Model-Based Deep Autoencoder Networks for Nonlinear Hyperspectral Unmixing
IEEE GEOSCIENCE AND REMOTE SENSING LETTERS(2022)
摘要
Autoencoder (AEC) networks have recently emerged as a promising approach to perform unsupervised hyperspectral unmixing (HU) by associating the latent representations with the abundances, the decoder with the mixing model, and the encoder with its inverse. AECs are especially appealing for nonlinear HU since they lead to unsupervised and model-free algorithms. However, existing approaches fail to explore the fact that the encoder should invert the mixing process, which might reduce their robustness. In this letter, we propose a model-based AEC for nonlinear HU by considering the mixing model a nonlinear fluctuation over a linear mixture. Different from previous works, we show that this restriction naturally imposes a particular structure to both the encoder and decoder networks. This introduces prior information in the AEC without reducing the flexibility of the mixing model. Simulations with synthetic and real data indicate that the proposed strategy improves nonlinear HU.
更多查看译文
关键词
Decoding, Artificial neural networks, Data models, Training, Hyperspectral imaging, Robustness, Kernel, Autoencoder (AEC), deep neural networks (NNs), hyperspectral data, nonlinear unmixing
AI 理解论文
溯源树
样例
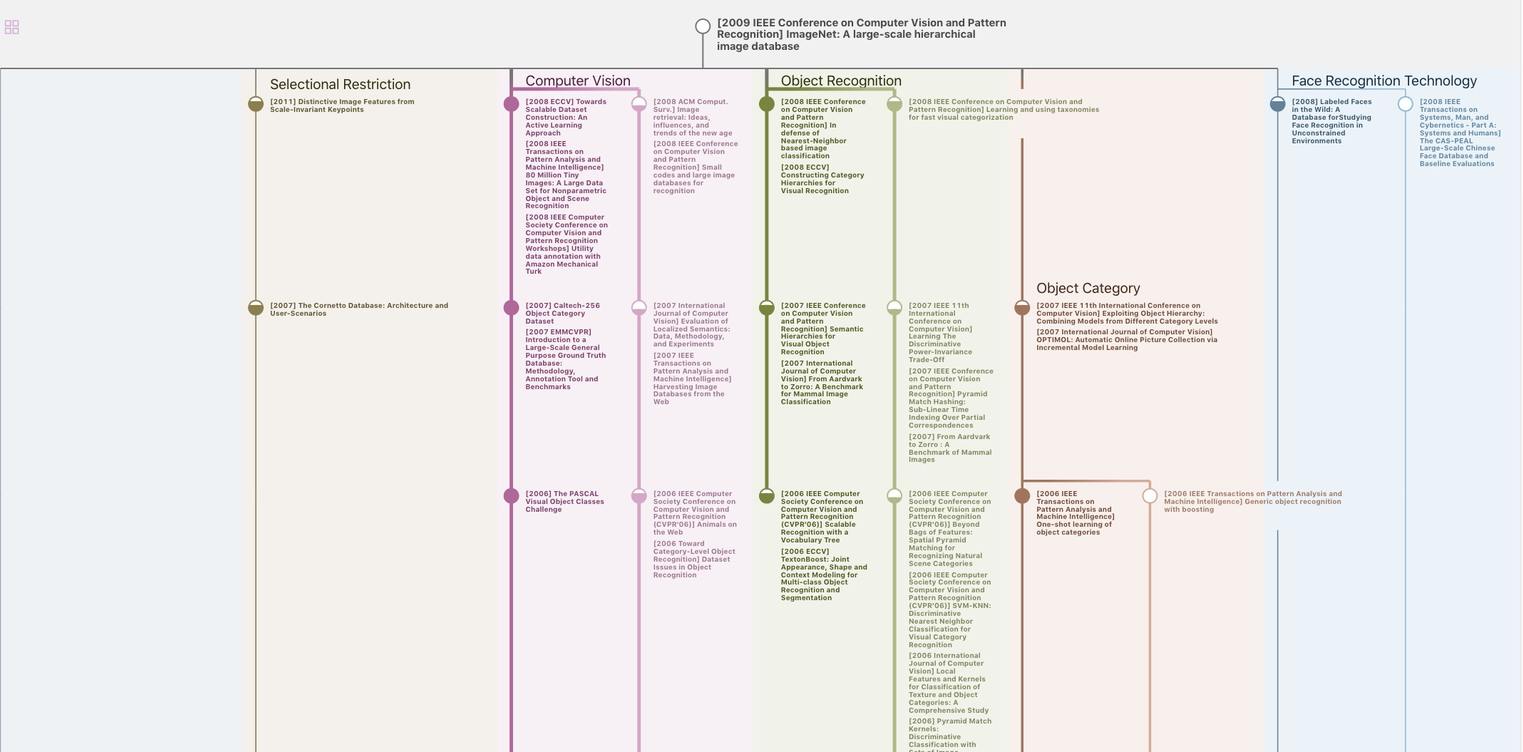
生成溯源树,研究论文发展脉络
Chat Paper
正在生成论文摘要