Finite Sample Approximations of Exact and Entropic Wasserstein Distances Between Covariance Operators and Gaussian Processes
SIAM-ASA JOURNAL ON UNCERTAINTY QUANTIFICATION(2022)
摘要
This work studies finite sample approximations of the exact and entropic regularized Wasserstein distances between centered Gaussian processes and, more generally, covariance operators of functional random processes. We first show that these distances/divergences are fully represented by reproducing kernel Hilbert space (RKHS) covariance and cross-covariance operators associated with the corresponding covariance functions. Using this representation, we show that the Sinkhorn divergence between two centered Gaussian processes can be consistently and efficiently estimated from the divergence between their corresponding normalized finite-dimensional covariance matrices or, alternatively, their sample covariance operators. Consequently, this leads to a consistent and efficient algorithm for estimating the Sinkhorn divergence from finite samples generated by the two processes. For a fixed regularization parameter, the convergence rates are dimension-independent and of the same order as those for the Hilbert-Schmidt distance. If at least one of the RKHS is finite-dimensional, we obtain a dimension-dependent sample complexity for the exact Wasserstein distance between the Gaussian processes.
更多查看译文
关键词
Wasserstein distance, entropic regularization, Gaussian processes, reproducing kernel Hilbert space, optimal transport
AI 理解论文
溯源树
样例
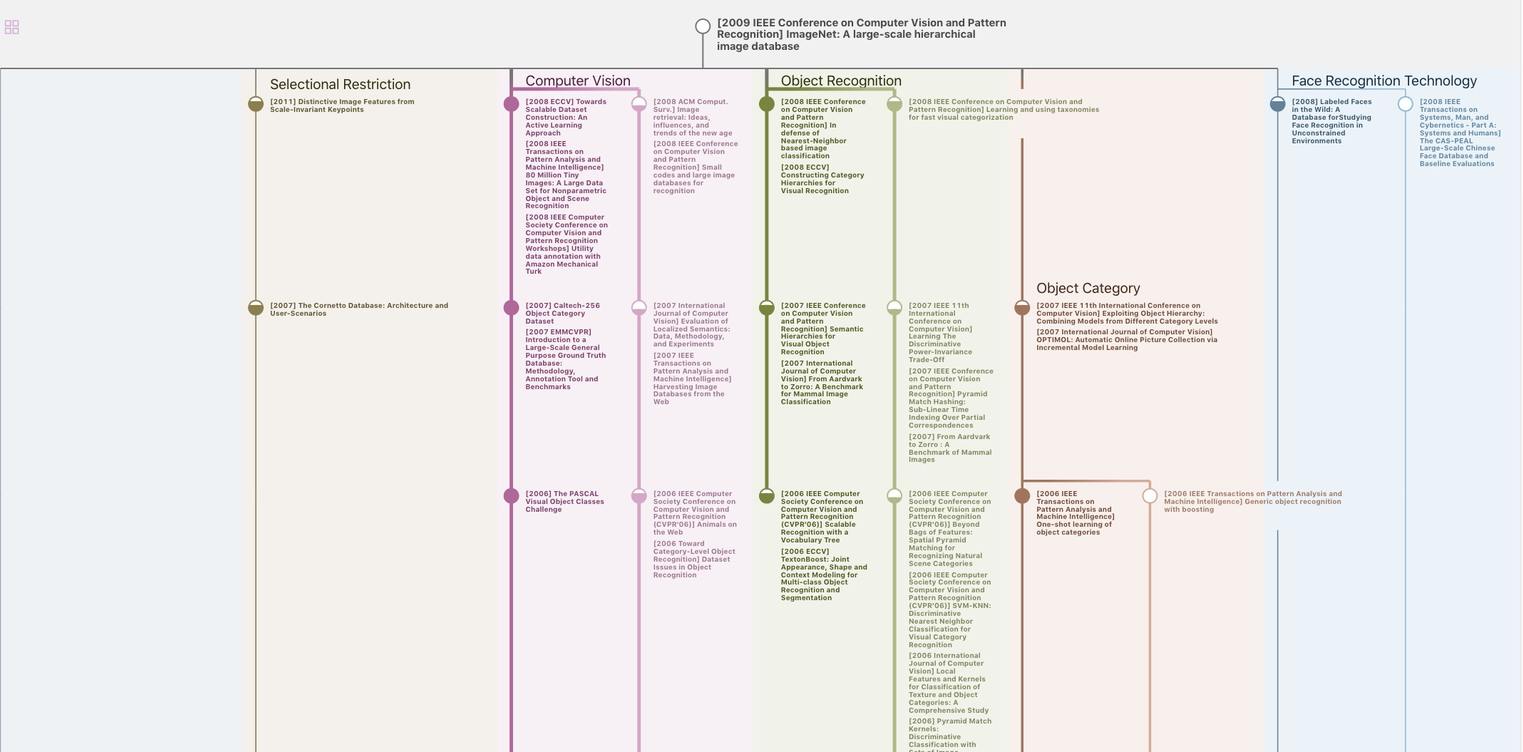
生成溯源树,研究论文发展脉络
Chat Paper
正在生成论文摘要