A Generalized Projected Bellman Error for Off-policy Value Estimation in Reinforcement Learning
arxiv(2022)
摘要
Many reinforcement learning algorithms rely on value estimation, however, the most widely used algorithms-namely temporal difference algorithms-can diverge under both off-policy sampling and nonlinear function approximation. Many algorithms have been developed for off-policy value estimation based on the linear mean squared projected Bellman error (PBE) and are sound under linear function approximation. Extending these methods to the nonlinear case has been largely unsuccessful. Recently, several methods have been introduced that approximate a different objective-the mean-squared Bellman error (BE)- which naturally facilitate nonlinear approximation. In this work, we build on these insights and introduce a new generalized PBE that extends the linear PBE to the nonlinear setting. We show how this generalized objective unifies previous work and obtain new bounds for the value error of the solutions of the generalized objective. We derive an easy-to-use, but sound, algorithm to minimize the generalized objective, and show that it is more stable across runs, is less sensitive to hyperparameters, and performs favorably across four control domains with neural network function approximation.
更多查看译文
关键词
generalized projected bellman error,reinforcement learning,estimation,value,off-policy
AI 理解论文
溯源树
样例
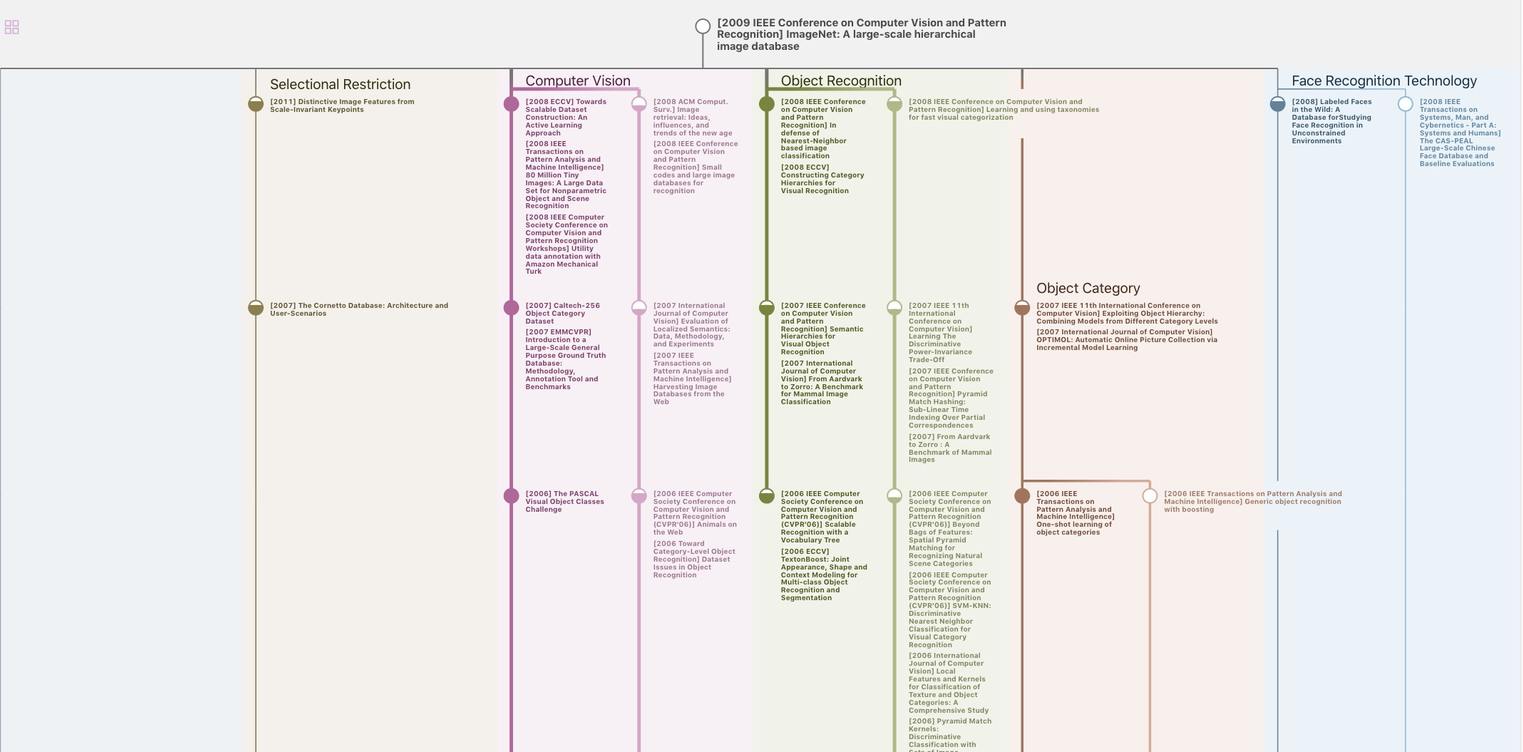
生成溯源树,研究论文发展脉络
Chat Paper
正在生成论文摘要