A gaussian process based algorithm for stochastic simulation optimization with input distribution uncertainty
Winter Simulation Conference(2020)
摘要
ABSTRACTStochastic simulation models are increasingly popular for analyzing complex stochastic systems. However, the input distributions driving the simulation models are typically unknown in practice and are usually estimated from real world data. Since the size of real world data tends to be limited, the resulting estimation of input distribution will contain errors. This estimation error is commonly known as input uncertainty. In this paper, we consider the stochastic simulation optimization problem when the input uncertainty is present and assume that both the family and parameters of the input distribution are unknown. Traditional efficient metamodel-based optimization approaches like Efficient Global Optimization (EGO) do not take the input uncertainty into account. This can lead to sub-optimal decisions when the input uncertainty level is high. Here, we adopt a nonparametric Bayesian approach to model the input uncertainty and propose an EGO-based simulation optimization algorithm that explicitly accounts for the input uncertainty.
更多查看译文
关键词
complex stochastic systems,stochastic simulation optimization problem,metamodel-based optimization,input uncertainty level,EGO-based simulation optimization algorithm,input distribution uncertainty,stochastic simulation models,Efficient Global Optimization,nonparametric Bayesian approach
AI 理解论文
溯源树
样例
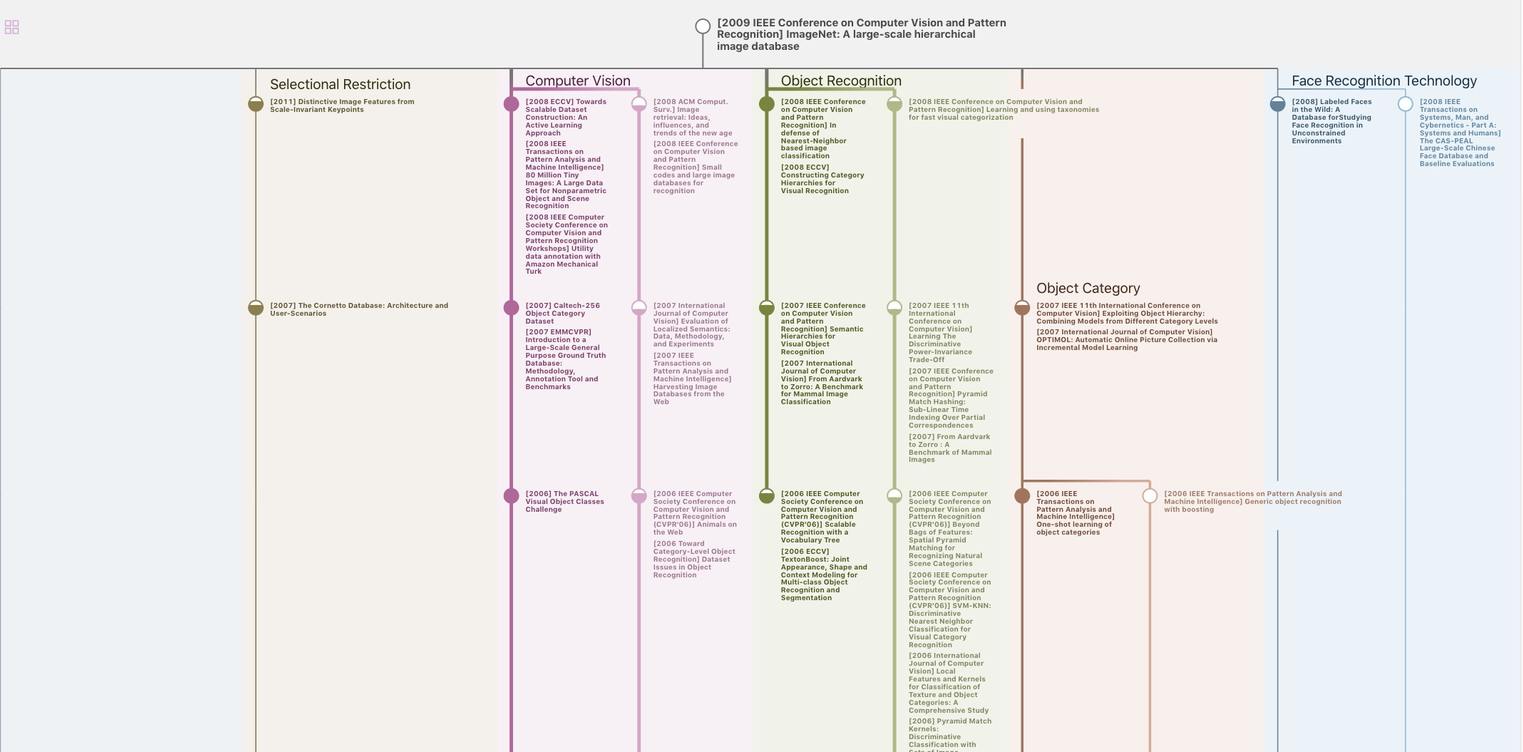
生成溯源树,研究论文发展脉络
Chat Paper
正在生成论文摘要