Selection-Expansion: A Unifying Framework for Motion-Planning and Diversity Search Algorithms
ARTIFICIAL NEURAL NETWORKS AND MACHINE LEARNING - ICANN 2021, PT IV(2021)
摘要
Reinforcement learning agents need a reward signal to learn successful policies. When this signal is sparse or the corresponding gradient is deceptive, such agents need a dedicated mechanism to efficiently explore their search space without relying on the reward. Looking for a large diversity of behaviors or using Motion Planning (MP) algorithms are two options in this context. In this paper, we build on the common roots between these two options to investigate the properties of two diversity search algorithms, the Novelty Search and the Goal Exploration Process algorithms. These algorithms look for diversity in an outcome space or behavioral space which is generally hand-designed to represent what matters for a given task. The relation to MP algorithms reveals that the smoothness, or lack of smoothness of the mapping between the policy parameter space and the outcome space plays a key role in the search efficiency. In particular, we show empirically that, if the mapping is smooth enough, i.e. if two close policies in the parameter space lead to similar outcomes, then diversity algorithms tend to inherit exploration properties of MP algorithms. By contrast, if it is not, diversity algorithms lose the properties of their MP counterparts and their performance strongly depends on heuristics like filtering mechanisms.
更多查看译文
关键词
diversity,search,selection-expansion,motion-planning
AI 理解论文
溯源树
样例
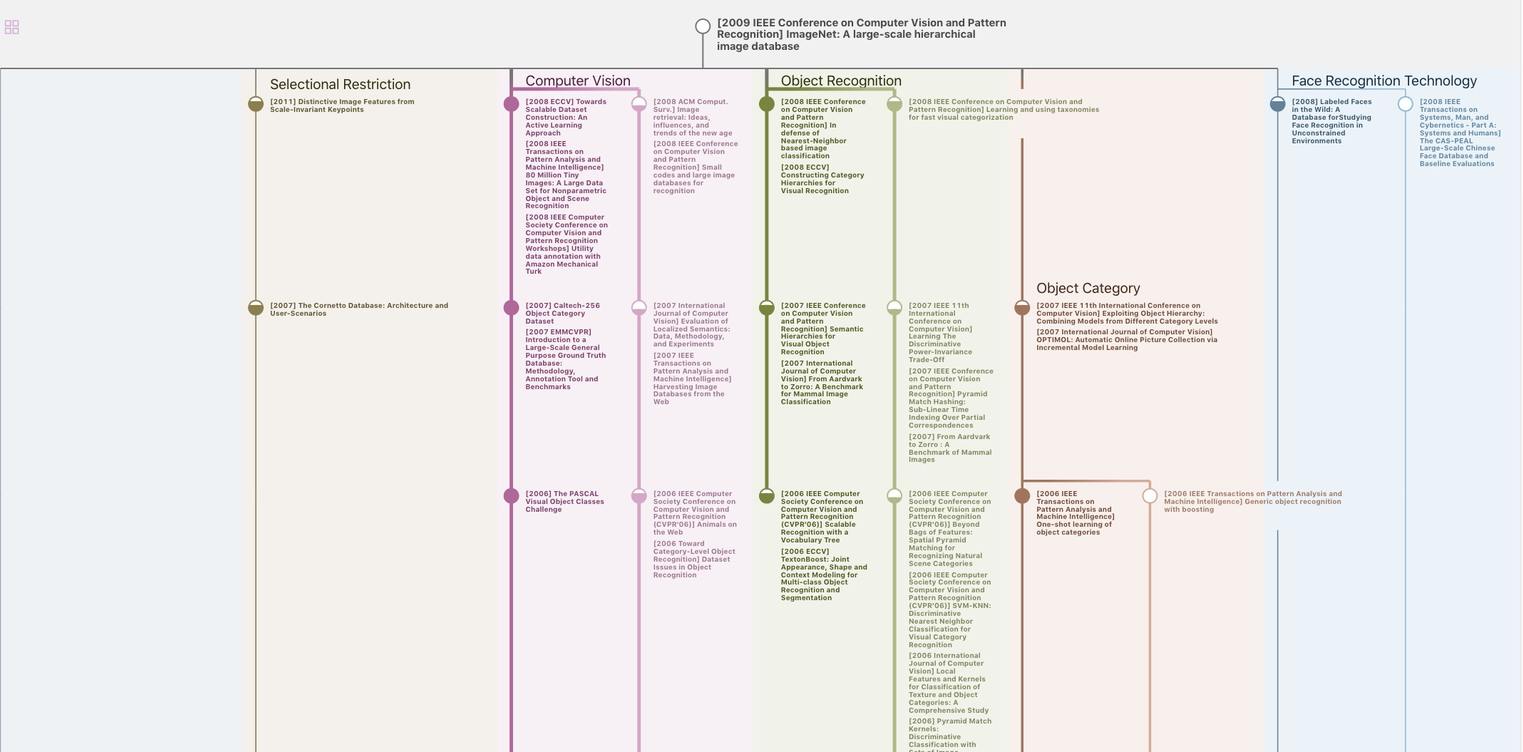
生成溯源树,研究论文发展脉络
Chat Paper
正在生成论文摘要