Operational statistical postprocessing of temperature ensemble forecasts with station‐specific predictors
Meteorological Applications(2020)
摘要
A proper account for forecast uncertainty is crucial in operational weather services and weather-related decision-making. Ensemble forecasts provide such information. However, they may be biased and tend to be under-dispersive. Therefore, ensemble forecasts need to be post-processed before using them in operational weather products. The present study post-processes the European Centre for Medium-Range Weather Forecasts (ECMWF) ensemble prediction system temperature forecasts over Europe with lead times up to 240 hr using the statistical calibration method that is currently implemented in operational workflow at the Finnish Meteorological Institute (FMI). The calibration coefficients are estimated simultaneously for all stations using a 30 day rolling training period. Station-specific characteristic are accounted for by using elevation, latitude and land-sea mask as additional predictors in the calibration. On average the calibration improved the ensemble spread over Europe, although the improvements varied between different verification months. In March, the calibration improved ensemble forecasts the most, while in January the performance depended strongly on location. A comparison between three versions with different sets of station-specific predictors in calibration showed that elevation was the most important predictor, while latitude and land-sea mask improved the forecasts mostly with shorter lead times. The calibration for the Finnish stations was also tested using three different size training domains in order to find the optimal training area. The results showed that smaller training domains had a significant effect on calibration performance only at lead times up to a few days. With longer lead times, the calibrated forecasts were better when all available stations were included.
更多查看译文
关键词
non‐, homogeneous Gaussian regression, probabilistic forecasting, station specific, statistical ensemble post‐, processing, temperature, weather forecasting
AI 理解论文
溯源树
样例
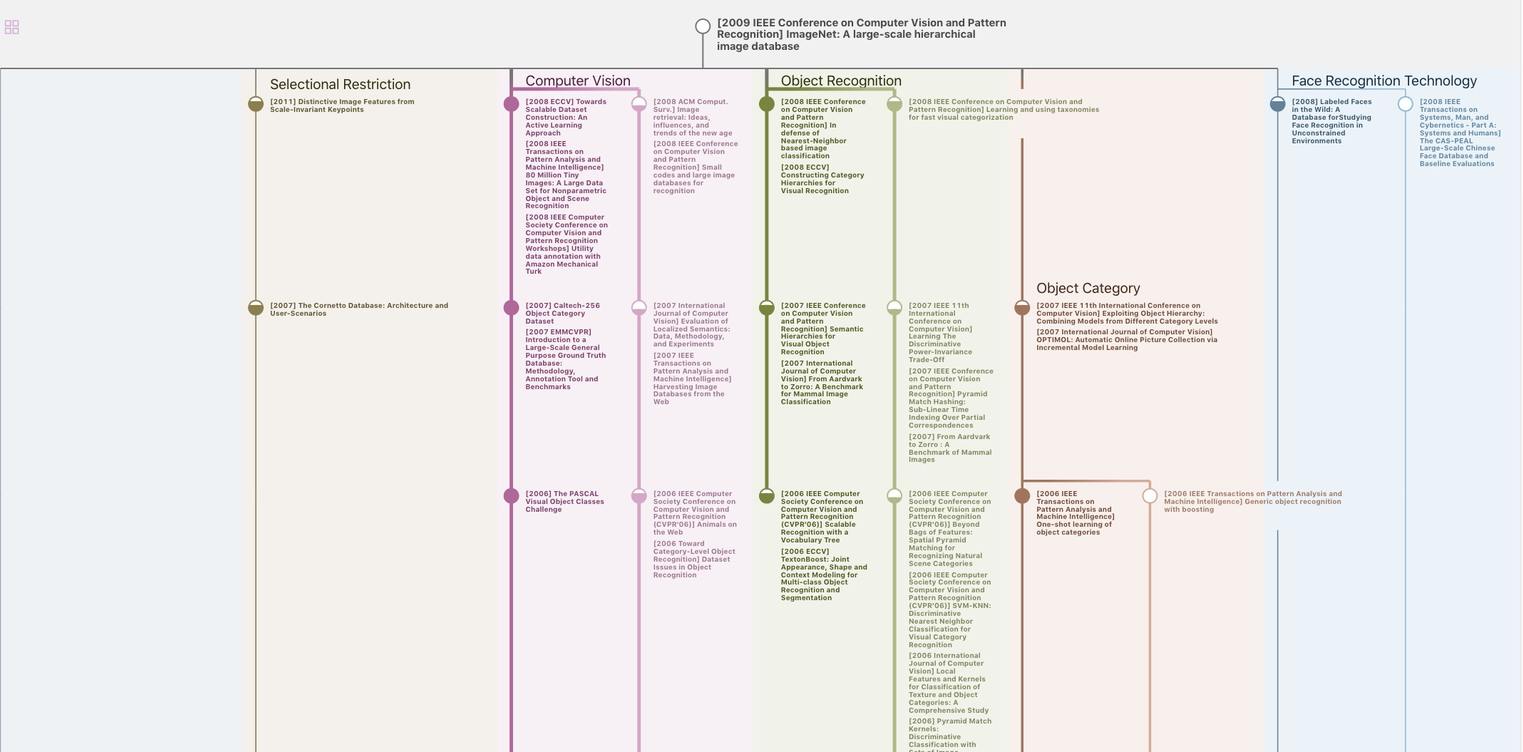
生成溯源树,研究论文发展脉络
Chat Paper
正在生成论文摘要