From Prediction to Prescription: Evolutionary Optimization of Nonpharmaceutical Interventions in the COVID-19 Pandemic
IEEE Transactions on Evolutionary Computation(2021)
摘要
Several models have been developed to predict how the COVID-19 pandemic spreads, and how it could be contained with nonpharmaceutical interventions, such as social distancing restrictions and school and business closures. This article demonstrates how evolutionary AI can be used to facilitate the next step, i.e., determining most effective intervention strategies automatically. Through evolutionary surrogate-assisted prescription, it is possible to generate a large number of candidate strategies and evaluate them with predictive models. In principle, strategies can be customized for different countries and locales, and balance the need to contain the pandemic and the need to minimize their economic impact. Early experiments suggest that workplace and school restrictions are the most important and need to be designed carefully. They also demonstrate that results of lifting restrictions can be unreliable, and suggest creative ways in which restrictions can be implemented softly, e.g., by alternating them over time. As more data becomes available, the approach can be increasingly useful in dealing with COVID-19 as well as possible future pandemics.
更多查看译文
关键词
Decision support systems,evolutionary computation,neural networks,neuroevolution,predictive models,prescriptive models,surrogate modeling,uncertainty estimation
AI 理解论文
溯源树
样例
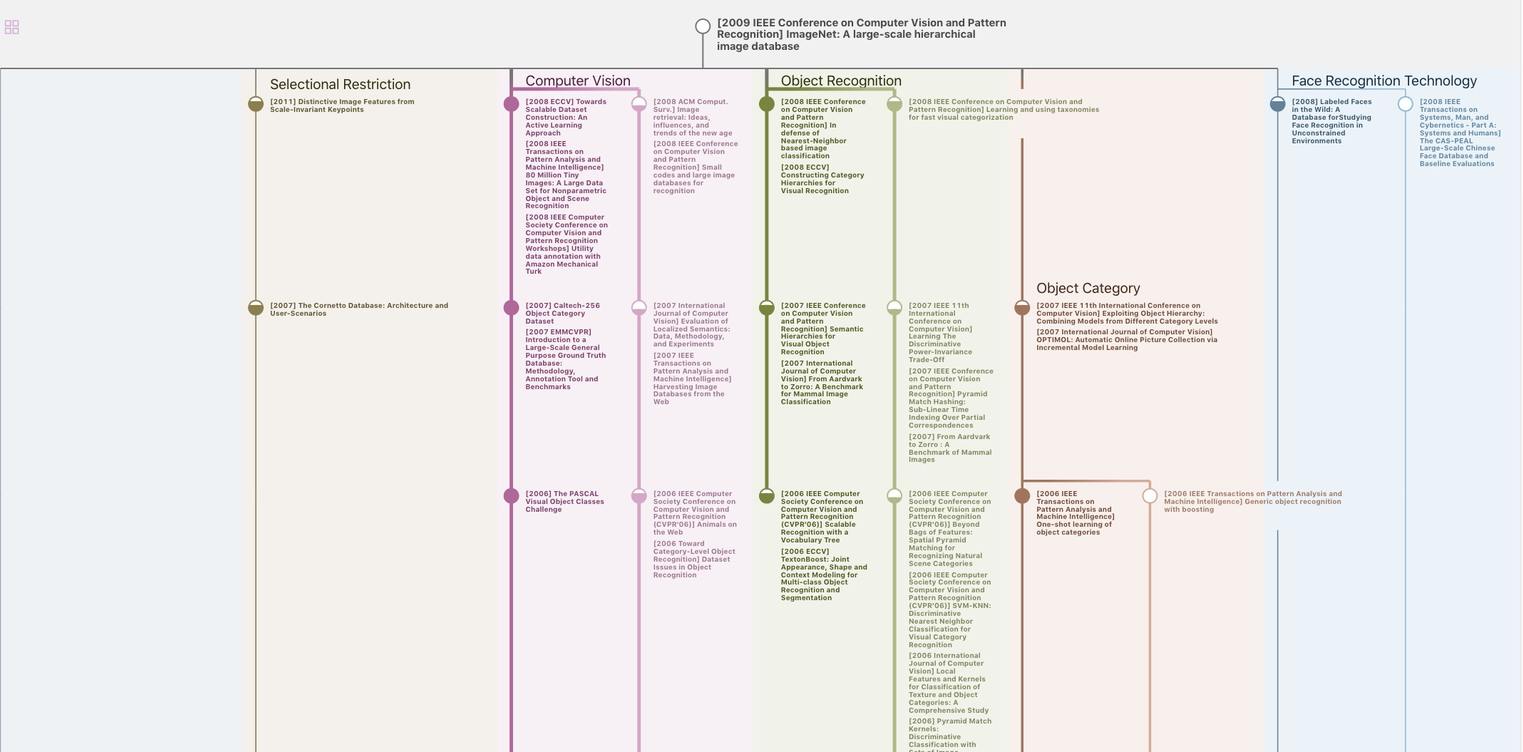
生成溯源树,研究论文发展脉络
Chat Paper
正在生成论文摘要