Segmenting Kidney On Multiple Phase Ct Images Using Ulbnet
2020 25TH INTERNATIONAL CONFERENCE ON PATTERN RECOGNITION (ICPR)(2020)
摘要
Segmentation of kidney on CT images is critical to computer-assisted surgical planning for kidney interventional therapy. Segmenting kidney manually is impractical in clinical, automatic segmentation is desirable. U-Net has been successful in medical image segmentation and is a promising candidate for the task. However, semantic gap still exists, especially when multiple phase images or multiple center images are involved. In this paper, we proposed an ULBNet to reduce the semantic gap and to improve segmentation performance. The proposed architecture includes new skip connections of local binary convolution (LBC). We also proposed a novel strategy of fast retraining a model for a new task without manually labelling required. We evaluated the network for kidney segmentation on multiple phase CT images. ULBNet resulted in an overall accuracy of 98.0% with comparison to Resunet 97.5 degrees A Specifically, on the plain phase CT images, 98.1% resulted from ULBNet and 97.6% from Resunet; on the corticomedullay phase images, 97.8% from ULBNet and 97.2% from Resunet; on the nephrographic phase images, 97.6% from ULBNet and 97.4%from Resunet; on the excretory phase images, 98.1% from ULBNet and 97.4% from Resunet. The proposed network architecture performs better than Resunet on generalizing to multiple phase images.
更多查看译文
关键词
Kidney Segmentation, Multiple phase CT images, Network Retraining Strategy, Resunet, LBC
AI 理解论文
溯源树
样例
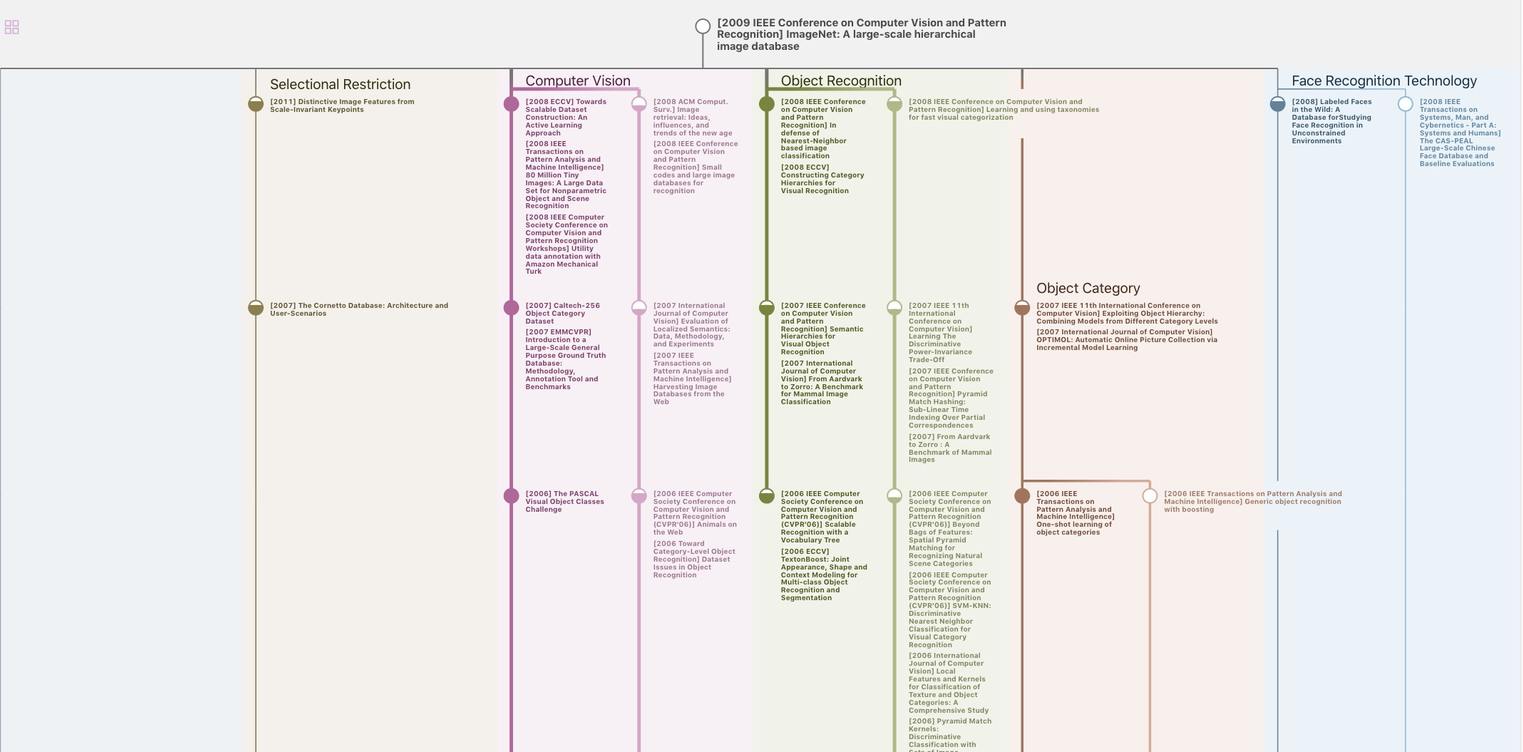
生成溯源树,研究论文发展脉络
Chat Paper
正在生成论文摘要