Ten Seconds Of My Nights: Exploring Methods To Measure Brightness, Loudness And Attendance And Their Associations With Alcohol Use From Video Clips
PLOS ONE(2021)
摘要
Introduction Most evidence on associations between alcohol use behaviors and the characteristics of its social and physical context is based on self-reports from study participants and, thus, only account for their subjective impressions of the situation. This study explores the feasibility of obtaining alternative measures of loudness, brightness, and attendance (number of people) using 10-second video clips of real-life drinking occasions rated by human annotators and computer algorithms, and explores the associations of these measures with participants' choice to drink alcohol or not.Methods Using a custom-built smartphone application, 215 16-25-year-olds documented characteristics of 2,380 weekend night drinking events using questionnaires and videos. Ratings of loudness, brightness, and attendance were obtained from three sources, namely in-situ participants' ratings, video-based annotator ratings, and video-based computer algorithm ratings. Bivariate statistics explored differences in ratings across sources. Multilevel logistic regressions assessed the associations of contextual characteristics with alcohol use. Finally, model fit indices and cross-validation were used to assess the ability of each set of contextual measures to predict participants' alcohol use.Results Raw ratings of brightness, loudness and attendance differed slightly across sources, but were all correlated (r = .21 to .82, all p < .001). Participants rated bars/pubs as being louder (Cohen's d = 0.50 [95%-CI: 0.07-0.92]), and annotators rated private places as darker (d = 1.21 [95%-CI: 0.99-1.43]) when alcohol was consumed than when alcohol was not consumed. Multilevel logistic regressions showed that drinking in private places was more likely in louder (ORparticipants = 1.74 [CI: 1.31-2.32]; ORannotators = 3.22 [CI: 2.06-5.03]; ORalgorithm = 2.62 [CI: 1.83-3.76]), more attended (ORparticipants = 1.10 [CI: 1.03-1.18]; ORalgorithm = 1.19 [CI: 1.07-1.32]) and darker (OR = 0.64 [CI: 0.44-0.94]) situations. In commercial venues, drinking was more likely in darker (ORparticipants = 0.67 [CI: 0.47-0.94]; ORannotators = 0.53 [CI: 0.33-0.85]; ORalgorithm = 0.58 [CI: 0.37-0.88]) and louder (ORparticipants = 1.40 [CI: 1.02-1.92]; ORalgorithm = 2.45 [CI: 1.25-4.80]) places. Higher inference accuracies were found for the models based on the annotators' ratings (80% to 84%) and the algorithms' ratings (76% to 86%) than on the participants' ratings (69% to 71%).Conclusions Several contextual characteristics are associated with increased odds of drinking in private and commercial settings, and might serve as a basis for the development of prevention measures. Regarding assessment of contextual characteristics, annotators and algorithms might serve as appropriate substitutes of participants' in-situ impressions for correlational and regression analyses despite differences in raw ratings. Collecting contextual data by means of sensors or media files is recommended for future research.
更多查看译文
AI 理解论文
溯源树
样例
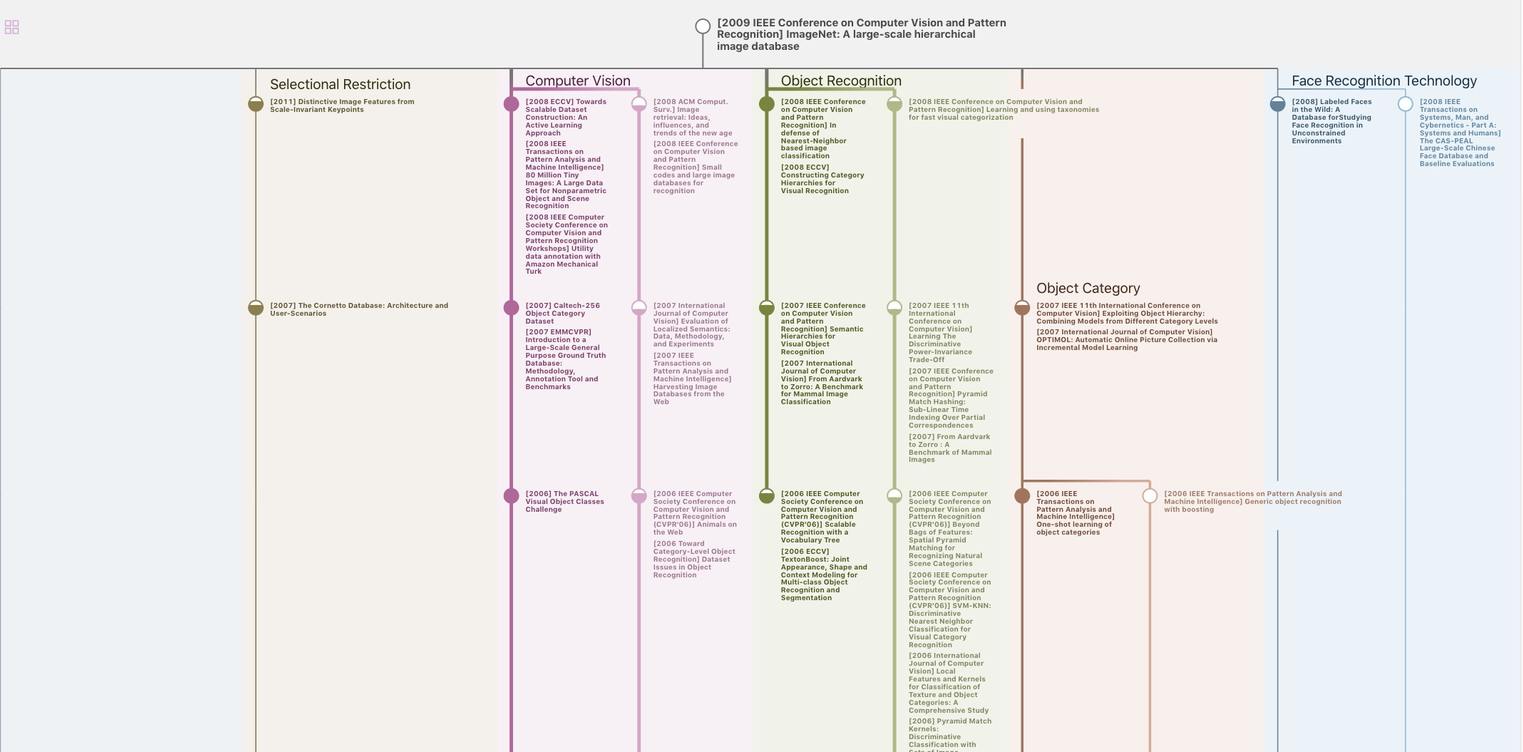
生成溯源树,研究论文发展脉络
Chat Paper
正在生成论文摘要