Deep nonparametric regression on approximate manifolds: nonasymptotic error bounds with polynomial prefactors
arxiv(2023)
摘要
We study the properties of nonparametric least squares regression using deep neural networks. We derive nonasymptotic upper bounds for the excess risk of the empirical risk minimizer of feedforward deep neural regression. Our error bounds achieve minimax optimal rate and improve over the exist-ing ones in the sense that they depend polynomially on the dimension of the predictor, instead of exponentially on dimension. We show that the neural regression estimator can circumvent the curse of dimensionality under the as-sumption that the predictor is supported on an approximate low-dimensional manifold or a set with low Minkowski dimension. We also establish the opti-mal convergence rate under the exact manifold support assumption. We inves-tigate how the prediction error of the neural regression estimator depends on the structure of neural networks and propose a notion of network relative ef-ficiency between two types of neural networks, which provides a quantitative measure for evaluating the relative merits of different network structures. To establish these results, we derive a novel approximation error bound for the Holder smooth functions using ReLU activated neural networks, which may be of independent interest. Our results are derived under weaker assumptions on the data distribution and the neural network structure than those in the existing literature.
更多查看译文
关键词
Approximation error,curse of dimensionality,deep neural network,low-dimensional manifolds,network relative efficiency,nonasymptotic error bound
AI 理解论文
溯源树
样例
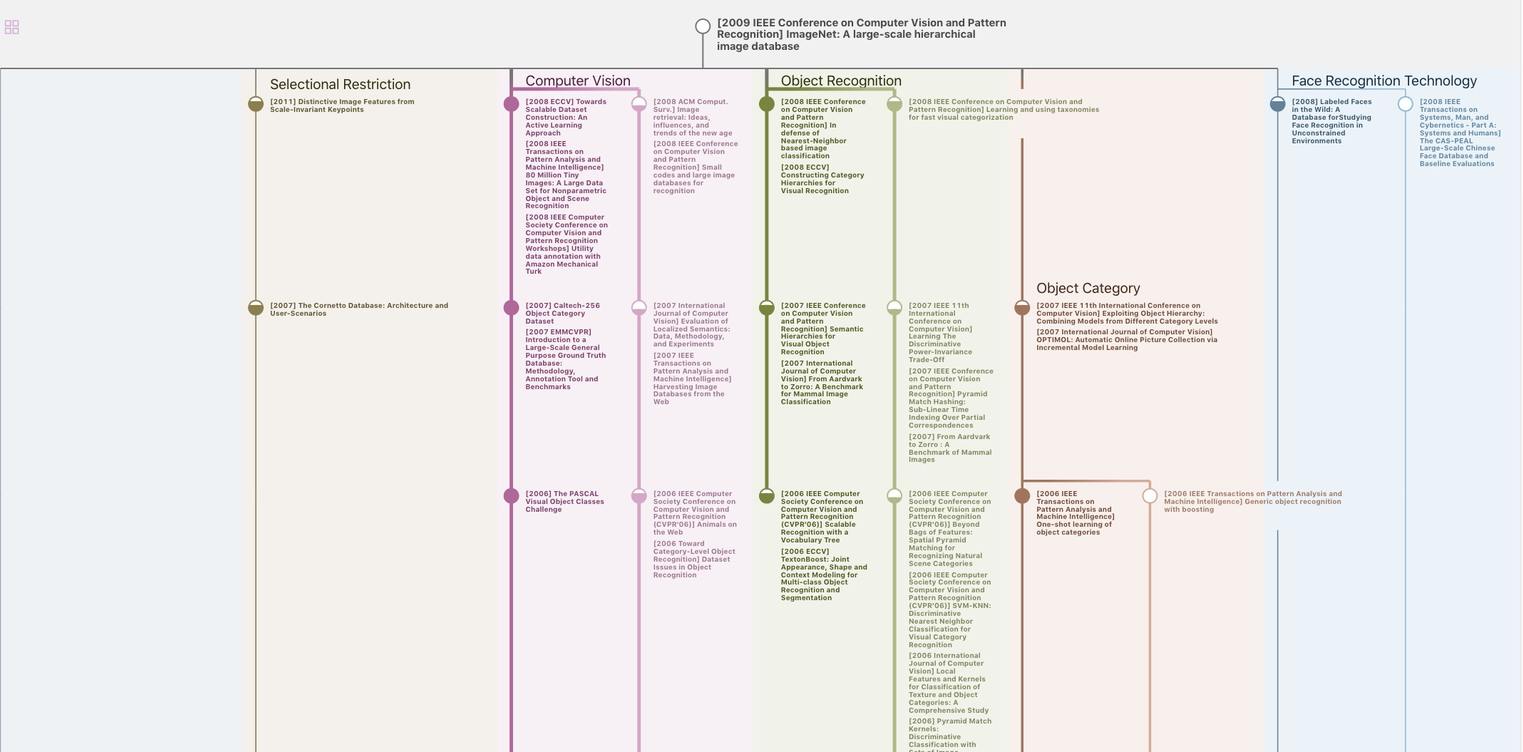
生成溯源树,研究论文发展脉络
Chat Paper
正在生成论文摘要