Dynamic Defense Approach for Adversarial Robustness in Deep Neural Networks via Stochastic Ensemble Smoothed Model
arxiv(2021)
摘要
Deep neural networks have been shown to suffer from critical vulnerabilities under adversarial attacks. This phenomenon stimulated the creation of different attack and defense strategies similar to those adopted in cyberspace security. The dependence of such strategies on attack and defense mechanisms makes the associated algorithms on both sides appear as closely reciprocating processes. The defense strategies are particularly passive in these processes, and enhancing initiative of such strategies can be an effective way to get out of this arms race. Inspired by the dynamic defense approach in cyberspace, this paper builds upon stochastic ensemble smoothing based on defense method of random smoothing and model ensemble. Proposed method employs network architecture and smoothing parameters as ensemble attributes, and dynamically change attribute-based ensemble model before every inference prediction request. The proposed method handles the extreme transferability and vulnerability of ensemble models under white-box attacks. Experimental comparison of ASR-vs-distortion curves with different attack scenarios shows that even the attacker with the highest attack capability cannot easily exceed the attack success rate associated with the ensemble smoothed model, especially under untargeted attacks.
更多查看译文
关键词
adversarial robustness,dynamic defense approach,deep neural,neural
networks
AI 理解论文
溯源树
样例
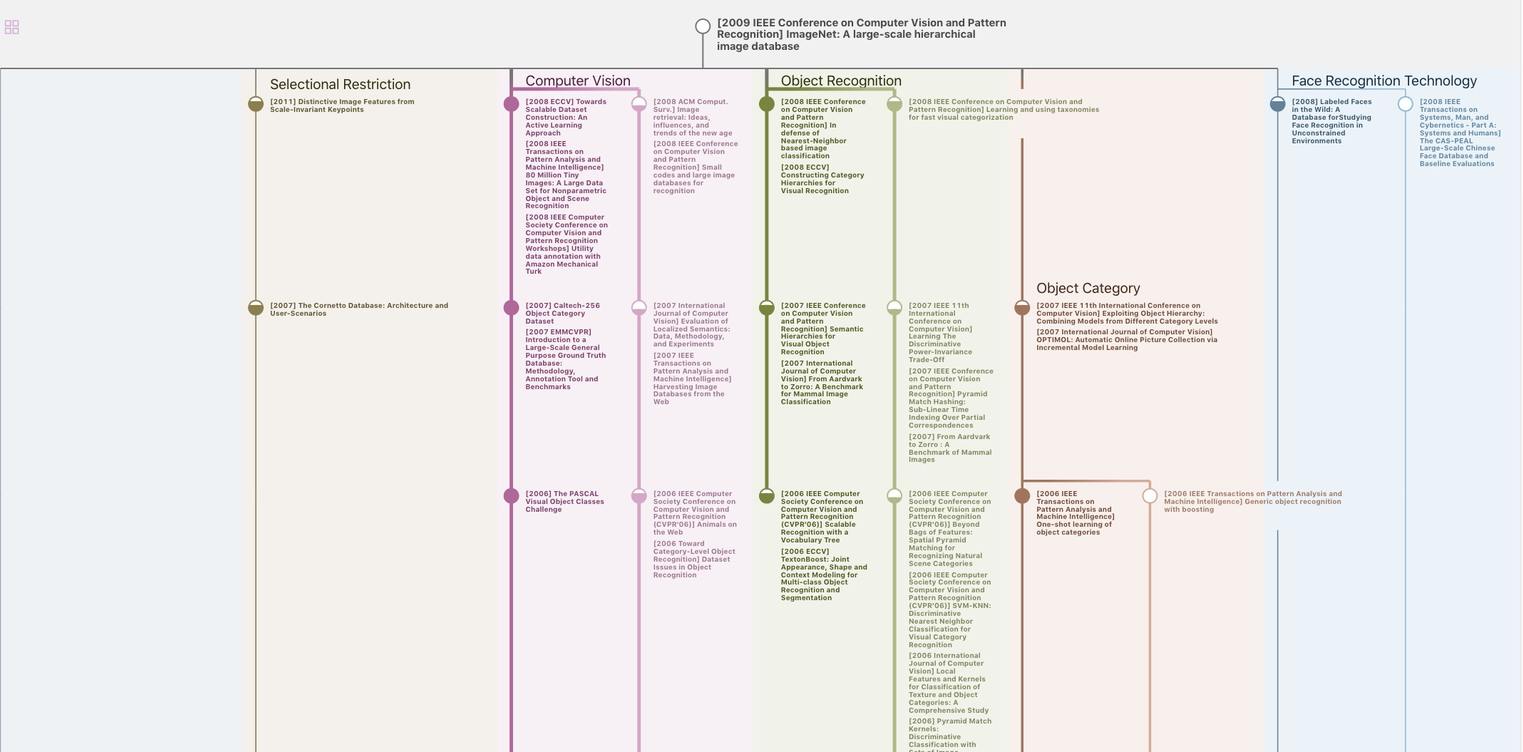
生成溯源树,研究论文发展脉络
Chat Paper
正在生成论文摘要