Correcting Susceptibility Artifacts Of Mri Sensors In Brain Scanning: A 3d Anatomy-Guided Deep Learning Approach
SENSORS(2021)
摘要
Echo planar imaging (EPI), a fast magnetic resonance imaging technique, is a powerful tool in functional neuroimaging studies. However, susceptibility artifacts, which cause misinterpretations of brain functions, are unavoidable distortions in EPI. This paper proposes an end-to-end deep learning framework, named TS-Net, for susceptibility artifact correction (SAC) in a pair of 3D EPI images with reversed phase-encoding directions. The proposed TS-Net comprises a deep convolutional network to predict a displacement field in three dimensions to overcome the limitation of existing methods, which only estimate the displacement field along the dominant-distortion direction. In the training phase, anatomical T1-weighted images are leveraged to regularize the correction, but they are not required during the inference phase to make TS-Net more flexible for general use. The experimental results show that TS-Net achieves favorable accuracy and speed trade-off when compared with the state-of-the-art SAC methods, i.e., TOPUP, TISAC, and S-Net. The fast inference speed (less than a second) of TS-Net makes real-time SAC during EPI image acquisition feasible and accelerates the medical image-processing pipelines.
更多查看译文
关键词
susceptibility artifacts, deep learning, high-speed, echo planar imaging, reversed phase-encoding
AI 理解论文
溯源树
样例
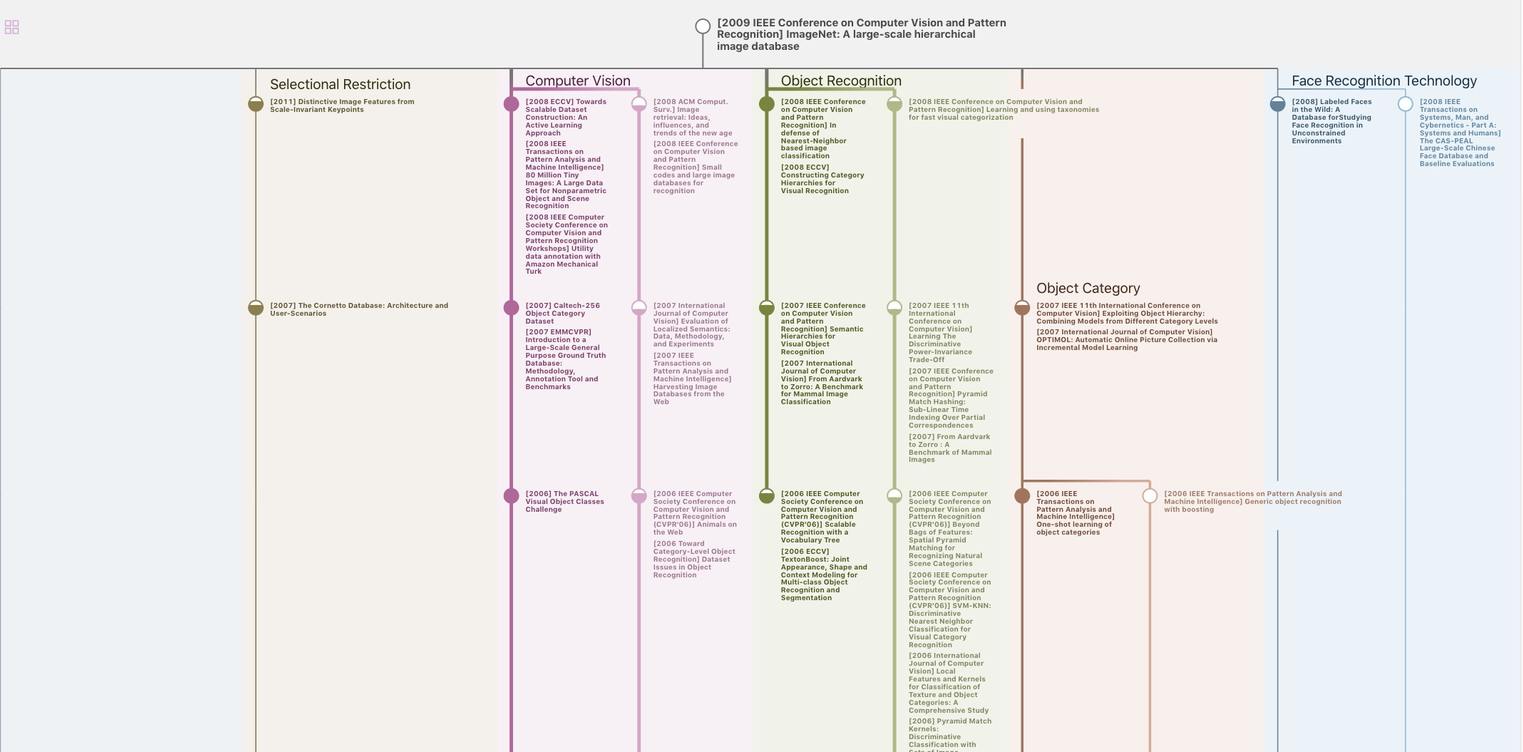
生成溯源树,研究论文发展脉络
Chat Paper
正在生成论文摘要