BlockGNN: Towards Efficient GNN Acceleration Using Block-Circulant Weight Matrices
2021 58TH ACM/IEEE DESIGN AUTOMATION CONFERENCE (DAC)(2021)
摘要
In recent years, Graph Neural Networks (GNNs) appear to be state-of-the-art algorithms for analyzing non-euclidean graph data. By applying deep-learning to extract high-level representations from graph structures, GNNs achieve extraordinary accuracy and great generalization ability in various tasks. However, with the ever-increasing graph sizes, more and more complicated GNN layers, and higher feature dimensions, the computational complexity of GNNs grows exponentially. How to inference GNNs in real time has become a challenging problem, especially for some resource-limited edge-computing platforms. To tackle this challenge, we propose BlockGNN, a software-hardware co-design approach to realize efficient GNN acceleration. At the algorithm level, we propose to leverage block-circulant weight matrices to greatly reduce the complexity of various GNN models. At the hardware design level, we propose a pipelined CirCore architecture, which supports efficient block-circulant matrices computation. Basing on CirCore, we present a novel BlockGNN accelerator to compute various GNNs with low latency. Moreover, to determine the optimal configurations for diverse deployed tasks, we also introduce a performance and resource model that helps choose the optimal hardware parameters automatically. Comprehensive experiments on the ZC706 FPGA platform demonstrate that on various GNN tasks, BlockGNN achieves up to 8.3x speedup compared to the baseline HyGCN architecture and 111.9x energy reduction compared to the Intel Xeon CPU platform.
更多查看译文
关键词
Graph Neural Network, Computer Architecture, Compression, Acceleration, FPGA
AI 理解论文
溯源树
样例
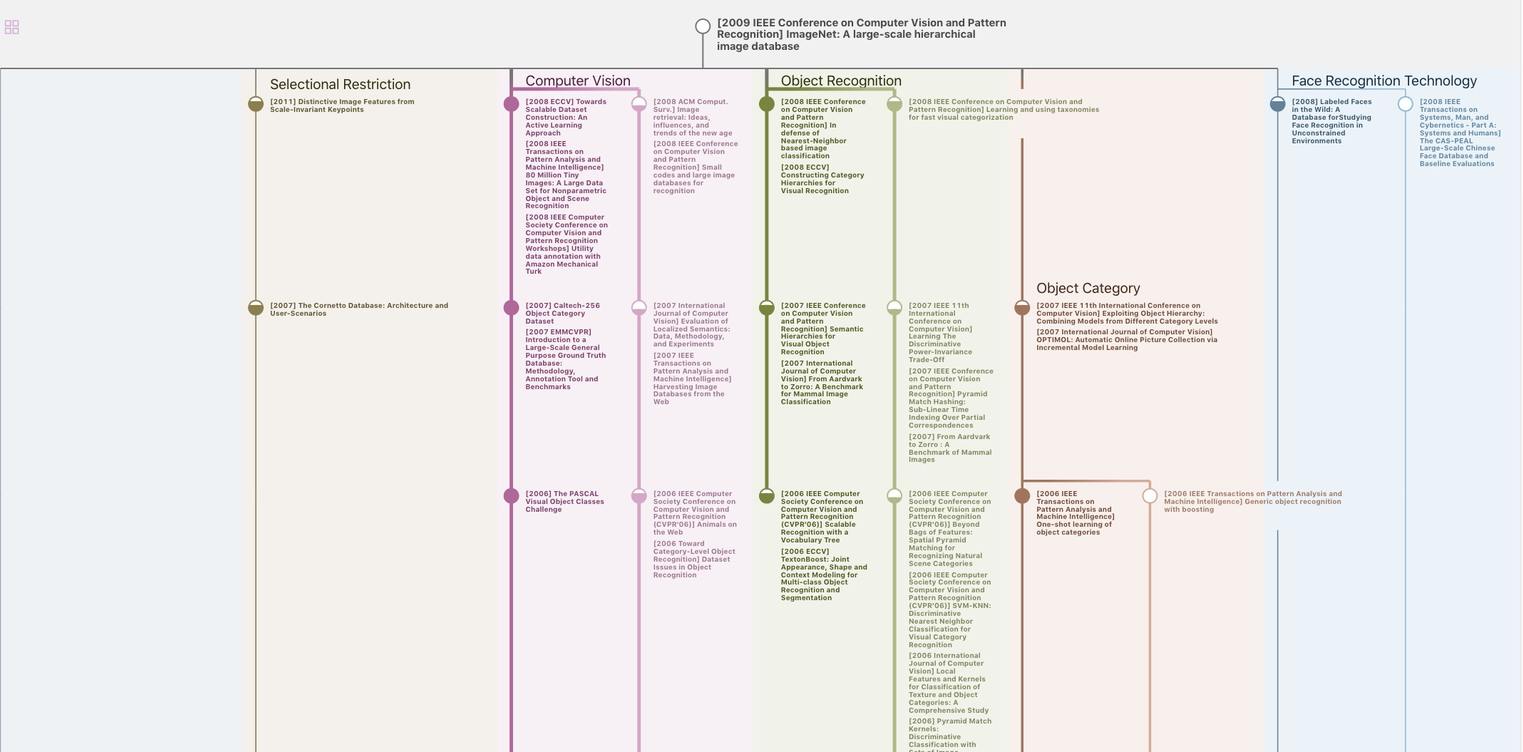
生成溯源树,研究论文发展脉络
Chat Paper
正在生成论文摘要