Deep Weakly Supervised Positioning
IEEE Robotics and Automation Letters(2021)
摘要
PoseNet can map a photo to the position where it is taken, which is appealing in robotics. However, training PoseNet requires full supervision, where ground truth positions are non-trivial to obtain. Can we train PoseNet without knowing the ground truth positions for each observation? We show that this is possible via constraint-based weak-supervision, leading to the proposed framework: DeepGPS. Particularly, using wheel-encoder-estimated distances traveled by a robot along random straight line segments as constraints between PoseNet outputs, DeepGPS can achieve a relative positioning error of less than 2%. Moreover, training DeepGPS can be done as auto-calibration with almost no human attendance, which is more attractive than its competing methods that typically require careful and expert-level manual calibration. We conduct various experiments on simulated and real datasets to demonstrate the general applicability, effectiveness, and accuracy of DeepGPS, and perform a comprehensive analysis of its robustness. Our code is available at https://ai4ce.github.io/DeepGPS/.
更多查看译文
关键词
Deep learning for visual perception,localization
AI 理解论文
溯源树
样例
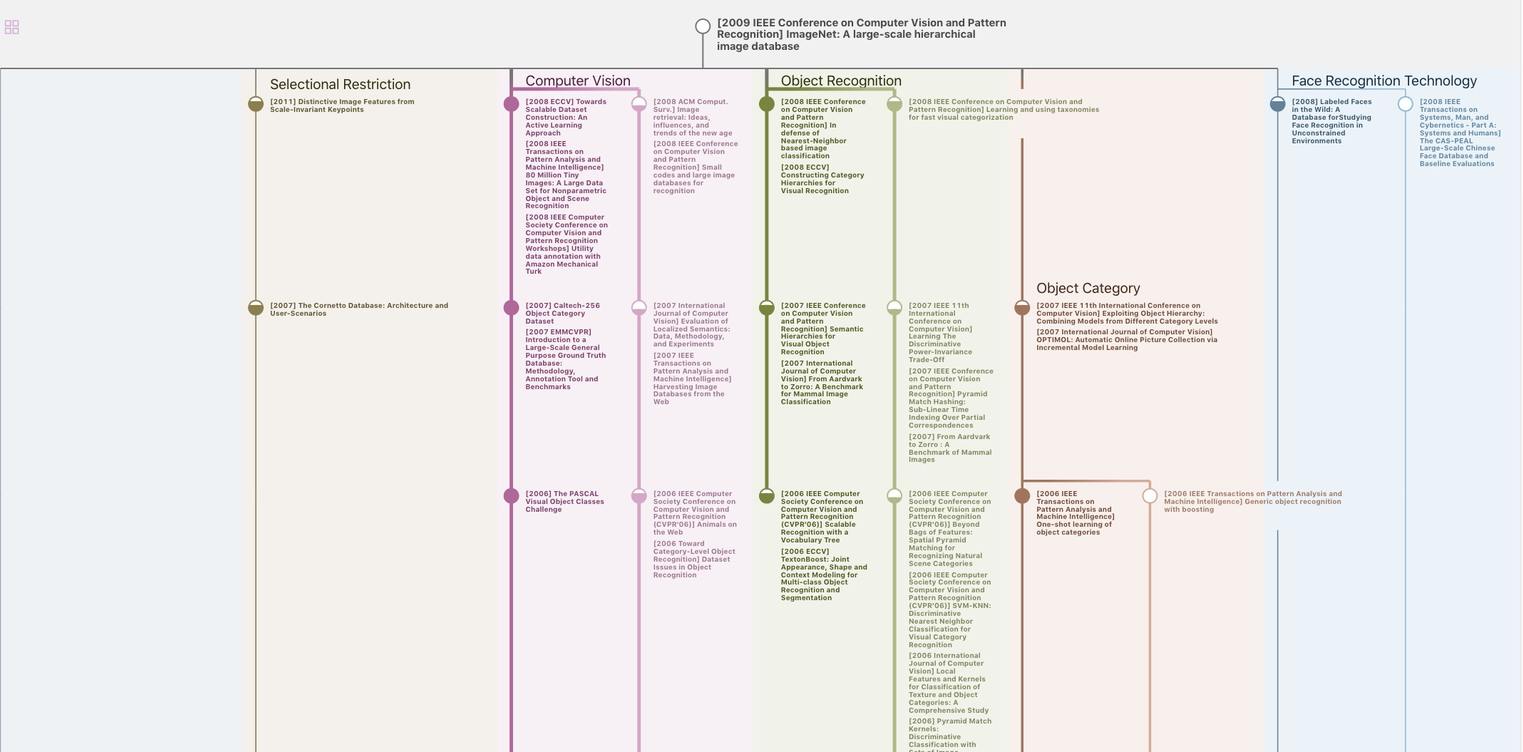
生成溯源树,研究论文发展脉络
Chat Paper
正在生成论文摘要