Interpretable anomaly detection for knowledge discovery in semiconductor manufacturing
Winter Simulation Conference(2020)
摘要
ABSTRACTMachine Learning-based Anomaly Detection approaches are efficient tools to monitor complex processes. One of the advantages of such approaches is that they provide a unique anomaly indicator, a quantitative index that captures the degree of 'outlierness' of the process at hand considering possibly hundreds or more variables at the same time, the typical scenario in semiconductor manufacturing. One of the drawbacks of such approaches is that Root Cause Analysis is not guided by the system itself. In this work, we show the effectiveness of a method, called DIFFI, to equip Isolation Forest, one of the most popular Anomaly Detection algorithms, with interpretability traits that can help corrective actions and knowledge understanding. Such approach is validated on real world semiconductor manufacturing data related to a Chemical Vapor Deposition process.
更多查看译文
关键词
anomaly detection,knowledge discovery,machine learning,anomaly indicator,quantitative index,root cause analysis,interpretability traits,knowledge understanding,semiconductor manufacturing,chemical vapor deposition,outlierness,DIFFI,isolation forest,corrective actions,unsupervised learning
AI 理解论文
溯源树
样例
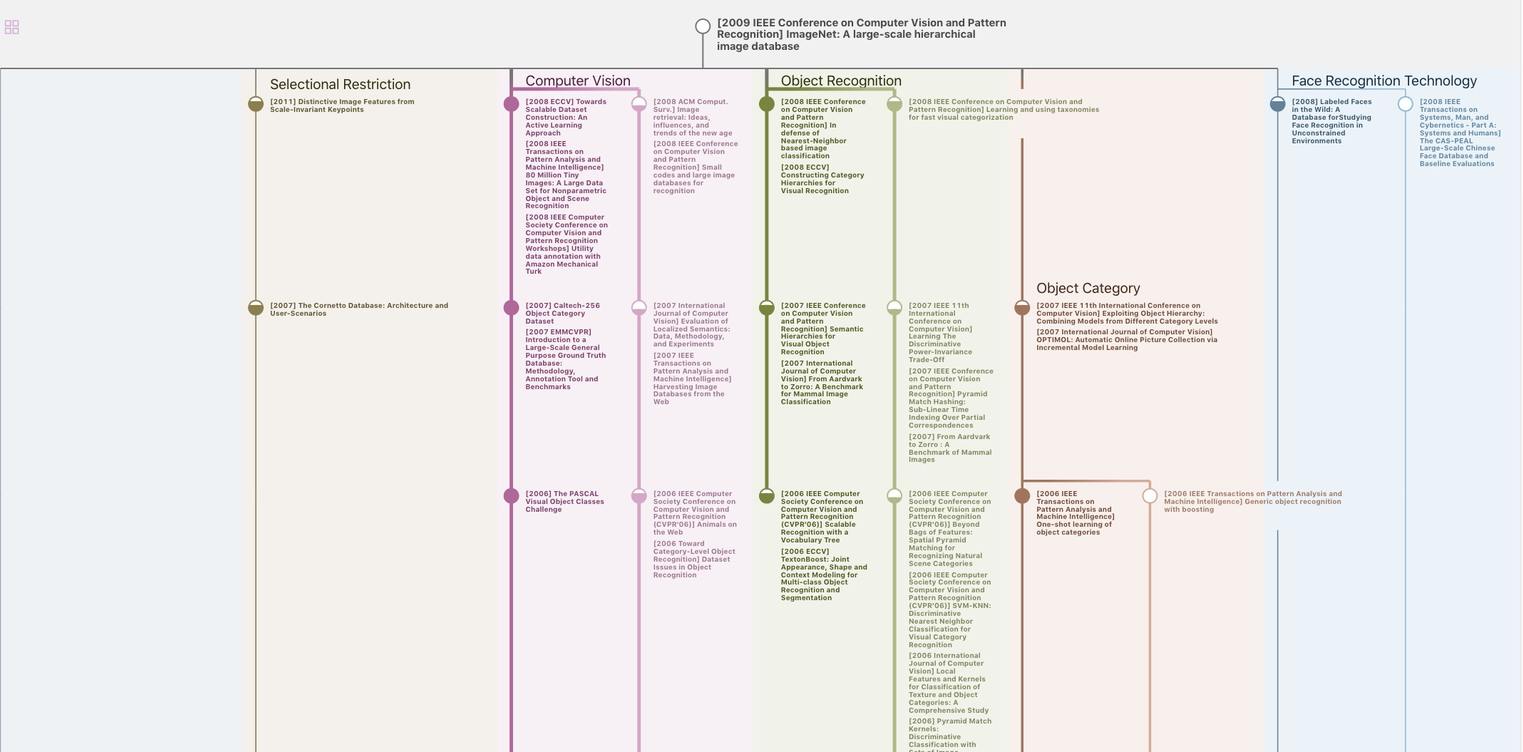
生成溯源树,研究论文发展脉络
Chat Paper
正在生成论文摘要