Unifying Theory Of Quantum State Estimation Using Past And Future Information
PHYSICS REPORTS-REVIEW SECTION OF PHYSICS LETTERS(2021)
摘要
Quantum state estimation for continuously monitored dynamical systems involves assigning a quantum state to an individual system at some time, conditioned on the results of continuous observations. The quality of the estimation depends on how much observed information is used and on how optimality is defined for the estimate. In this work, we consider problems of quantum state estimation where some of the measurement records are not available, but where the available records come from both before (past) and after (future) the estimation time, enabling better estimates than is possible using the past information alone. Past-future information for quantum systems has been used in various ways in the literature, in particular, the quantum state smoothing, the most-likely path, and the two-state vector and related formalisms. To unify these seemingly unrelated approaches, we propose a framework for partially observed quantum systems with continuous monitoring, wherein the first two existing formalisms can be accommodated, with some generalization. The unifying framework is based on state estimation with expected cost minimization, where the cost can be defined either in the space of the unknown record or in the space of the unknown true state. Moreover, we connect all three existing approaches conceptually by defining five new cost functions, and thus new types of estimators, which bridge the gaps between them. We illustrate the applicability of our method by calculating all seven estimators we consider for the example of a driven two-level system dissipatively coupled to bosonic baths. Our theory also allows connections to classical state estimation, which create further conceptual links between our quantum state estimators. (C) 2021 Elsevier B.V. All rights reserved.
更多查看译文
关键词
Quantum state estimation, Filtering, Smoothing, Continuous quantum measurement, Quantum trajectory, Cost functions, Stochastic processes
AI 理解论文
溯源树
样例
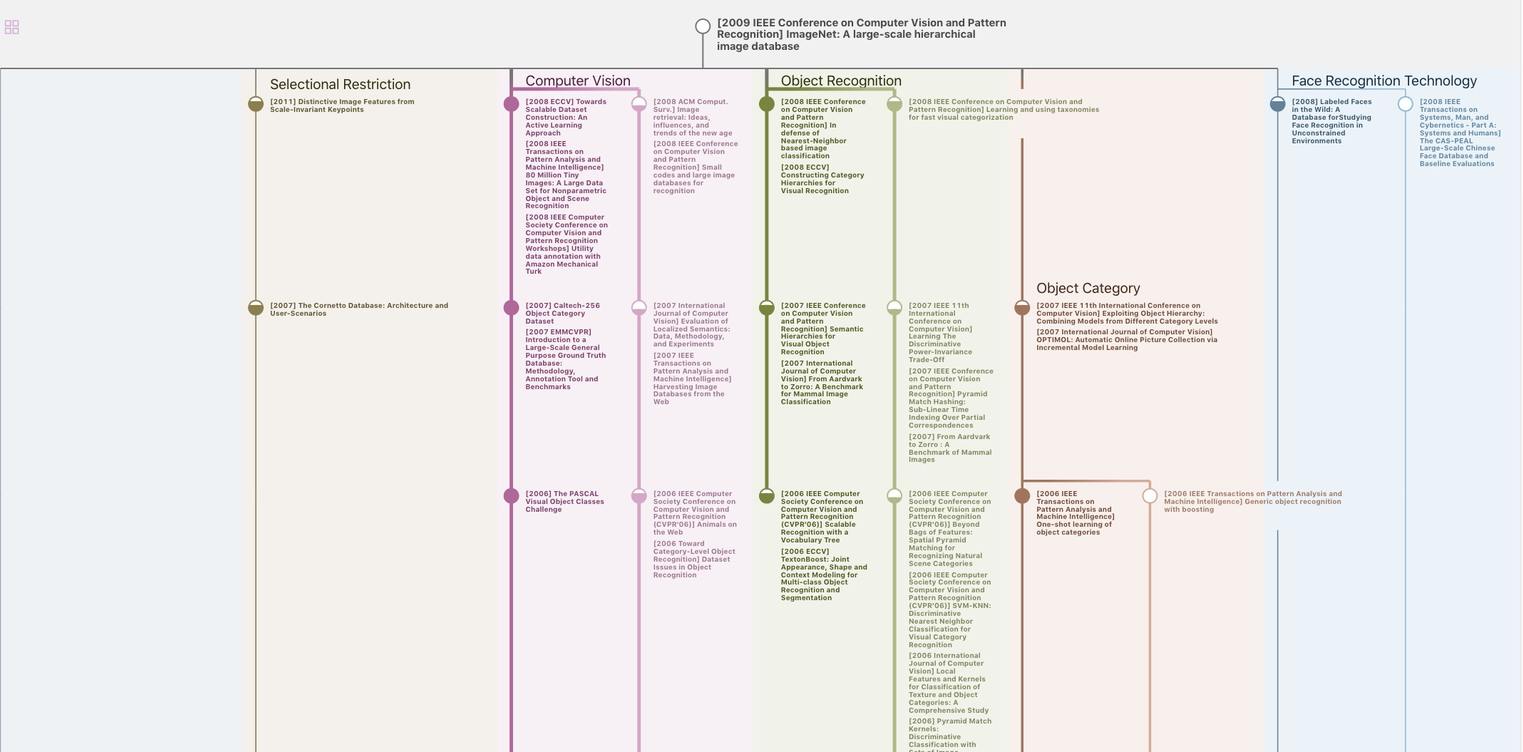
生成溯源树,研究论文发展脉络
Chat Paper
正在生成论文摘要