Centrality And Scalability Analysis On Distributed Graph Of Large-Scale E-Mail Dataset For Digital Forensics
2020 IEEE INTERNATIONAL CONFERENCE ON BIG DATA (BIG DATA)(2020)
摘要
Today's digital forensics software tools mostly do not offer automatic analysis methods to reveal evidences among huge amounts of digital files within hard disk images. It is important that finding evidence in digital and cyber forensics investigations as soon as possible by examining hard disk images. E-mails constitute a rich source of information in hard disk images, and they are the most possible data source to obtain an evidence. The analyzers search e-mail files by manually or using traditional methods in order to find an evidence. However, this operation could take a long time due to the size of the e-mail data which can contain a huge number of files and a huge volume of data. This study introduces an end-to-end distributed graph analysis framework for large-scale digital forensic datasets, and evaluates the accuracy of the centrality algorithms and the scalability of the proposed framework in terms of running time performance. The framework is comprised of specific processes to perform pre-processing, graph building, and algorithm activities. An architecture is introduced based on distributed big data techniques. Three different centrality algorithms are implemented to analyze the accuracy of our framework. Further, three implementations are provided to demonstrate the running time performance of our framework. Experiments are performed on Enron e-mail dataset to analyze the centrality algorithms, to evaluate the performance of the framework, and to compare the running times between the traditional approach and our approach. Moreover, the running time performance of the framework is evaluated under various parallelization level. The accuracy of the results is also evaluated and compared between the centrality algorithms. The comparison shows that some certain algorithms provide more accurate results and it is possible to improve the running time by orders of magnitude utilizing our end-to-end distributed graph analysis approach.
更多查看译文
关键词
graph analysis, centrality measurement, runtime comparison, scalability, distributed systems, big data, digital forensics, e-mail forensics
AI 理解论文
溯源树
样例
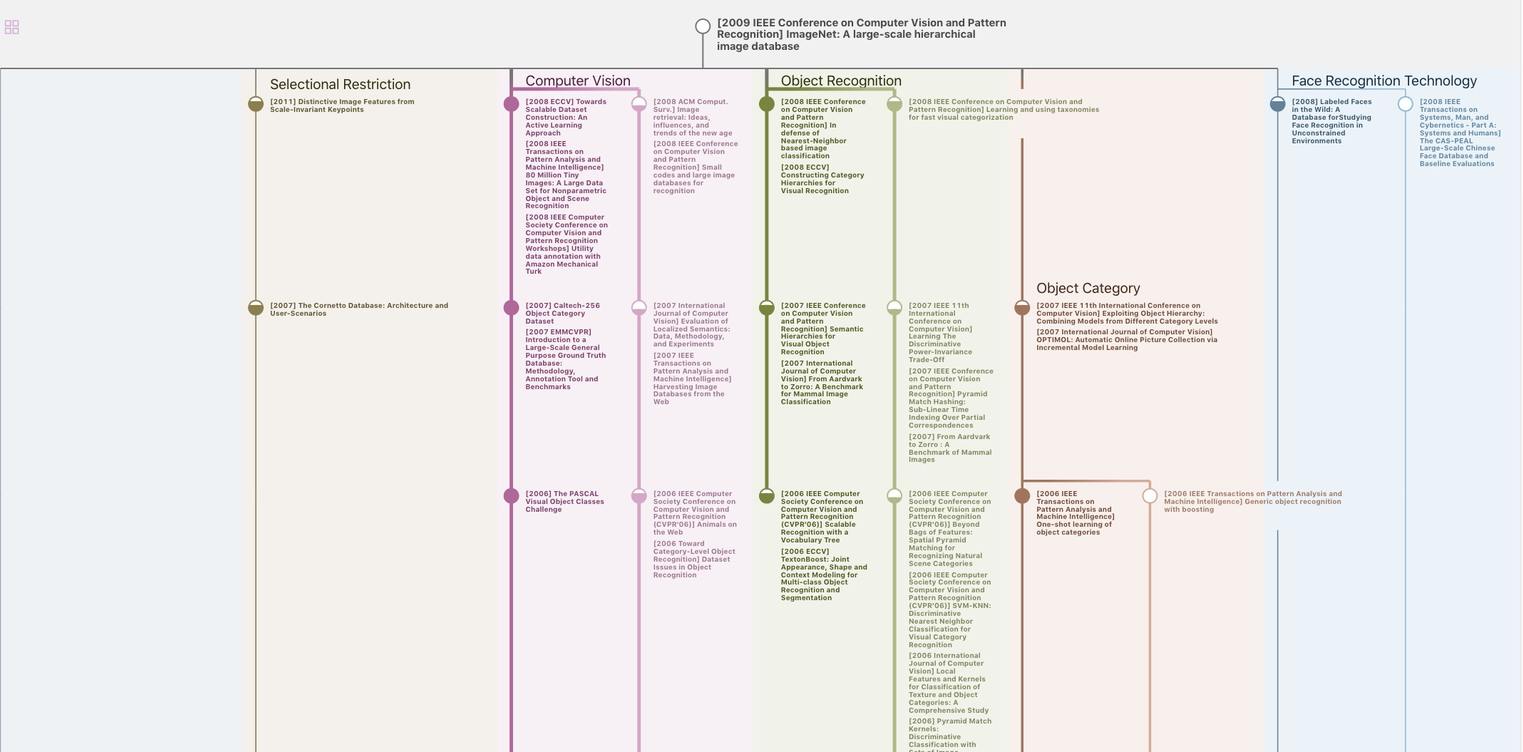
生成溯源树,研究论文发展脉络
Chat Paper
正在生成论文摘要