究论文发展脉络
Chat Paper
正在生成论文摘要