Learning Robust Variational Information Bottleneck with Reference
arxiv(2021)
摘要
We propose a new approach to train a variational information bottleneck (VIB) that improves its robustness to adversarial perturbations. Unlike the traditional methods where the hard labels are usually used for the classification task, we refine the categorical class information in the training phase with soft labels which are obtained from a pre-trained reference neural network and can reflect the likelihood of the original class labels. We also relax the Gaussian posterior assumption in the VIB implementation by using the mutual information neural estimation. Extensive experiments have been performed with the MNIST and CIFAR-10 datasets, and the results show that our proposed approach significantly outperforms the benchmarked models.
更多查看译文
关键词
robust variational information bottleneck
AI 理解论文
溯源树
样例
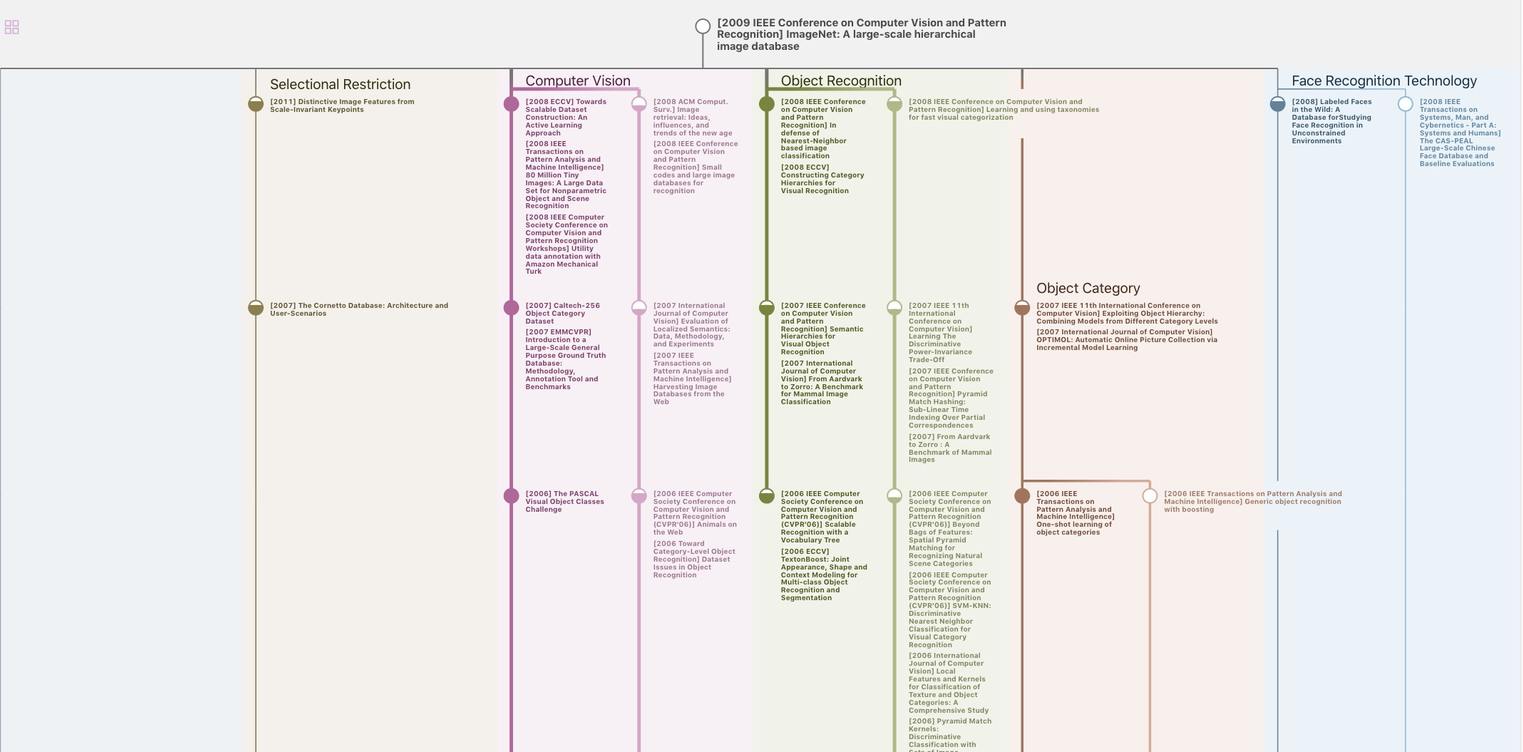
生成溯源树,研究论文发展脉络
Chat Paper
正在生成论文摘要