Detection of COVID-19 in chest X-ray images using transfer learning with deep convolutional neural network
Symposium on Applied Computing(2021)
摘要
ABSTRACTOver the years, Computer-Aided Diagnosis (CAD) systems have been proving their effectiveness in classifying many pathologies. With the advent of the COVID-19 pandemic, new systems were developed quickly. The chest radiography is one of the least expensive among the imaging exams that assist in the detection of COVID-19. Despite not having high sensitivity for pattern detection compared to other tests - such as ground-glass opacities in computed tomography - this test helps screen infected patients. Therefore, in this work, we propose a methodology for detecting COVID-19 in chest radiography considering three possible scenarios: the healthy, presence of COVID-19, and presence of other pathologies. We developed the methodology by evaluating transfer learning techniques in five well know pre-trained Convolutional Neural Networks (CNNs) architectures. For training CNNs, we used 1,932 healthy images, 3,651 of other pathologies, and 1,436 images related to the presence of COVID-19. We obtained an accuracy of 94.36% in the scenario COVID-19 vs. healthy, 99.80% for COVID-19 vs. others pathologies, and 95.01% differentiating in three classes. The results are considered promising when compared to state of the art since the database used in this work has the largest number of examples for the class COVID-19.
更多查看译文
AI 理解论文
溯源树
样例
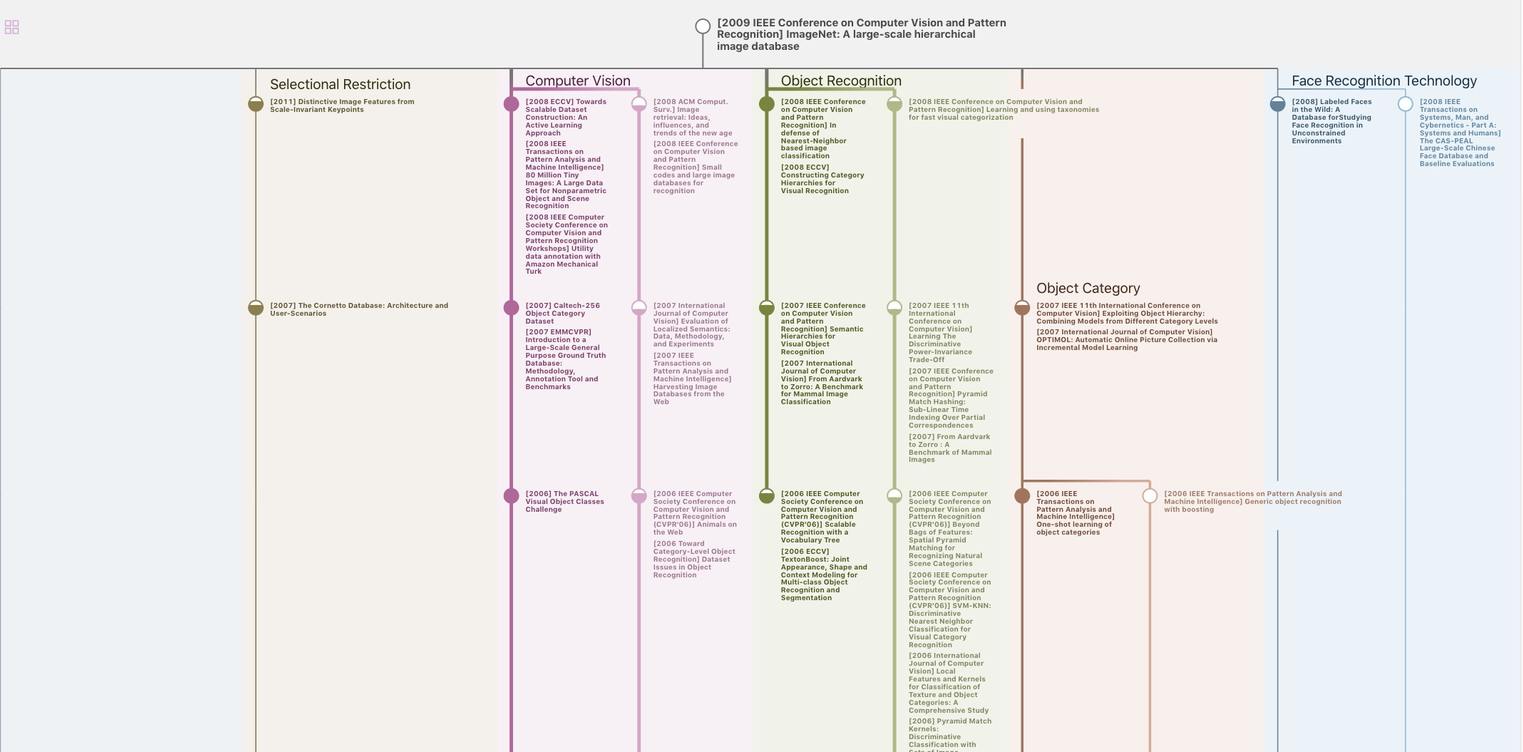
生成溯源树,研究论文发展脉络
Chat Paper
正在生成论文摘要