On the Limit of English Conversational Speech Recognition.
Interspeech(2021)
摘要
In our previous work we demonstrated that a single headed attention encoder-decoder model is able to reach state-of-the-art results in conversational speech recognition. In this paper, we further improve the results for both Switchboard 300 and 2000. Through use of an improved optimizer, speaker vector embeddings, and alternative speech representations we reduce the recognition errors of our LSTM system on Switchboard-300 by 4% relative. Compensation of the decoder model with the probability ratio approach allows more efficient integration of an external language model, and we report 5.9% and 11.5% WER on the SWB and CHM parts of Hub5'00 with very simple LSTM models. Our study also considers the recently proposed conformer, and more advanced self-attention based language models. Overall, the conformer shows similar performance to the LSTM; nevertheless, their combination and decoding with an improved LM reaches a new record on Switchboard-300, 5.0% and 10.0% WER on SWB and CHM. Our findings are also confirmed on Switchboard-2000, and a new state of the art is reported, practically reaching the limit of the benchmark.
更多查看译文
关键词
encoder-decoder,attention,speech recognition,AdamW,Switchboard,i-vector
AI 理解论文
溯源树
样例
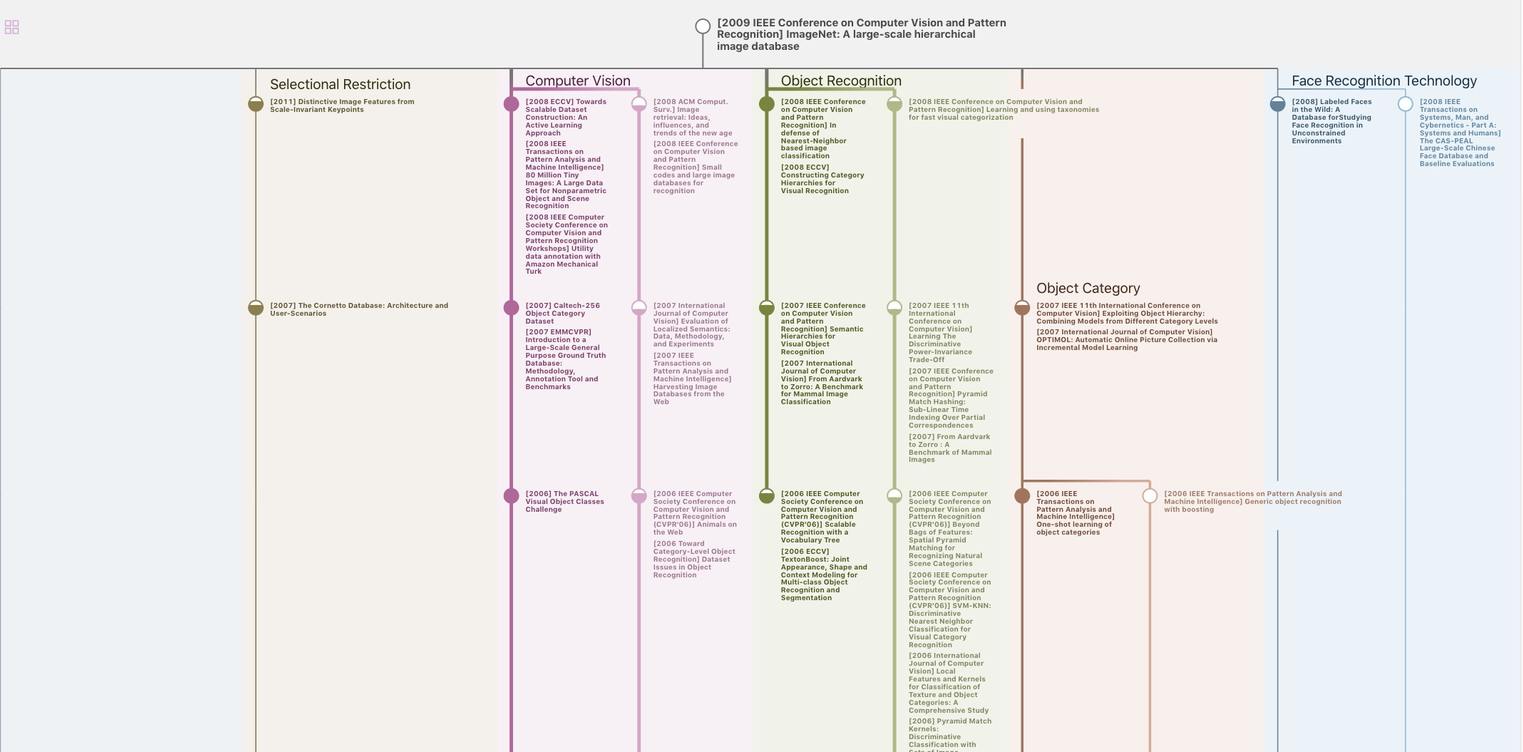
生成溯源树,研究论文发展脉络
Chat Paper
正在生成论文摘要