Few-Shot Meta-Learning on Point Cloud for Semantic Segmentation
arxiv(2021)
摘要
The promotion of construction robots can solve the problem of human resource shortage and improve the quality of decoration. Meanwhile, 3D point cloud is an important form of data for obtaining environmental information, which is widely used in robotics, autonomous driving and other fields. In order to work better, construction robots need to be able to understand their surroundings. However, as the robot renovates a house, the point cloud information changes dynamically. For the purpose of making the robot dynamically adapt to the changes of the environment, this paper proposes a semantic segmentation method for point cloud based on meta-learning. The method includes a basic learning module and a meta-learning module. The learning module is responsible for learning data features and evaluating the model, while the meta-learning module is responsible for updating the parameters of the model and improving the model generalization capability. In our work, we pioneered the method of producing datasets for meta-learning in 3D scenes, as well as demonstrated that the Model-Agnostic Meta-Learning (MAML) algorithm can be applied to process 3D point cloud data. At the same time, experiments show that our method can allow the model to be quickly applied to new environments with a small number of samples. Our proposed method has great application value.
更多查看译文
关键词
point cloud,semantic segmentation,few-shot,meta-learning
AI 理解论文
溯源树
样例
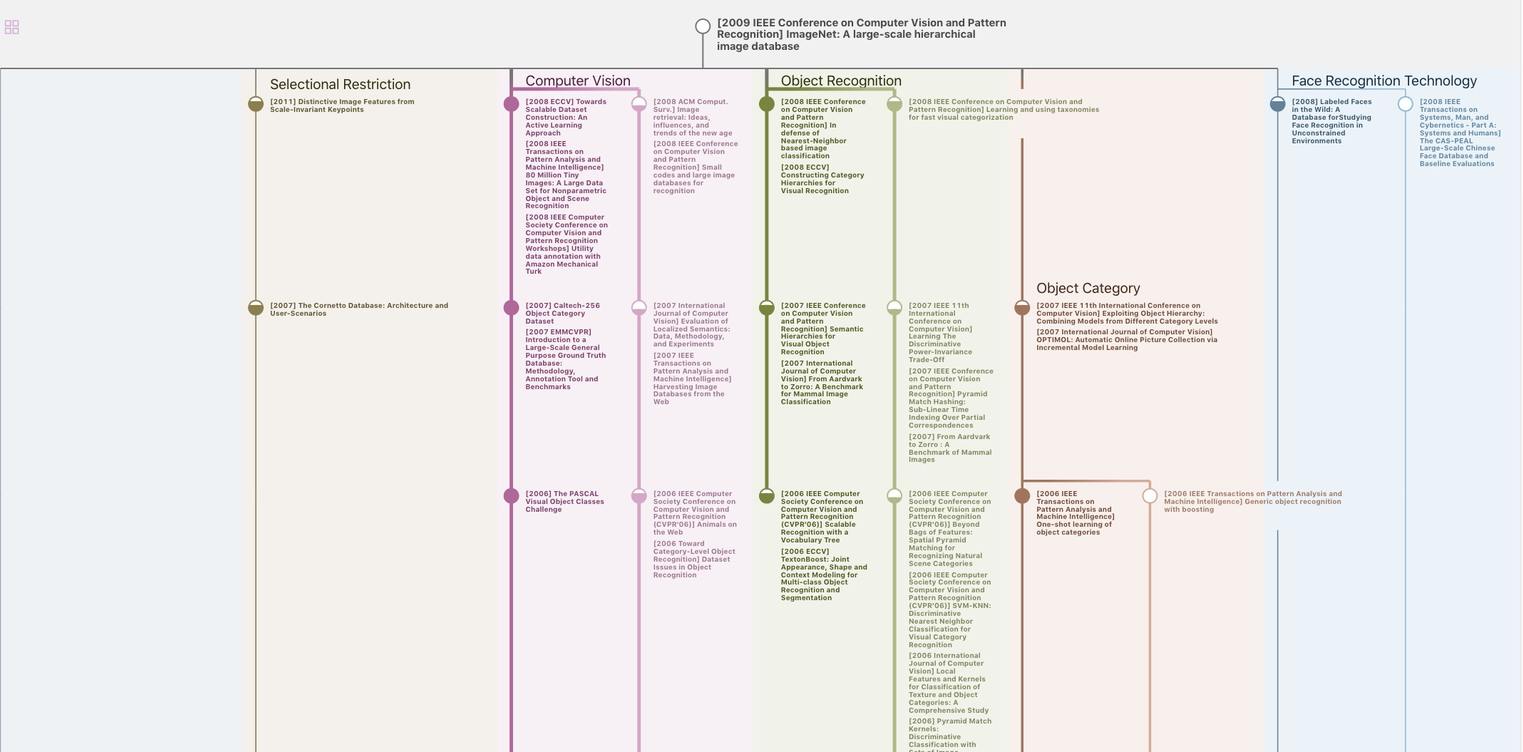
生成溯源树,研究论文发展脉络
Chat Paper
正在生成论文摘要