Nonblind Image Deblurring via Deep Learning in Complex Field
IEEE Transactions on Neural Networks and Learning Systems(2022)
摘要
Nonblind image deblurring is about recovering the latent clear image from a blurry one generated by a known blur kernel, which is an often-seen yet challenging inverse problem in imaging. Its key is how to robustly suppress noise magnification during the inversion process. Recent approaches made a breakthrough by exploiting convolutional neural network (CNN)-based denoising priors in the image domain or the gradient domain, which allows using a CNN for noise suppression. The performance of these approaches is highly dependent on the effectiveness of the denoising CNN in removing magnified noise whose distribution is unknown and varies at different iterations of the deblurring process for different images. In this article, we introduce a CNN-based image prior defined in the Gabor domain. The prior not only utilizes the optimal space-frequency resolution and strong orientation selectivity of the Gabor transform but also enables using complex-valued (CV) representations in intermediate processing for better denoising. A CV CNN is developed to exploit the benefits of the CV representations, with better generalization to handle unknown noises over the real-valued ones. Combining our Gabor-domain CV CNN-based prior with an unrolling scheme, we propose a deep-learning-based approach to nonblind image deblurring. Extensive experiments have demonstrated the superior performance of the proposed approach over the state-of-the-art ones.
更多查看译文
关键词
Complex-valued (CV) convolutional neural network (CNN),deep learning,Gabor transform,image deblurring,inverse problem
AI 理解论文
溯源树
样例
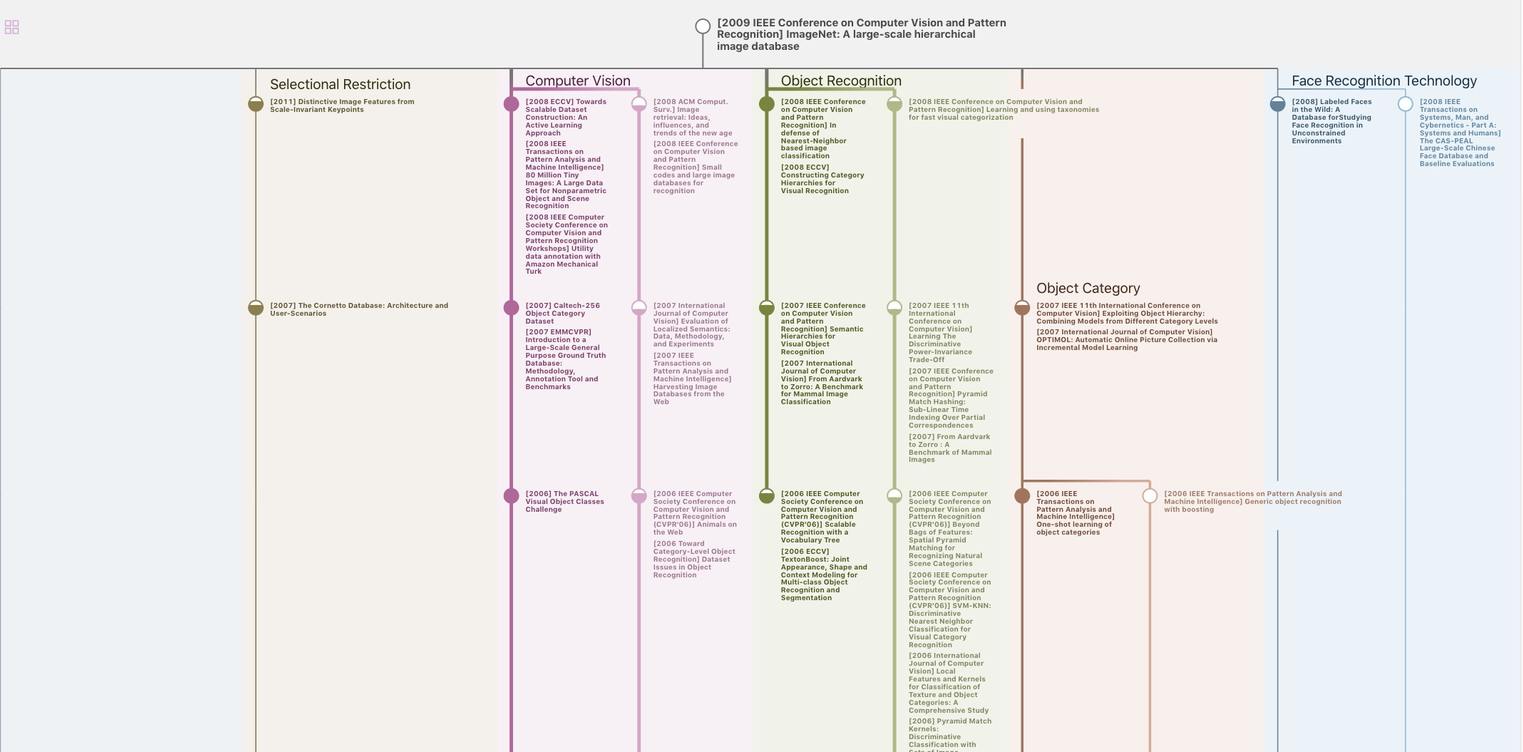
生成溯源树,研究论文发展脉络
Chat Paper
正在生成论文摘要