EXPATS: A Toolkit for Explainable Automated Text Scoring
arxiv(2021)
摘要
Automated text scoring (ATS) tasks, such as automated essay scoring and readability assessment, are important educational applications of natural language processing. Due to their interpretability of models and predictions, traditional machine learning (ML) algorithms based on handcrafted features are still in wide use for ATS tasks. Practitioners often need to experiment with a variety of models (including deep and traditional ML ones), features, and training objectives (regression and classification), although modern deep learning frameworks such as PyTorch require deep ML expertise to fully utilize. In this paper, we present EXPATS, an open-source framework to allow its users to develop and experiment with different ATS models quickly by offering flexible components, an easy-to-use configuration system, and the command-line interface. The toolkit also provides seamless integration with the Language Interpretability Tool (LIT) so that one can interpret and visualize models and their predictions. We also describe two case studies where we build ATS models quickly with minimal engineering efforts. The toolkit is available at \url{https://github.com/octanove/expats}.
更多查看译文
关键词
explainable automated text scoring,toolkit
AI 理解论文
溯源树
样例
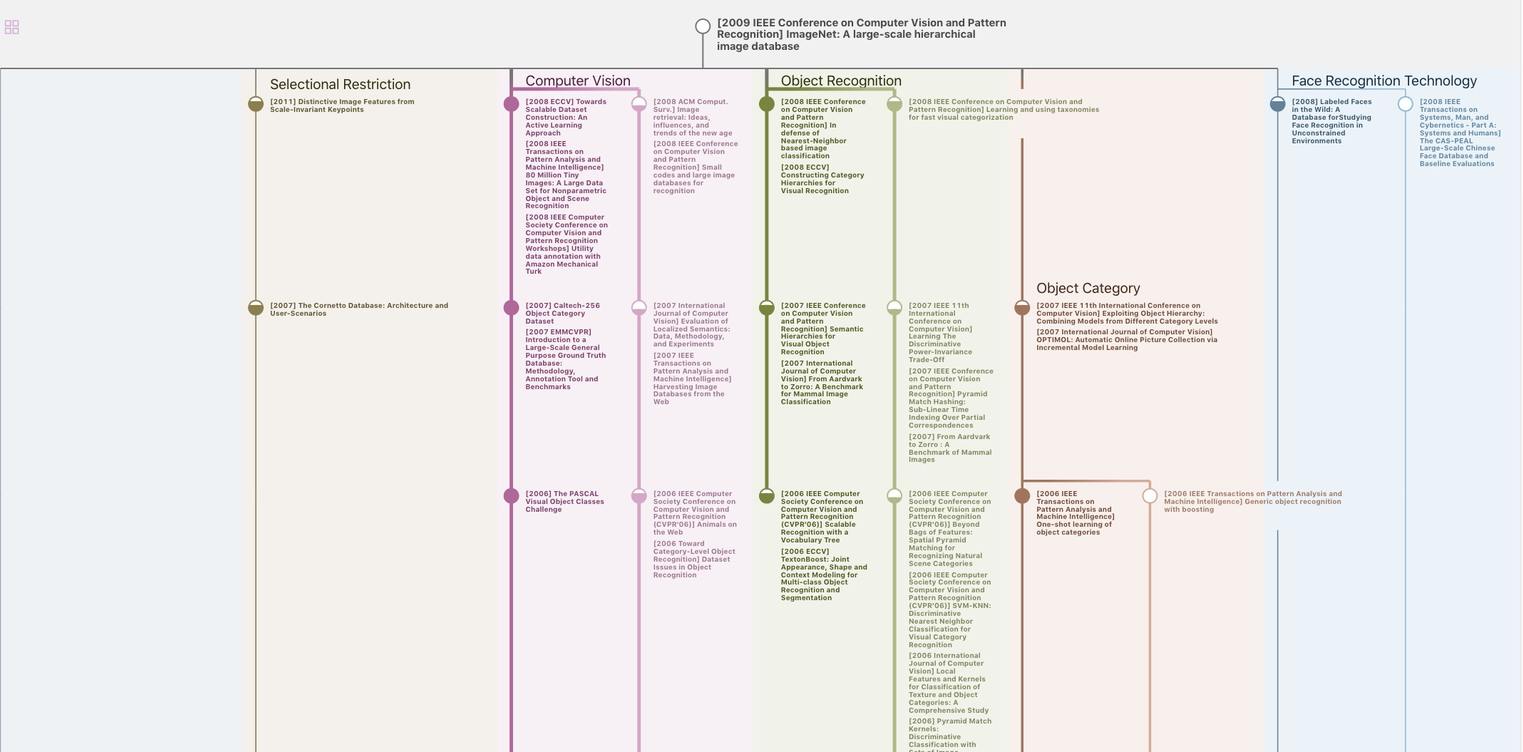
生成溯源树,研究论文发展脉络
Chat Paper
正在生成论文摘要