GuILD: Guided Incremental Local Densification for Accelerated Sampling-based Motion Planning.
arxiv(2023)
摘要
Sampling-based motion planners rely on incremental densification to discover progressively shorter paths. After computing feasible path xi between start x(s) and goal x(t), the Informed Set (IS) prunes the configuration space X by conservatively eliminating points that cannot yield shorter paths. Densification via sampling from this Informed Set retains asymptotic optimality of sampling from the entire configuration space. For path length c(xi) and Euclidean heuristic h, IS = {x vertical bar x is an element of X, h(x(s), x) + h(x, x(t)) <= c(xi)}. Relying on the heuristic can render the IS especially conservative in high dimensions or complex environments. Furthermore, the IS only shrinks when shorter paths are discovered. Thus, the computational effort from each iteration of densification and planning is wasted if it fails to yield a shorter path, despite improving the cost-to-come for vertices in the search tree. Our key insight is that even in such a failure, shorter paths to vertices in the search tree (rather than just the goal) can immediately improve the planner's sampling strategy. Guided Incremental Local Densification (GuILD) leverages this information to sample from Local Subsets of the IS. We show that GuILD significantly outperforms uniform sampling of the Informed Set in simulated R-2, SE(2) environments and manipulation tasks in R-7.
更多查看译文
关键词
accelerated sampling-based motion planning,configuration space X,entire configuration space,guided incremental local densification,GuILD,incremental densification,informed set,planner,progressively shorter paths,sampling-based motion planners,shorter path,uniform sampling
AI 理解论文
溯源树
样例
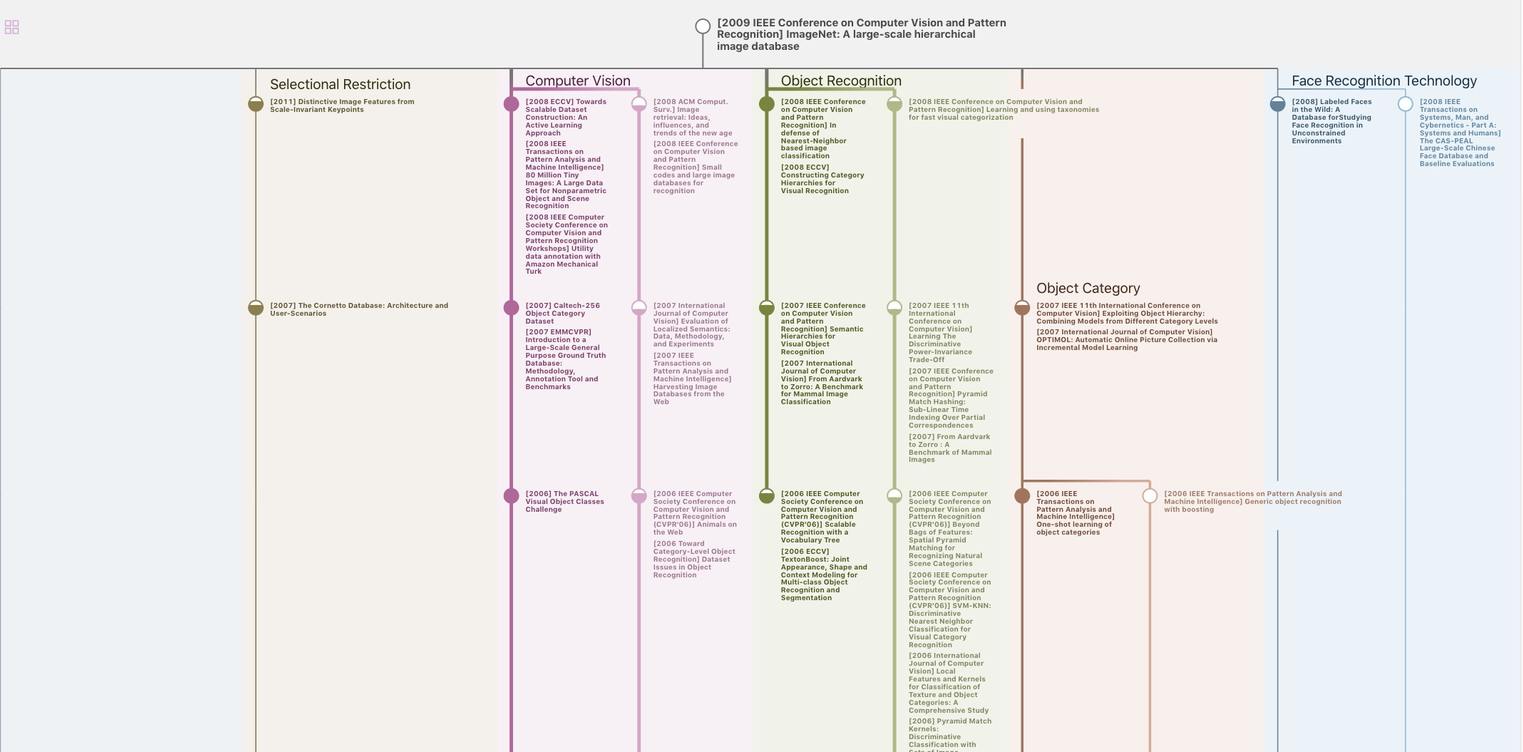
生成溯源树,研究论文发展脉络
Chat Paper
正在生成论文摘要