Comparing CTC and LFMMI for Out-of-Domain Adaptation of wav2vec 2.0 Acoustic Model.
Interspeech(2021)
摘要
In this work, we investigate if the wav2vec 2.0 self-supervised pretraining helps mitigate the overfitting issues with connectionist temporal classification (CTC) training to reduce its performance gap with flat-start lattice-free MMI (E2E-LFMMI) for automatic speech recognition with limited training data. Towards that objective, we use the pretrained wav2vec 2.0 BASE model and fine-tune it on three different datasets including out-of-domain (Switchboard) and cross-lingual (Babel) scenarios. Our results show that for supervised adaptation of the wav2vec 2.0 model, both E2E-LFMMI and CTC achieve similar results; significantly outperforming the baselines trained only with supervised data. Fine-tuning the wav2vec 2.0 model with E2E-LFMMI and CTC we obtain the following relative WER improvements over the supervised baseline trained with E2E-LFMMI. We get relative improvements of 40% and 44% on the clean-set and 64% and 58% on the test set of Librispeech (100h) respectively. On Switchboard (300h) we obtain relative improvements of 33% and 35% respectively. Finally, for Babel languages, we obtain relative improvements of 26% and 23% on Swahili (38h) and 18% and 17% on Tagalog (84h) respectively.
更多查看译文
关键词
speech recognition,wav2vec 2.0,e2e-lfmmi,ctc,cross-lingual adaptation
AI 理解论文
溯源树
样例
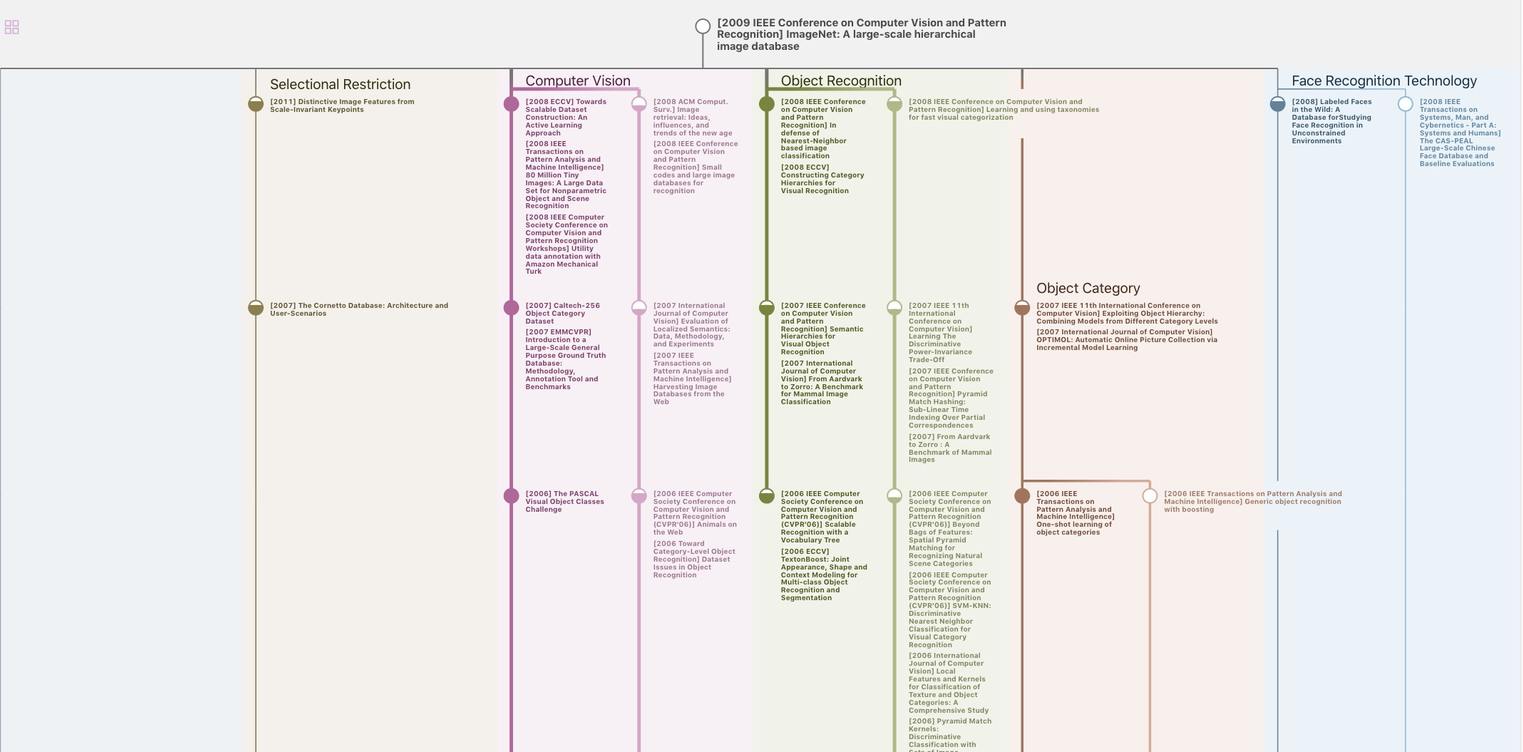
生成溯源树,研究论文发展脉络
Chat Paper
正在生成论文摘要