A Scalable and Reproducible System-on-Chip Simulation for Reinforcement Learning
arxiv(2021)
摘要
Deep Reinforcement Learning (DRL) underlies in a simulated environment and optimizes objective goals. By extending the conventional interaction scheme, this paper proffers gym-ds3, a scalable and reproducible open environment tailored for a high-fidelity Domain-Specific System-on-Chip (DSSoC) application. The simulation corroborates to schedule hierarchical jobs onto heterogeneous System-on-Chip (SoC) processors and bridges the system to reinforcement learning research. We systematically analyze the representative SoC simulator and discuss the primary challenging aspects that the system (1) continuously generates indefinite jobs at a rapid injection rate, (2) optimizes complex objectives, and (3) operates in steady-state scheduling. We provide exemplary snippets and experimentally demonstrate the run-time performances on different schedulers that successfully mimic results achieved from the standard DS3 framework and real-world embedded systems.
更多查看译文
关键词
reinforcement learning,simulation,system-on-chip
AI 理解论文
溯源树
样例
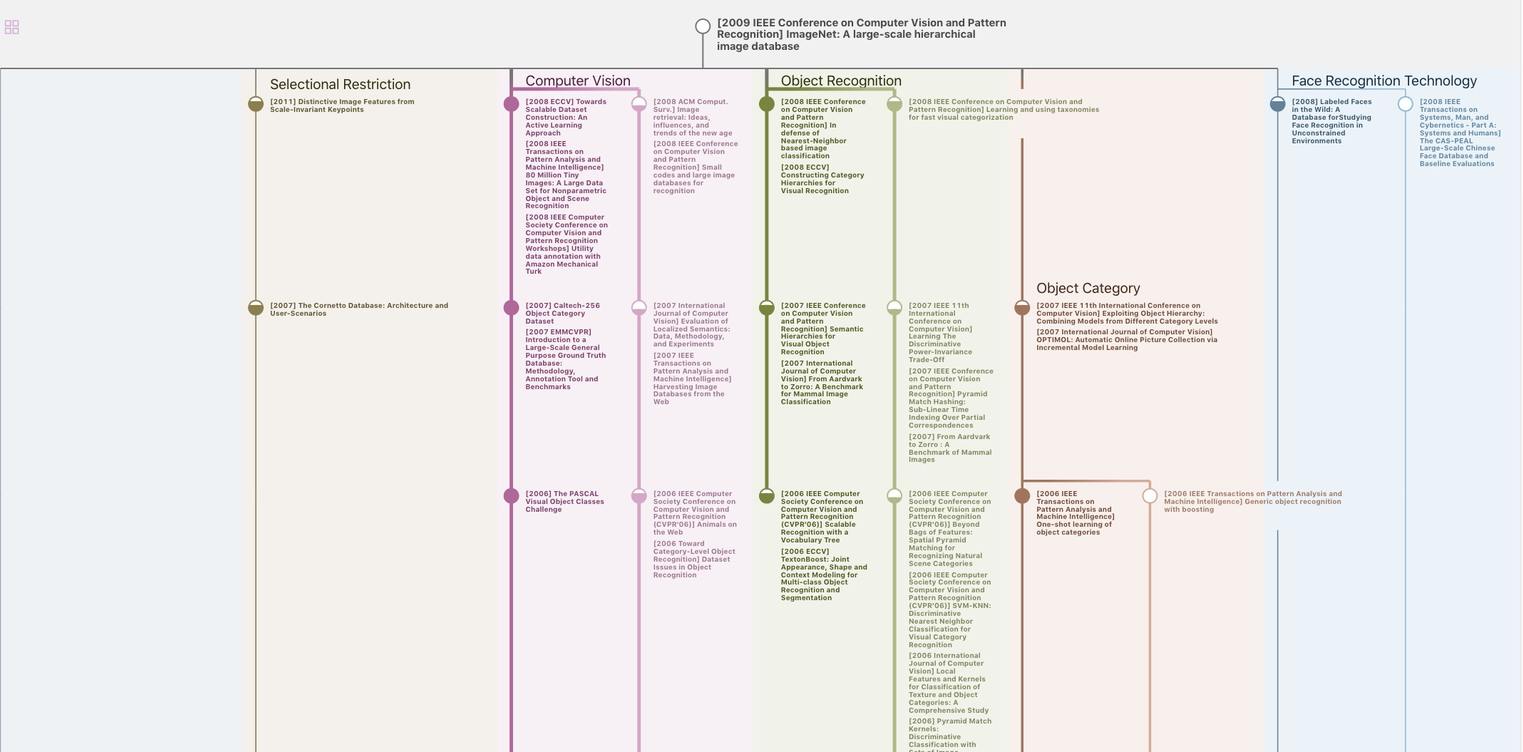
生成溯源树,研究论文发展脉络
Chat Paper
正在生成论文摘要