Online Product Feature Recommendations with Interpretable Machine Learning
arxiv(2021)
摘要
Product feature recommendations are critical for online customers to purchase the right products based on the right features. For a customer, selecting the product that has the best trade-off between price and functionality is a time-consuming step in an online shopping experience, and customers can be overwhelmed by the available choices. However, determining the set of product features that most differentiate a particular product is still an open question in online recommender systems. In this paper, we focus on using interpretable machine learning methods to tackle this problem. First, we identify this unique product feature recommendation problem from a business perspective on a major US e-commerce site. Second, we formulate the problem into a price-driven supervised learning problem to discover the product features that could best explain the price of a product in a given product category. We build machine learning models with a model-agnostic method Shapley Values to understand the importance of each feature, rank and recommend the most essential features. Third, we leverage human experts to evaluate its relevancy. The results show that our method is superior to a strong baseline method based on customer behavior and significantly boosts the coverage by 45%. Finally, our proposed method shows comparable conversion rate against the baseline in online A/B tests.
更多查看译文
关键词
interpretable machine
learning,interpretable machine,recommendations,machine
learning,product
AI 理解论文
溯源树
样例
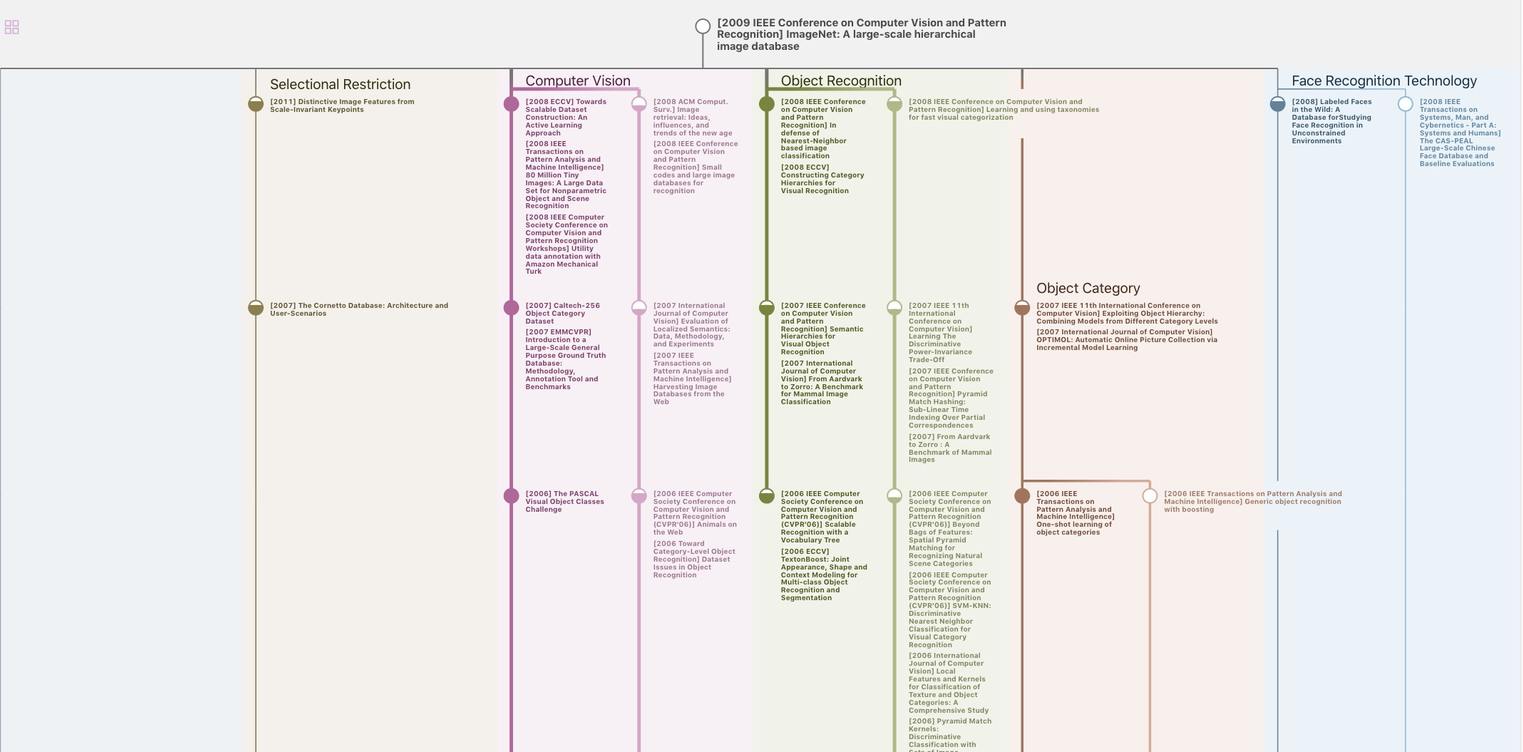
生成溯源树,研究论文发展脉络
Chat Paper
正在生成论文摘要