A latent‐factor‐driven endogenous regime‐switching non‐Gaussian model: Evidence from simulation and application
International Journal of Finance & Economics(2020)
摘要
Regime-switching models are widely used in empirical economics and finance research for their ability to identify and account for the impact of latent regimes or states on the behaviour of the interested variables. Meanwhile, empirical evidence often reveals complex non-Gaussian characteristics in the state-dependent dynamics. We generalize the latent-factor-driven endogenous regime-switching Gaussian model of Chang, et al.,Journal of Econometrics, 2017,196, 127-143 by allowing the state-dependent conditional distributions to be non-Gaussian. Our setup is more general and promises substantially broader relevance and applicability to empirical studies. We provide evidence to justify our generalization by a simulation study and a real data application. Our simulation results confirm that when the state-dependent dynamics are misspecified, the bias of model parameter estimates, the power of the likelihood ratio test against endogenous regime changes, and the quality of the extracted latent factor all deteriorate quite considerably. In addition, our application to the S&P 500 index return data reveals strong evidence in favour of our non-Gaussian assumption, and the superiority of our model specification delivers an important risk management implication.
更多查看译文
关键词
endogeneity, fat tails, latent factor, non-Gaussian process, regime switching
AI 理解论文
溯源树
样例
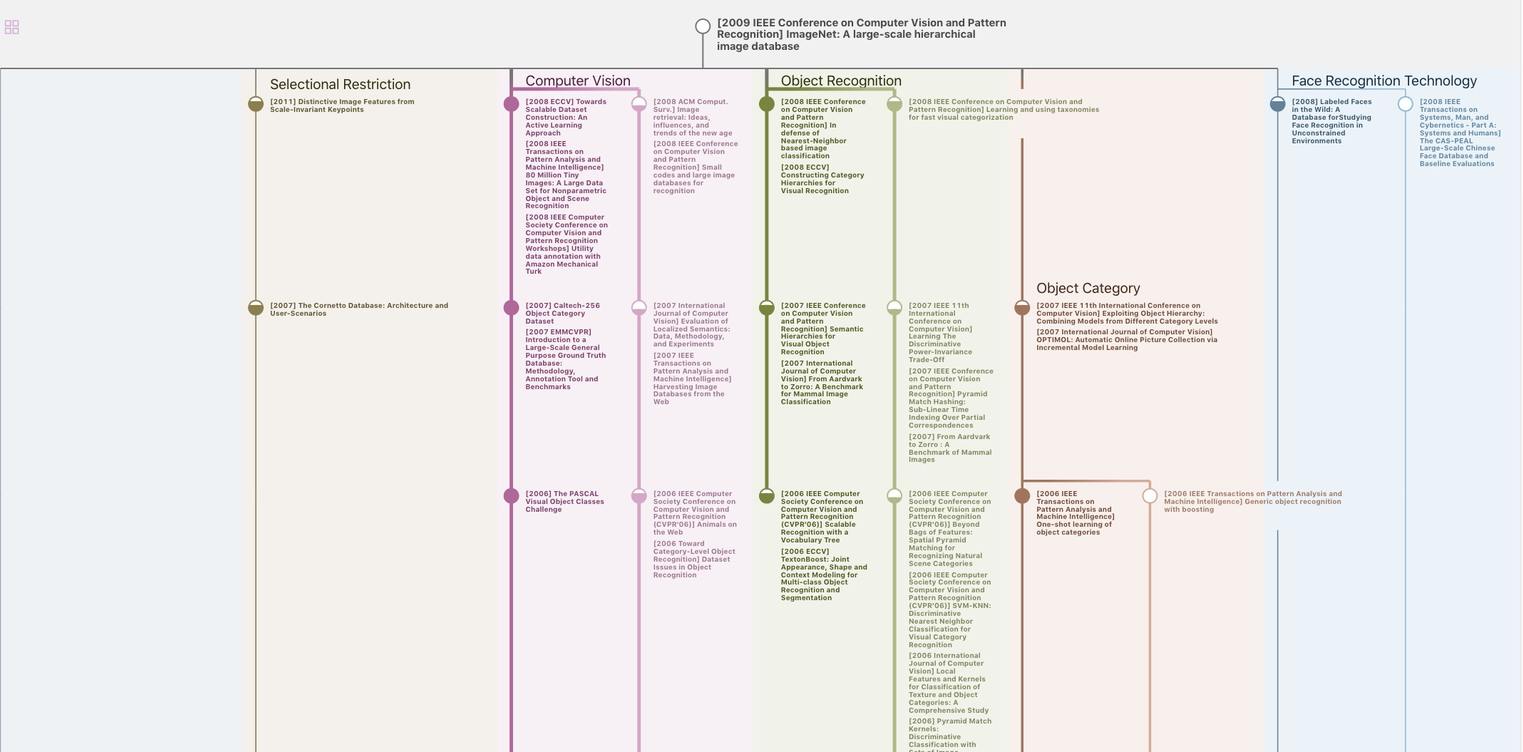
生成溯源树,研究论文发展脉络
Chat Paper
正在生成论文摘要