Prediction Of Neurologically Intact Survival In Cardiac Arrest Patients Without Pre-Hospital Return Of Spontaneous Circulation: Machine Learning Approach
JOURNAL OF CLINICAL MEDICINE(2021)
摘要
Current multimodal approaches for the prognostication of out-of-hospital cardiac arrest (OHCA) are based mainly on the prediction of poor neurological outcomes; however, it is challenging to identify patients expected to have a favorable outcome, especially before the return of spontaneous circulation (ROSC). We developed and validated a machine learning-based system to predict good outcome in OHCA patients before ROSC. This prospective, multicenter, registry-based study analyzed non-traumatic OHCA data collected between October 2015 and June 2017. We used information available before ROSC as predictor variables, and the primary outcome was neurologically intact survival at discharge, defined as cerebral performance category 1 or 2. The developed models' robustness were evaluated and compared with various score metrics to confirm their performance. The model using a voting classifier had the best performance in predicting good neurological outcome (area under the curve = 0.926). We confirmed that the six top-weighted variables predicting neurological outcomes, such as several duration variables after the instant of OHCA and several electrocardiogram variables in the voting classifier model, showed significant differences between the two neurological outcome groups. These findings demonstrate the potential utility of a machine learning model to predict good neurological outcome of OHCA patients before ROSC.
更多查看译文
关键词
emergency departments, machine learning, out-of-hospital cardiac arrest, outcomes, resuscitation, targeted temperature management
AI 理解论文
溯源树
样例
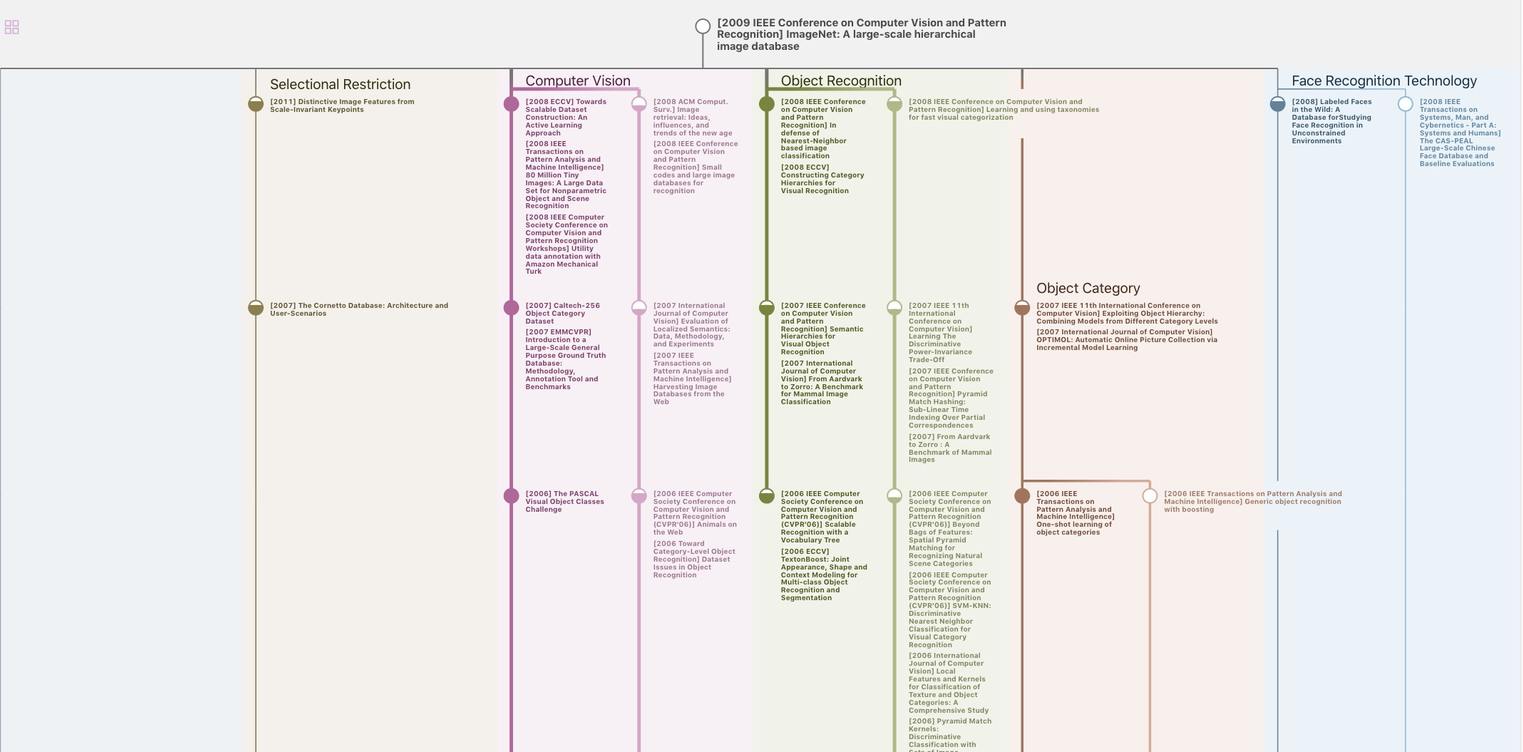
生成溯源树,研究论文发展脉络
Chat Paper
正在生成论文摘要