ResVGAE: Going Deeper with Residual Modules for Link Prediction
arxiv(2022)
摘要
Graph autoencoders are efficient at embedding graph-based data sets. Most graph autoencoder architectures have shallow depths which limits their ability to capture meaningful relations between nodes separated by multi-hops. In this paper, we propose Residual Variational Graph Autoencoder, ResVGAE, a deep variational graph autoencoder model with multiple residual modules. We show that our multiple residual modules, a convolutional layer with residual connection, improve the average precision of the graph autoencoders. Experimental results suggest that our proposed model with residual modules outperforms the models without residual modules and achieves similar results when compared with other state-of-the-art methods.
更多查看译文
关键词
link prediction,residual modules,deeper
AI 理解论文
溯源树
样例
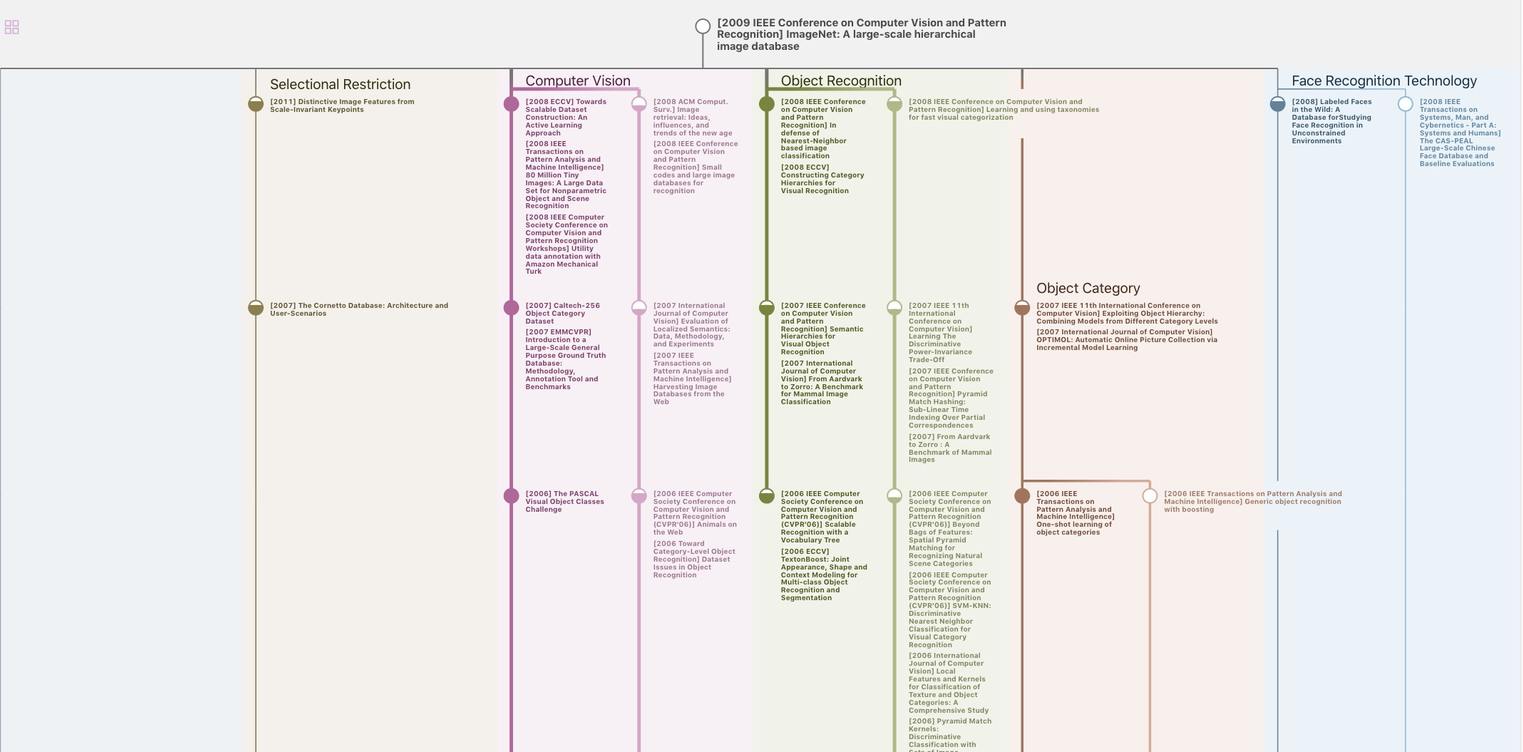
生成溯源树,研究论文发展脉络
Chat Paper
正在生成论文摘要