Homotopy Sampling, with an Application to Particle Filters
arxiv(2021)
摘要
We propose a homotopy sampling procedure, loosely based on importance sampling. Starting from a known probability distribution, the homotopy procedure generates the unknown normalization of a target distribution. In the context of stationary distributions that are associated with physical systems the method is an alternative way to estimate an unknown microcanonical ensemble. The process is iterative and also generates samples from the target distribution. In practice, the homotopy procedure does not circumvent using sample averages in the estimation of the normalization constant. The error in the procedure depends on the errors incurred in sample averaging and the number of stages used in the computational implementation of the process. However, we show that it is possible to exchange the number of homotopy stages and the total number of samples needed at each stage in order to enhance the computational efficiency of the implemented algorithm. Estimates of the error as a function of stages and sample averages are derived. These could guide computational efficiency decisions on how the calculation would be mapped to a given computer architecture. Consideration is given to how the procedure can be adapted to Bayesian estimation problems, both stationary and non-stationary. Emphasis is placed on the non-stationary problems, and in particular, on a sequential estimation technique known as particle filtering. It is shown that a modification of the particle filter framework to include the homotopy process can improve the computational robustness of particle filters. The homotopy process can ameliorate particle filter collapse, a common challenge to using particle filters when the sample dimension is small compared with the state space dimensions.
更多查看译文
关键词
sampling
AI 理解论文
溯源树
样例
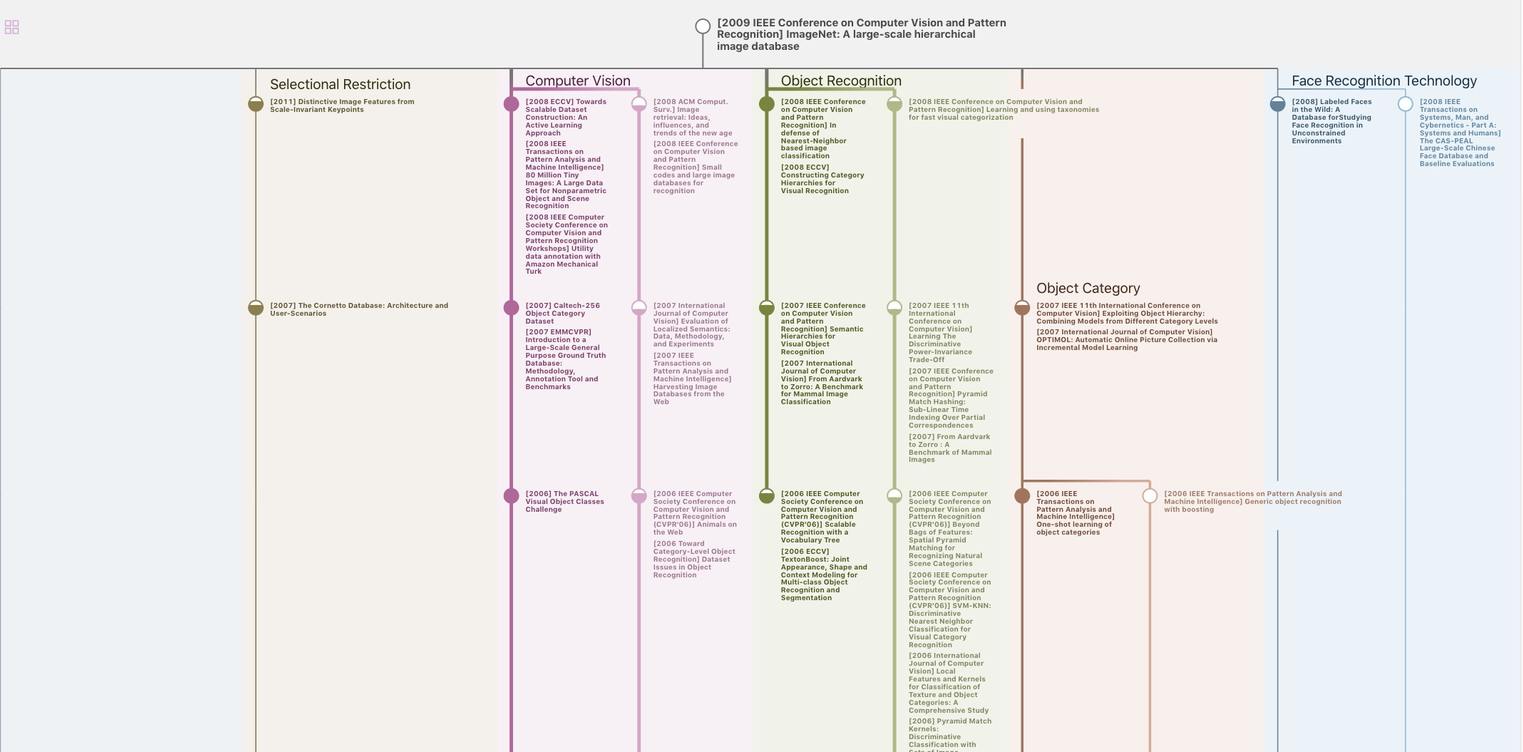
生成溯源树,研究论文发展脉络
Chat Paper
正在生成论文摘要