Real-Time End-To-End Lane Id Estimation Using Recurrent Networks
2020 25TH INTERNATIONAL CONFERENCE ON PATTERN RECOGNITION (ICPR)(2020)
摘要
Acquiring information about the road lane structure is a crucial step for autonomous navigation. To this end, several approaches tackle this task from different perspectives such as lane marking detection or semantic lane segmentation. However, to the best of our knowledge, there is yet no purely vision based end-to-end solution to answer the precise question: How to estimate the relative number or "ID" of the current driven lane within a multi-lane road or a highway?In this work, we propose a real-time, vision-only (i.e. monocular camera) solution to the problem based on a dual left-right convention. We interpret this task as a classification problem by limiting the maximum number of lane candidates to eight. Our approach is designed to meet low-complexity specifications and limited runtime requirements. It harnesses the temporal dimension inherent to the input sequences to improve upon high-complexity state-of-the-art models. We achieve more than 95% accuracy on a challenging test set with extreme conditions and different routes.
更多查看译文
关键词
time end-to-end lane ID estimation,recurrent networks,road lane structure,semantic lane segmentation,purely vision based end-to-end solution,current driven lane,multilane road,lane candidates
AI 理解论文
溯源树
样例
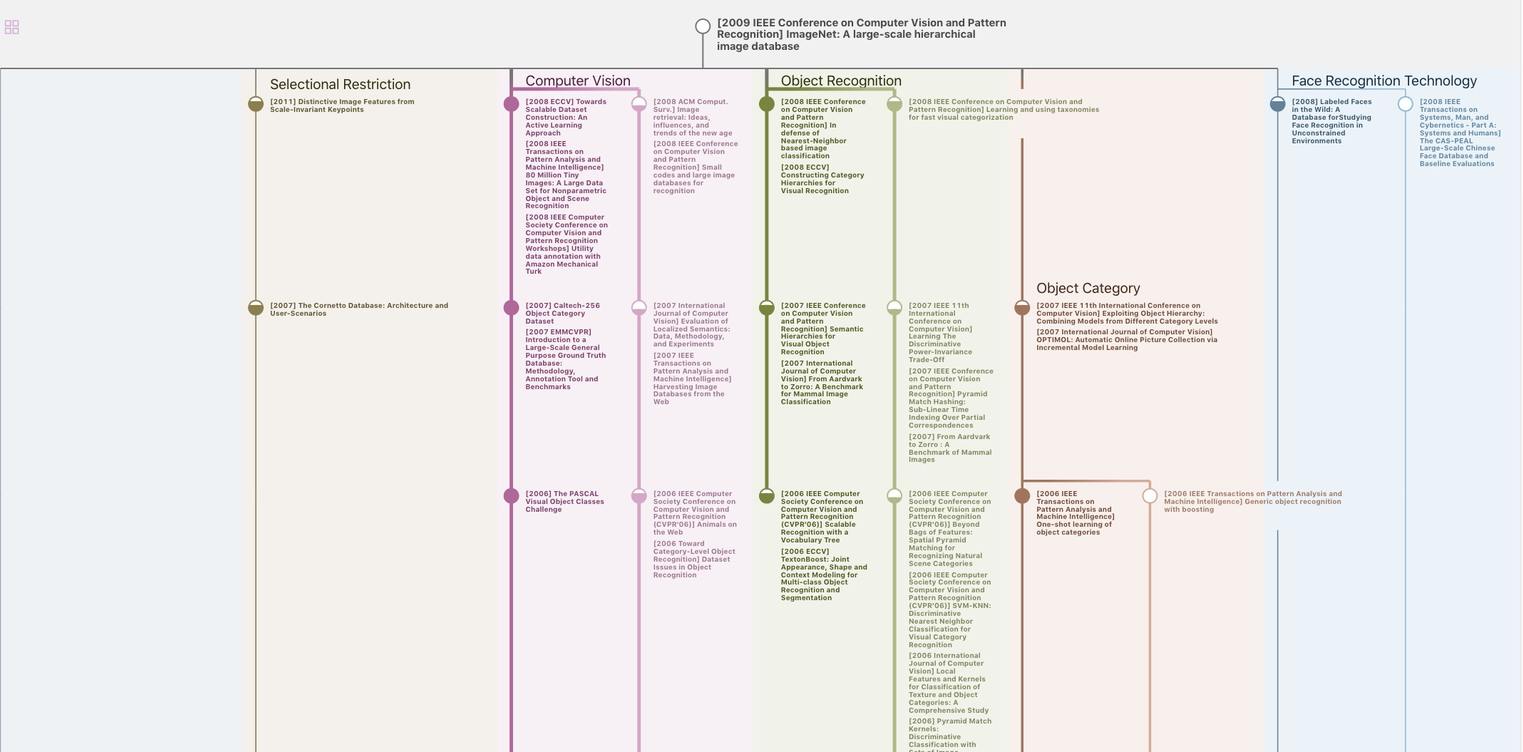
生成溯源树,研究论文发展脉络
Chat Paper
正在生成论文摘要